Exposing Text-Image Inconsistency Using Diffusion Models
ICLR 2024(2024)
摘要
In the battle against widespread online misinformation, a growing problem is text-image inconsistency, where images are misleadingly paired with texts with different intent or meaning. Existing classification-based methods for text-image inconsistency can identify contextual inconsistencies but fail to provide explainable justifications for their decisions that humans can understand. Although more nuanced, human evaluation is impractical at scale and susceptible to errors. To address these limitations, this study introduces D-TIIL (Diffusion-based Text-Image Inconsistency Localization), which employs text-to-image diffusion models to localize semantic inconsistencies in text and image pairs. These models, trained on large-scale datasets act as ``omniscient" agents that filter out irrelevant information and incorporate background knowledge to identify inconsistencies. In addition, D-TIIL uses text embeddings and modified image regions to visualize these inconsistencies. To evaluate D-TIIL's efficacy, we introduce a new TIIL dataset containing 14K consistent and inconsistent text-image pairs. Unlike existing datasets, TIIL enables assessment at the level of individual words and image regions and is carefully designed to represent various inconsistencies. D-TIIL offers a scalable and evidence-based approach to identifying and localizing text-image inconsistency, providing a robust framework for future research combating misinformation.
更多查看译文
关键词
inconsistency Detection,multi-modal learning,diffusion models
AI 理解论文
溯源树
样例
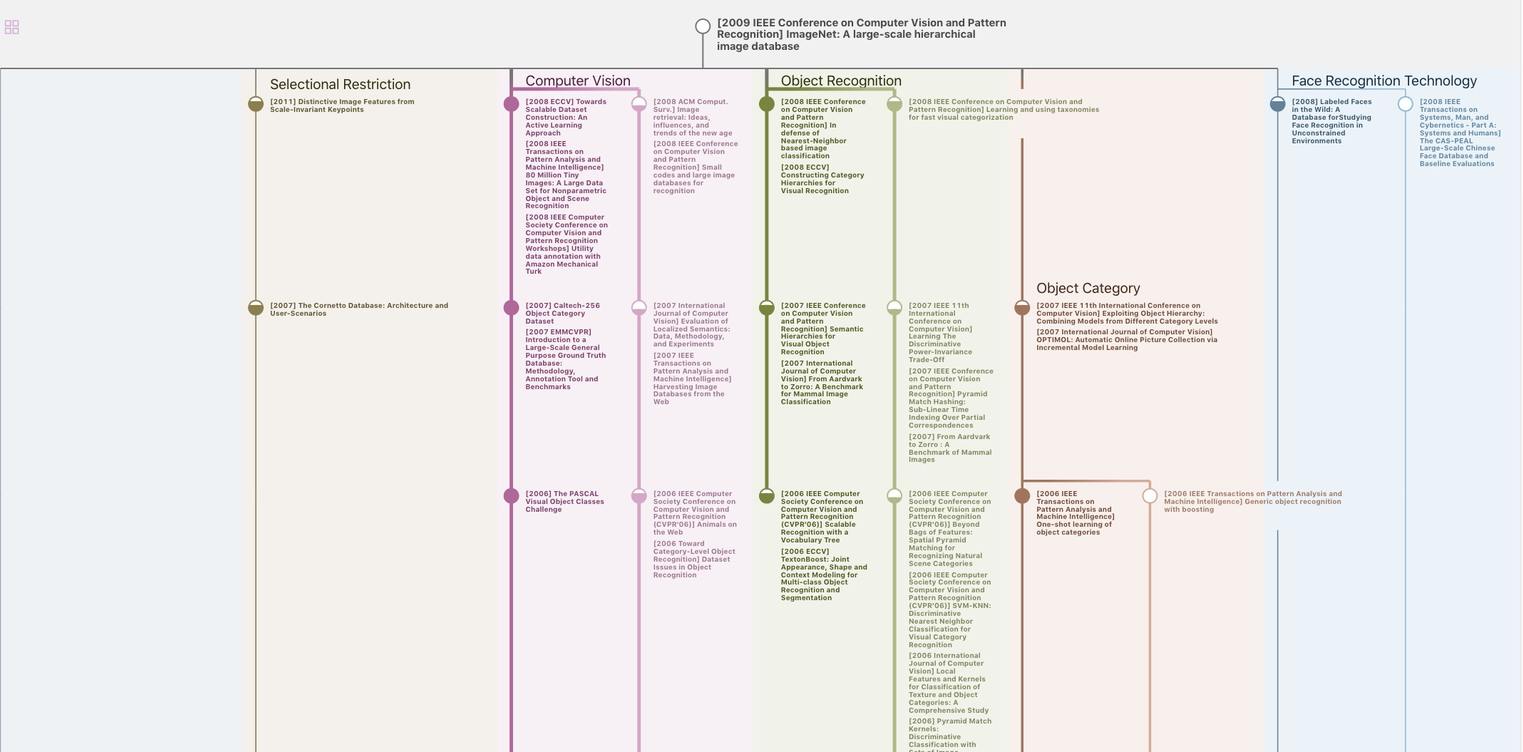
生成溯源树,研究论文发展脉络
Chat Paper
正在生成论文摘要