GNeRP: Gaussian-guided Neural Reconstruction of Reflective Objects with Noisy Polarization Priors
ICLR 2024(2024)
摘要
Learning surfaces from neural radiance field (NeRF) became a rising topic in
Multi-View Stereo (MVS). Recent Signed Distance Function (SDF)-based methods
demonstrated their ability to reconstruct accurate 3D shapes of Lambertian
scenes. However, their results on reflective scenes are unsatisfactory due to
the entanglement of specular radiance and complicated geometry. To address the
challenges, we propose a Gaussian-based representation of normals in SDF
fields. Supervised by polarization priors, this representation guides the
learning of geometry behind the specular reflection and captures more details
than existing methods. Moreover, we propose a reweighting strategy in the
optimization process to alleviate the noise issue of polarization priors. To
validate the effectiveness of our design, we capture polarimetric information,
and ground truth meshes in additional reflective scenes with various geometry.
We also evaluated our framework on the PANDORA dataset. Comparisons prove our
method outperforms existing neural 3D reconstruction methods in reflective
scenes by a large margin.
更多查看译文
关键词
Neural 3D Reconstruction,Specular Reflection
AI 理解论文
溯源树
样例
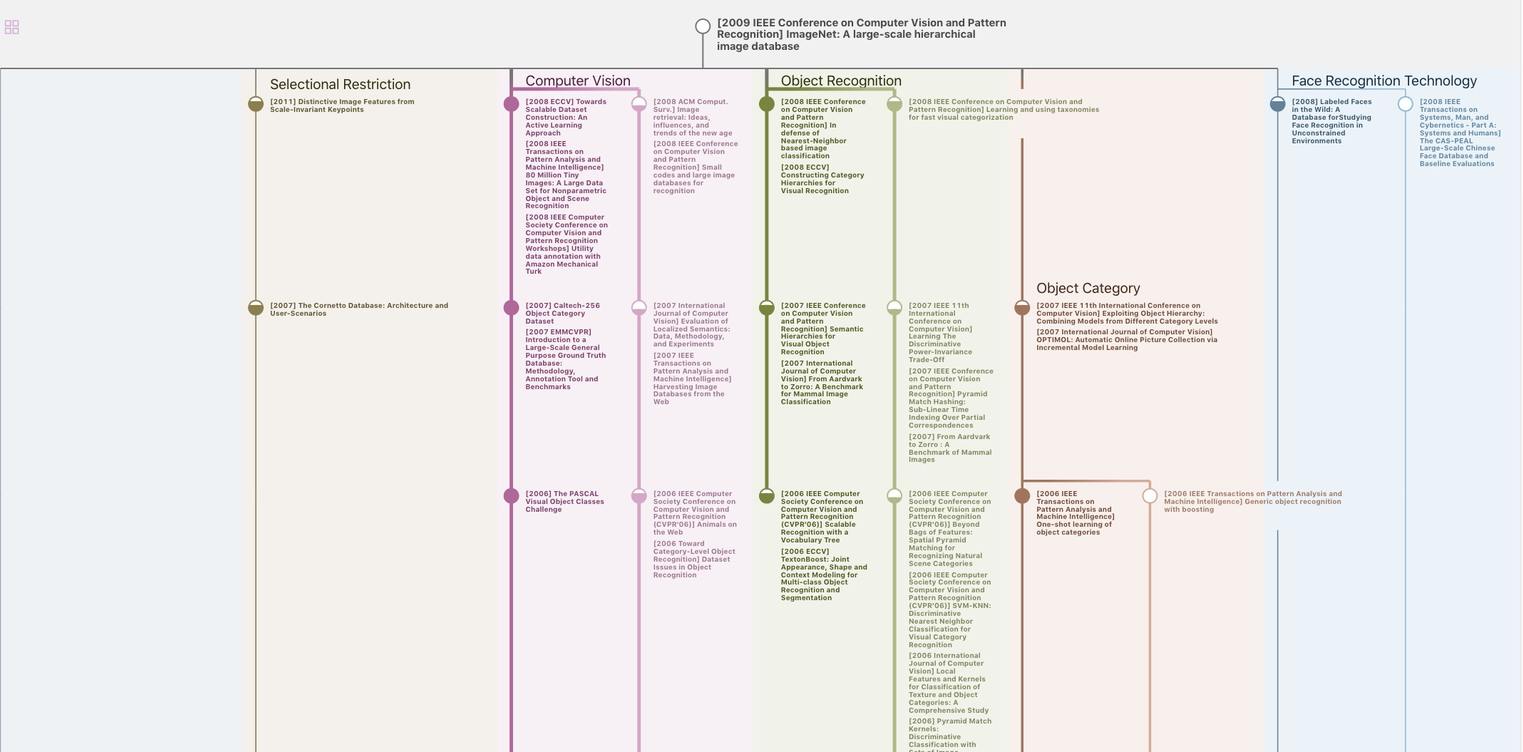
生成溯源树,研究论文发展脉络
Chat Paper
正在生成论文摘要