CPPO: Continual Learning for Reinforcement Learning with Human Feedback
ICLR 2024(2024)
摘要
The approach of Reinforcement Learning from Human Feedback (RLHF) is widely used for enhancing pre-trained Language Models (LM), enabling them to better align with human preferences. Existing RLHF-based LMs however require complete retraining whenever new queries or feedback are introduced, as human preferences may differ across different domains or topics. LM retraining is often impracticable in most real-world scenarios, due to the substantial time and computational costs involved, as well as data privacy concerns. To address this limitation, we propose Continual Proximal Policy Optimization (CPPO), a novel method that is able to continually align LM with dynamic human preferences. Specifically, CPPO adopts a weighting strategy to decide which samples should be utilized for enhancing policy learning and which should be used for solidifying past experiences. This seeks a good trade-off between policy learning and knowledge retention. Our experimental results show that CPPO outperforms strong Continuous learning (CL) baselines when it comes to consistently aligning with human preferences. Furthermore, compared to PPO, CPPO offers more efficient and stable learning in non-continual scenarios.
更多查看译文
关键词
lifelong learning,reinforcement learning,human feedback,proximal policy optimization
AI 理解论文
溯源树
样例
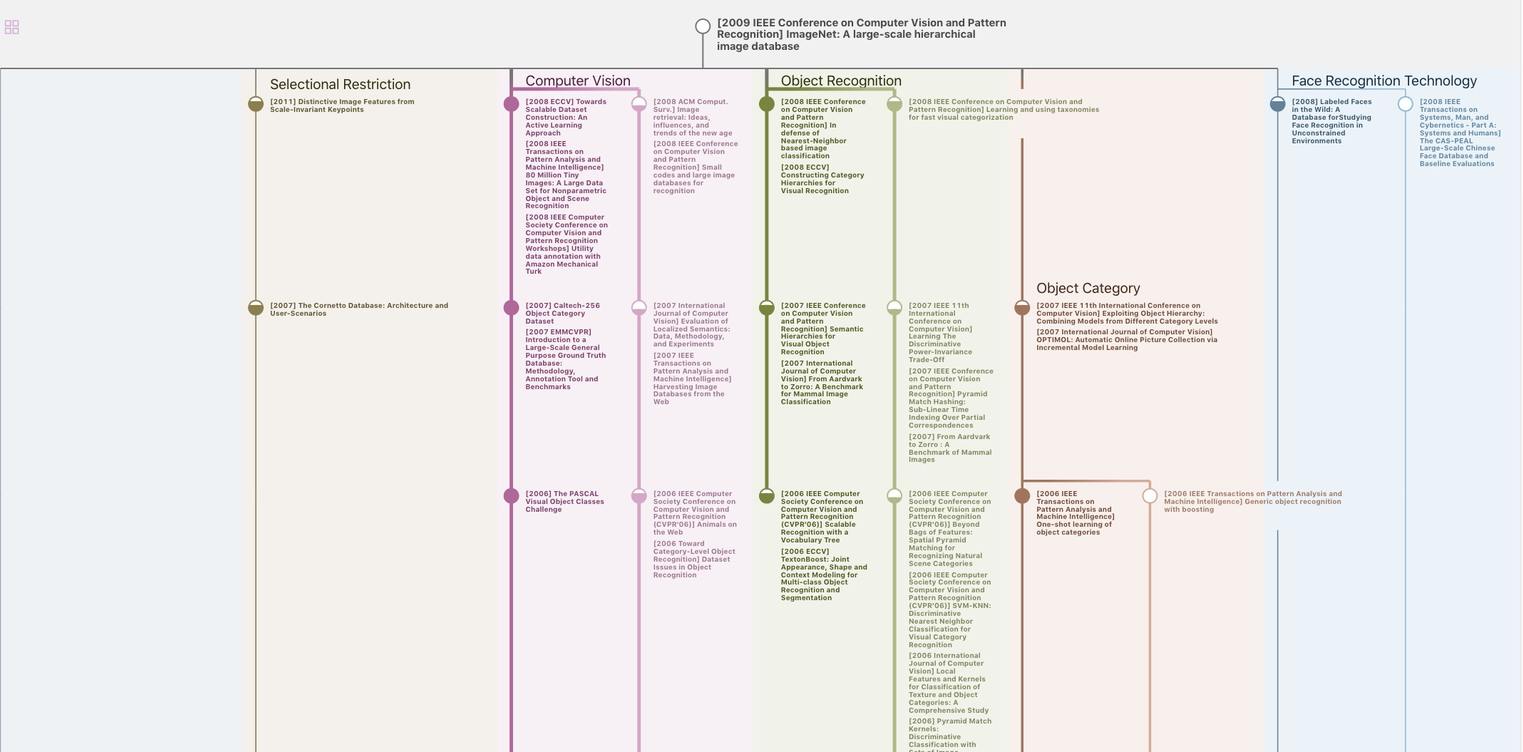
生成溯源树,研究论文发展脉络
Chat Paper
正在生成论文摘要