Policy Rehearsing: Training Generalizable Policies for Reinforcement Learning
ICLR 2024(2024)
摘要
Human beings can make adaptive decisions in a preparatory manner, i.e., by making preparations in advance, which offers significant advantages in scenarios where both online and offline experiences are expensive and limited. Meanwhile, current reinforcement learning methods commonly rely on numerous environment interactions but hardly obtain generalizable policies. In this paper, we introduce the idea of \textit{rehearsal} into policy optimization, where the agent plans for all possible outcomes in mind and acts adaptively according to actual responses from the environment. To effectively rehearse, we propose ReDM, an algorithm that generates a diverse and eligible set of dynamics models and then rehearse the policy via adaptive training on the generated model set. Rehearsal enables the policy to make decision plans for various hypothetical dynamics and to naturally generalize to previously unseen environments. Our experimental results demonstrate that ReDM is capable of learning a valid policy solely through rehearsal, even with \emph{zero} interaction data. We further extend ReDM to scenarios where limited or mismatched interaction data is available, and our experimental results reveal that ReDM produces high-performing policies compared to other offline RL baselines.
更多查看译文
关键词
Reinforcement Learning,Model-based Reinforcement Learning,Offline Reinforcement Learning
AI 理解论文
溯源树
样例
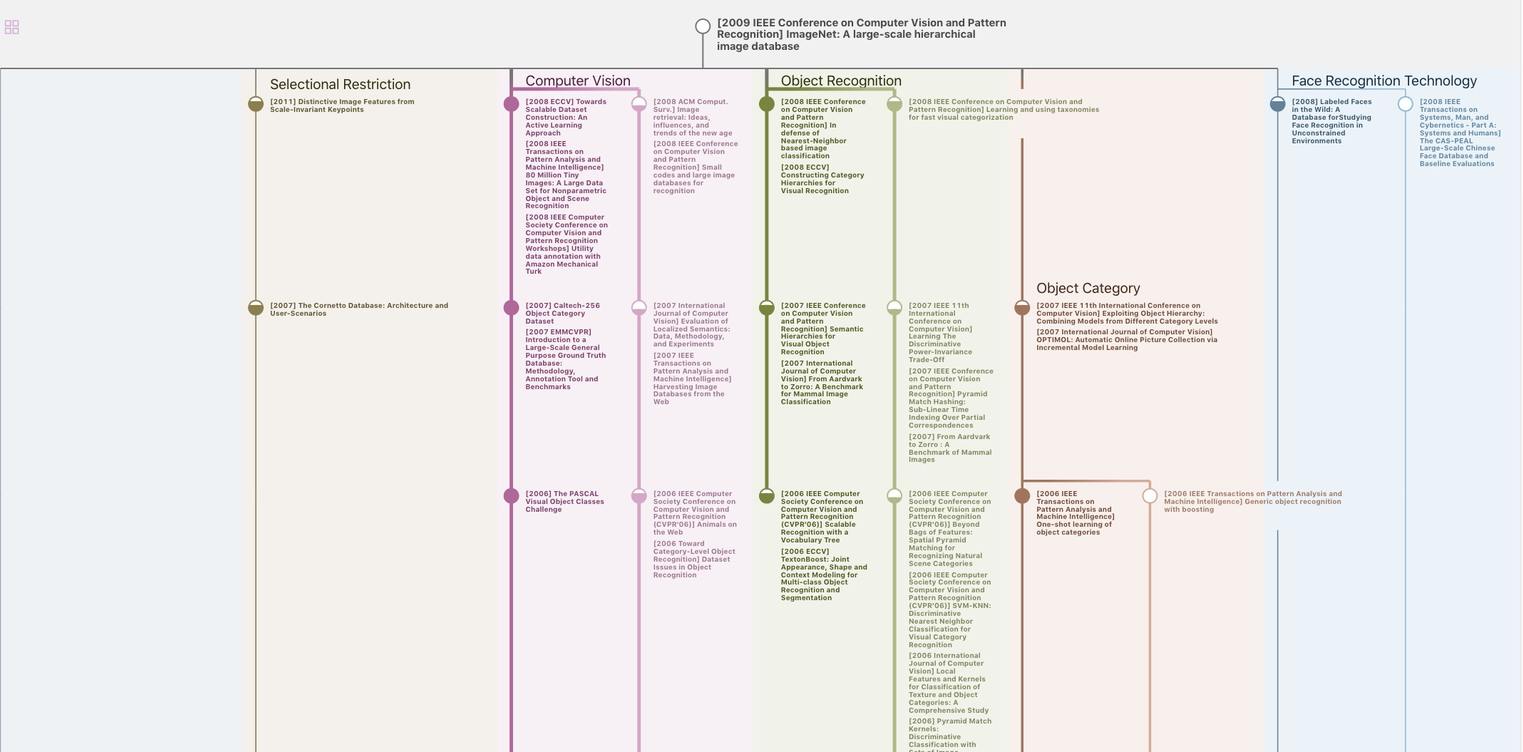
生成溯源树,研究论文发展脉络
Chat Paper
正在生成论文摘要