Learning interpretable control inputs and dynamics underlying animal locomotion
ICLR 2024(2024)
摘要
A central objective in neuroscience is to understand how the brain orchestrates movement. Recent advances in automated tracking technologies have made it possible to document behavior with unprecedented temporal resolution and scale, generating rich datasets which can be exploited to gain insights into the neural control of movement. One common approach is to identify stereotypical motor primitives using cluster analysis. However, this categorical description can limit our ability to model the effect of more continuous control schemes. Here we take a control theoretic approach to behavioral modeling and argue that movements can be understood as the output of a controlled dynamical system. Previously, models of movement dynamics, trained solely on behavioral data, have been effective in reproducing observed features of neural activity. These models addressed specific scenarios where animals were trained to execute particular movements upon receiving a prompt. In this study, we extend this approach to analyze the full natural locomotor repertoire of an animal: the zebrafish larva. Our findings demonstrate that this repertoire can be effectively generated through a sparse control signal driving a latent Recurrent Neural Network (RNN). Our model's learned latent space preserves key kinematic features and disentangles different categories of movements. To further interpret the latent dynamics, we used balanced model reduction to yield a simplified model. Collectively, our methods serve as a case study for interpretable system identification, and offer a novel framework for understanding neural activity in relation to movement.
更多查看译文
关键词
computational neuroscience,interpretable dynamics,motor control,animal behavior,dynamical systems,system identification,unsupervised learning,zebrafish
AI 理解论文
溯源树
样例
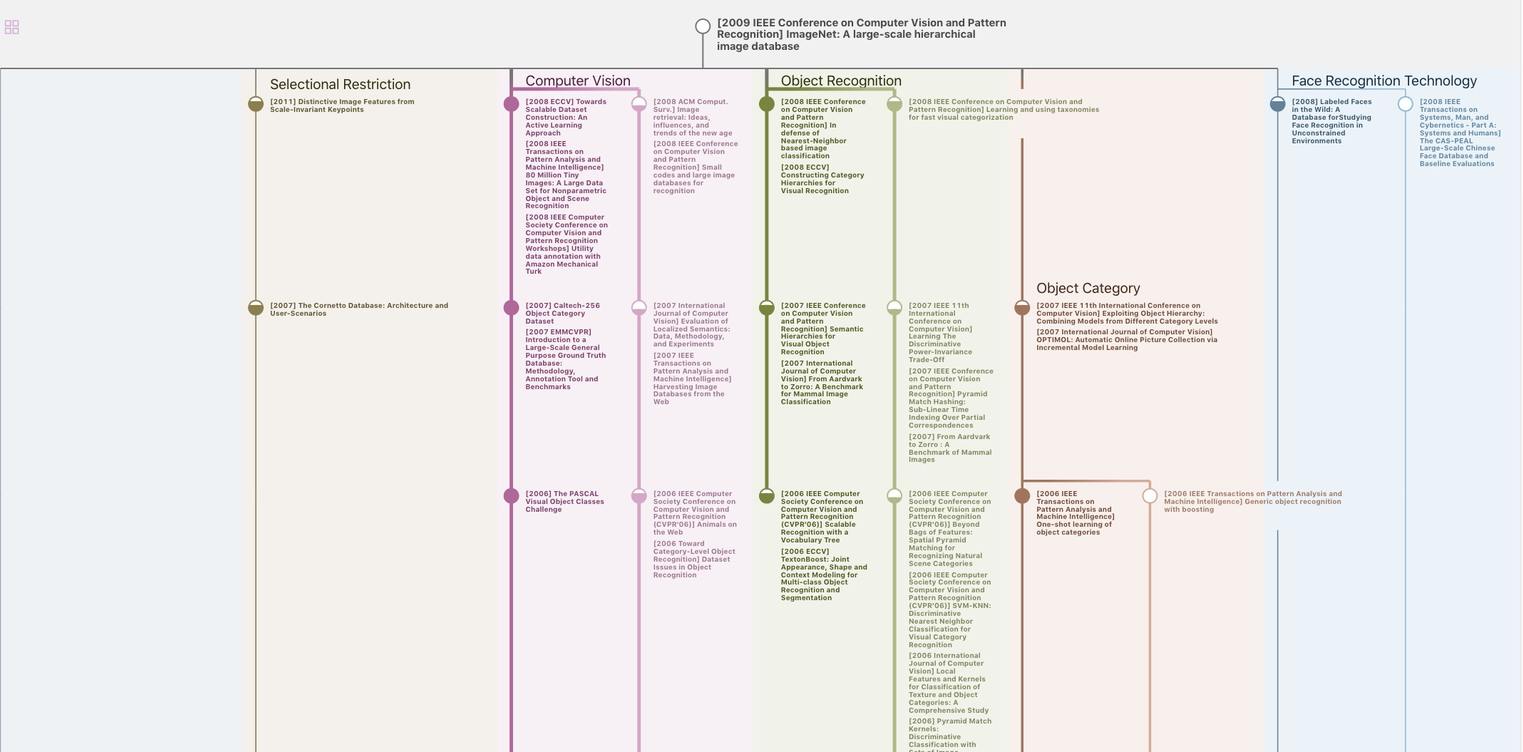
生成溯源树,研究论文发展脉络
Chat Paper
正在生成论文摘要