Canonpipe: Data Debugging with Shapley Importance over Machine Learning Pipelines
ICLR 2024(2024)
摘要
When a machine learning (ML) model exhibits poor quality (e.g., poor accuracy or fairness), the problem can often be traced back to errors in the training data. Being able to discover the data examples that are the most likely culprits is a fundamental concern that has received a lot of attention recently. One prominent way to measure "data importance" with respect to model quality is the Shapley value. Unfortunately, existing methods only focus on the ML model in isolation, without considering the broader ML pipeline for data preparation and feature extraction, which appears in the majority of real-world ML code. This presents a major limitation to applying existing methods in practical settings. In this paper, we propose Canonpipe, a method for efficiently computing Shapley-based data importance over ML pipelines. We introduce several approximations that lead to dramatic improvements in terms of computational speed. Finally, our experimental evaluation demonstrates that our methods are capable of data error discovery that is as effective as existing Monte Carlo baselines, and in some cases even outperform them.
更多查看译文
关键词
data debugging,data valuation,shapley value,machine learning pipelines
AI 理解论文
溯源树
样例
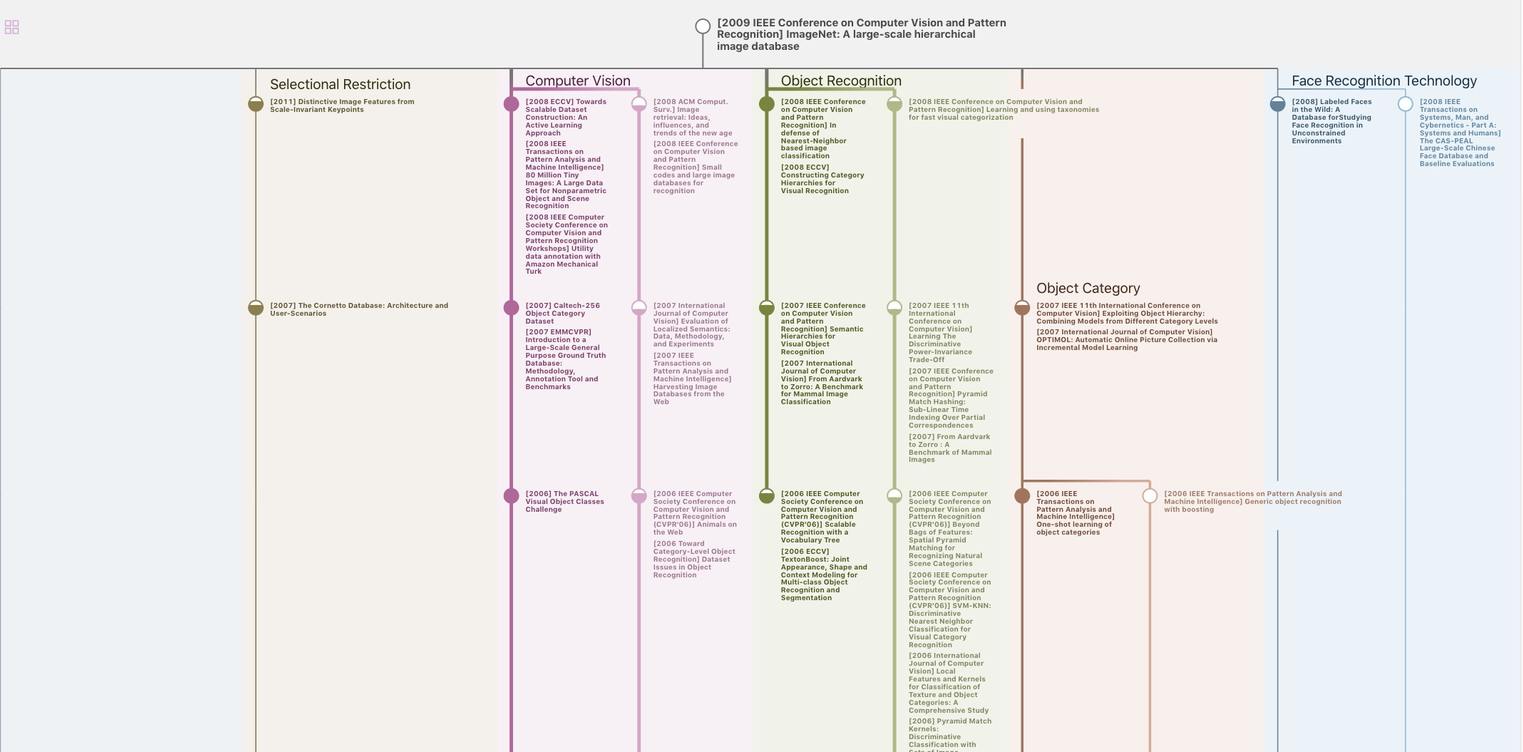
生成溯源树,研究论文发展脉络
Chat Paper
正在生成论文摘要