Federated Learning-based Privacy-aware Location Prediction Model for Internet of Vehicular Things
IEEE Transactions on Vehicular Technology(2024)
摘要
In the contemporary landscape of vehicular communications, the role of vehicular ad-hoc networks (VANETs) has become increasingly pivotal, transcending the capabilities of traditional mobile ad-hoc networks (MANETs). These advancements in VANETs play a critical role in enhancing traffic management systems, promoting collision prevention, bolstering road safety, and efficiently handling emergency scenarios. Modern vehicles, equipped with advanced data collection tools, accumulate extensive information encompassing vehicle health, fuel requirements, and comprehensive location histories. This rich data repository is instrumental in forecasting future destinations and facilitating timely arrangements, embodying the essence of ambient intelligence within the Internet of Things (IoT) framework. In emergency contexts, the rapid analysis of vehicle data is crucial for identifying the nearest emergency facilities. This paper proposes an innovative approach that leverages machine learning and edge computing techniques to predict vehicles' subsequent locations using large-scale data, concurrently prioritizing user privacy. We employ federated learning for processing at the network's edge and integrate a blockchain-based distributed database to ensure robust data privacy and security. The application of blockchain and federated learning in training models on expansive datasets is particularly effective in estimating the proximity to medical facilities and emergency services. Furthermore, this study introduces an optimization method to monitor vehicle speed and outlines a comprehensive attack model, along with effective protection measures.
更多查看译文
关键词
Large-Scale Data Models,Blockchain,Federated Learning,Location Prediction,Internet of Vehicles
AI 理解论文
溯源树
样例
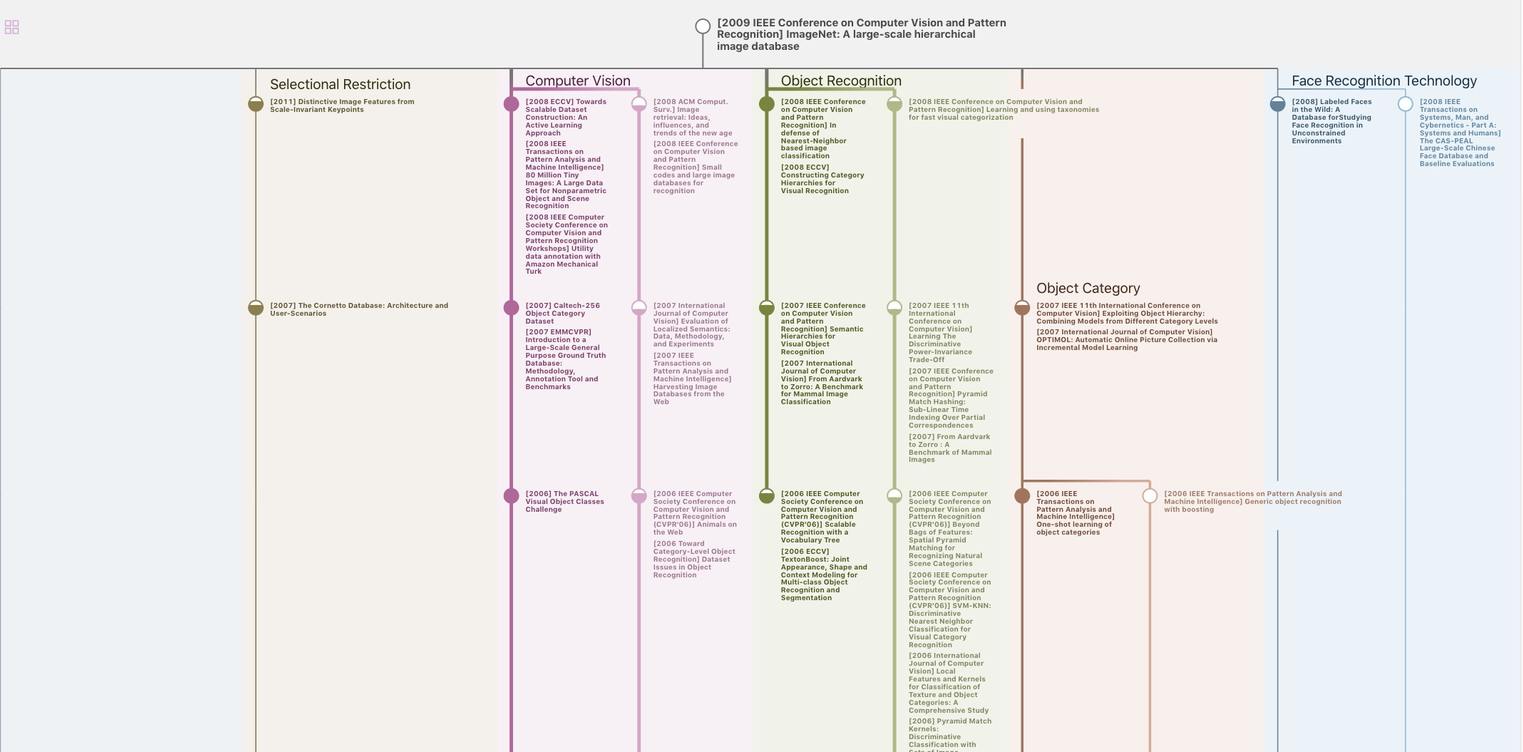
生成溯源树,研究论文发展脉络
Chat Paper
正在生成论文摘要