Kalman Filter Online Classification from non-Stationary Data
ICLR 2024(2024)
摘要
In Online Continual Learning (OCL) a learning system receives a stream of data and sequentially performs prediction and training steps. Important challenges in OCL are concerned with automatic adaptation to the particular non-stationary structure of the data, and with quantification of predictive uncertainty. Motivated by these challenges we introduce a probabilistic Bayesian online learning model by using a (possibly pretrained) neural representation and a state space model over the linear predictor weights. Non-stationarity over the linear predictor weights is modelled using a “parameter drift” transition density, parametrized by a coefficient that quantifies forgetting. Inference in the model is implemented with efficient Kalman filter recursions which track the posterior distribution over the linear weights, while online SGD updates over the transition dynamics coefficient allows to adapt to the non-stationarity seen in data. While the framework is developed assuming a linear Gaussian model, we also extend it to deal with classification problems and for fine-tuning the deep learning representation. In a set of experiments in multi-class classification using data sets such as CIFAR-100 and CLOC we demonstrate the predictive ability of the model and its flexibility to capture non-stationarity.
更多查看译文
关键词
online learning,non-stationarity,Kalman filter,continual learning,probabilistic modellling
AI 理解论文
溯源树
样例
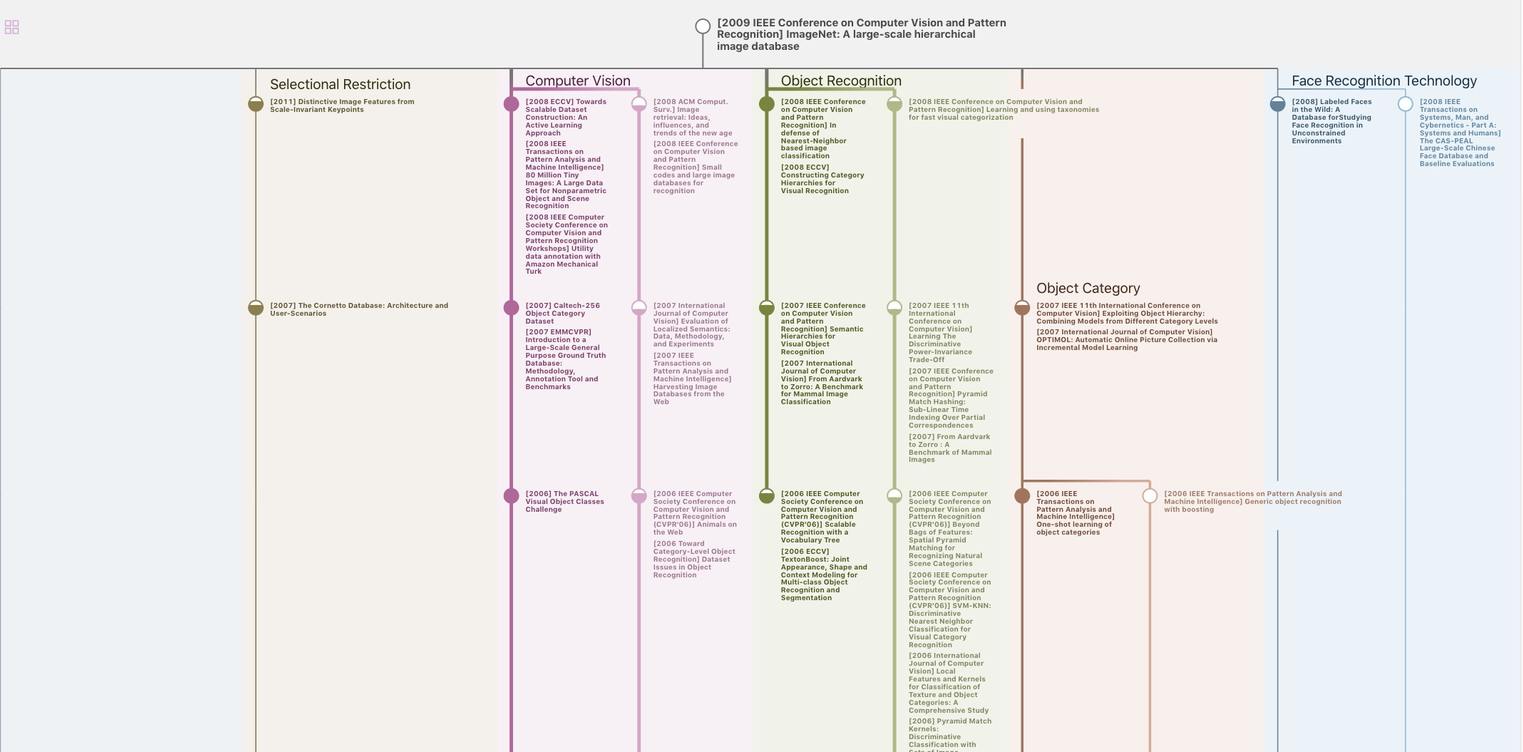
生成溯源树,研究论文发展脉络
Chat Paper
正在生成论文摘要