Threaten Spiking Neural Networks through Combining Rate and Temporal Information
ICLR 2024(2024)
摘要
Spiking Neural Networks (SNNs) have received widespread attention in academic communities due to their superior spatio-temporal processing capabilities and energy-efficient characteristics. With further in-depth application in various fields, the vulnerability of SNNs under adversarial attack has become a focus of concern.
In this paper, we draw inspiration from two mainstream learning algorithms of SNNs and observe that SNN models reserve both rate and temporal information. To better understand the capabilities of these two types of information, we conduct a quantitative analysis separately for each. In addition, we note that the retention degree of temporal information is related to the parameters and input settings of spiking neurons. Building on these insights, we propose a hybrid adversarial attack based on rate and temporal information (HART), which allows for dynamic adjustment of the rate and temporal attributes. Experimental results demonstrate that compared to previous works, HART attack can achieve significant superiority under different attack scenarios, data types, network architecture, time-steps, and model hyper-parameters. These findings call for further exploration into how both types of information can be effectively utilized to enhance the reliability of SNNs. Code is available at [https://github.com/hzc1208/HART_Attack](https://github.com/hzc1208/HART_Attack).
更多查看译文
关键词
Spiking Neural Networks,Rate and Temporal Information,Hybrid Adversarial Attack
AI 理解论文
溯源树
样例
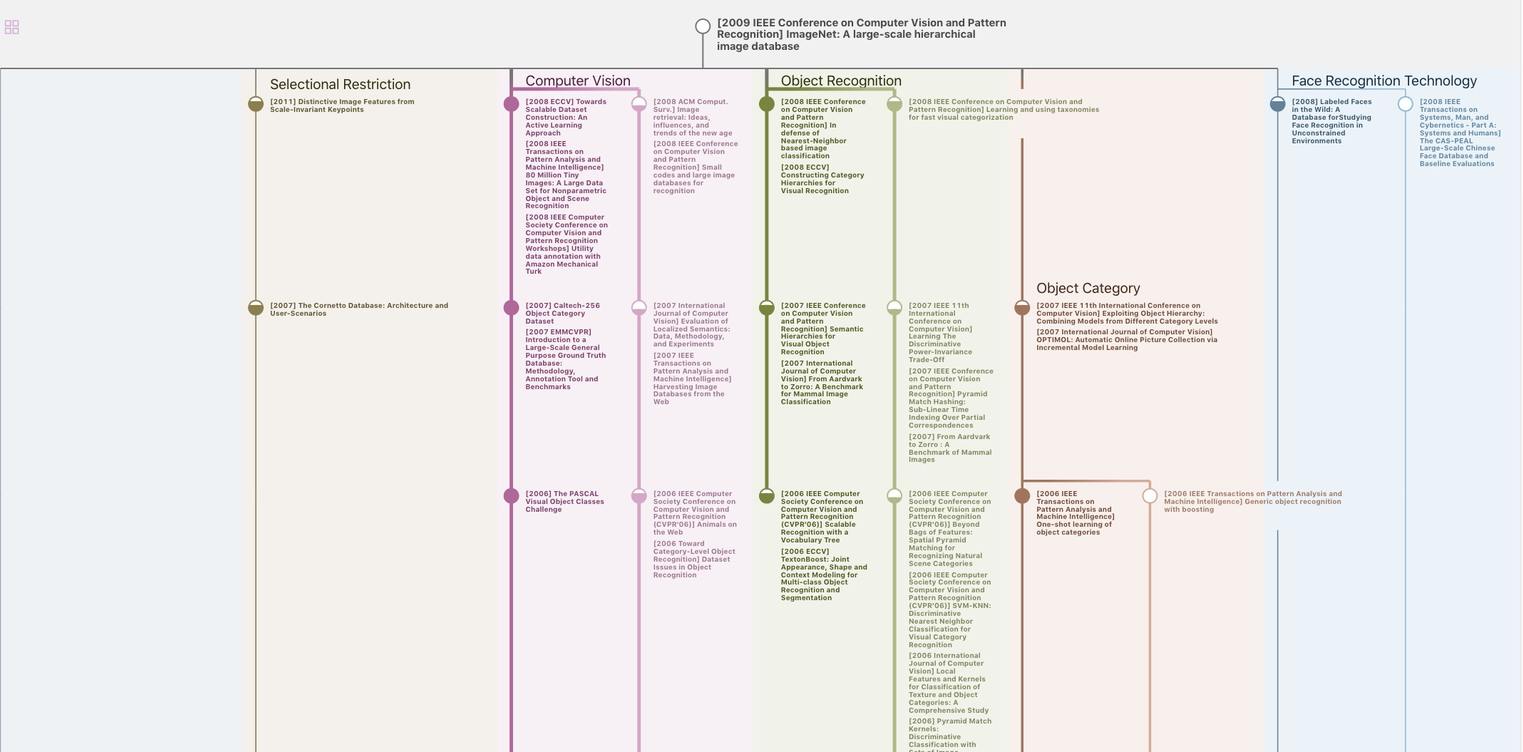
生成溯源树,研究论文发展脉络
Chat Paper
正在生成论文摘要