A deep learning model-based reconstruction of Landsat NDVI and its application for vegetation phenology monitoring
crossref(2024)
摘要
High quality Normalized Difference Vegetation Index (NDVI) time series data are important for regional and global ecological applications. However, missing observations in cloudy and shaded areas in current high-resolution satellite data severely limit their use. Traditional temporal filtering reconstruction methods are easily affected by frequent fluctuations, large data gaps and irregular noise in the observations. Meanwhile, satellite images often lack complete and accurate real data as training sets, which limits deep learning-based reconstruction methods. To address these problems, this paper proposes a reconstruction method with Landsat-8 NDVI time-series images based on bidirectional long-short memory networks (Bi-LSTM). The core of the method is a sequence-to-sequence time series reconstruction model using Bi-LSTM as an encoder, decoder, and additionally adding a mask layer to locate irregular gaps in the time series data. To train the model, a multi-scale continuous NDVI time series dataset was constructed using near-surface observations and satellite fused images. Simulation experiments were conducted in 48 areas covering PhenoCam sites in North America. The coefficient of determination (R2) between the simulation results of this method and the real observations reached 0.7024 with an absolute bias of -0.0080, which outperformed the filtering method both in predicting missing cloud-covered areas and in extracting key nodes for vegetation growth. This method is pre-trained on a large-scale ground sample dataset before fine-tuning the parameters for the scenarios, which can be easily and quickly applied to generate long-term continuous NDVI datasets at a large spatial scale. In addition, the analysis of the phenology monitoring in the experimental areas shows that the results extracted from the reconstructed results of this method is very close to the PhenoCam-measured values, which is significantly improved compared to the extraction results using original Landsat data only, and at the same time shows more spatial detail compared to the MODIS climate products. This indicates that the 30m gap-free NDVI time series is sufficient for analysis and understanding of climate dynamics in heterogeneous areas with multiple vegetation growth patterns, mixing plant components and microclimates variation, which are difficult to be identified by the coarse spatial resolution phenology product.
更多查看译文
AI 理解论文
溯源树
样例
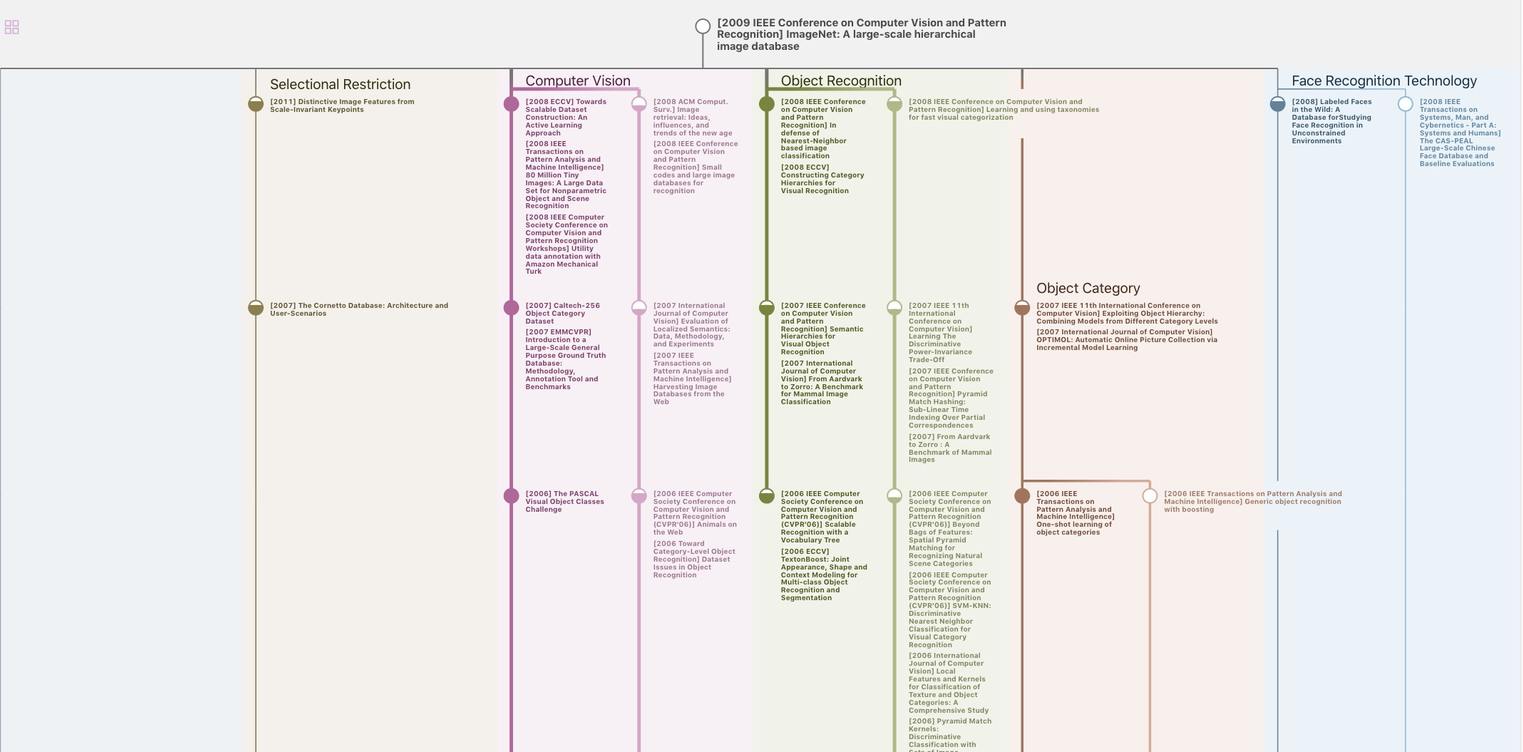
生成溯源树,研究论文发展脉络
Chat Paper
正在生成论文摘要