FedTrans: Client-Transparent Utility Estimation for Robust Federated Learning
ICLR 2024(2024)
摘要
Federated Learning (FL) is an important privacy-preserving learning paradigm that plays an important role in the Intelligent Internet of Things. Training a global model in FL, however, is vulnerable to the noise in the heterogeneous data across the clients. In this paper, we introduce **FedTrans**, a novel client-transparent client utility estimation method designed to guide client selection for noisy scenarios, mitigating performance degradation problems. To estimate the client utility, we propose a Bayesian framework that models client utility and its relationships with the weight parameters and the performance of local models. We then introduce a variational inference algorithm to effectively infer client utility, given only a small amount of auxiliary data. Our evaluation demonstrates that leveraging FedTrans as a guide for client selection can lead to a better accuracy performance (up to 7.8\%), ensuring robustness in noisy scenarios.
更多查看译文
关键词
Federated Learning
AI 理解论文
溯源树
样例
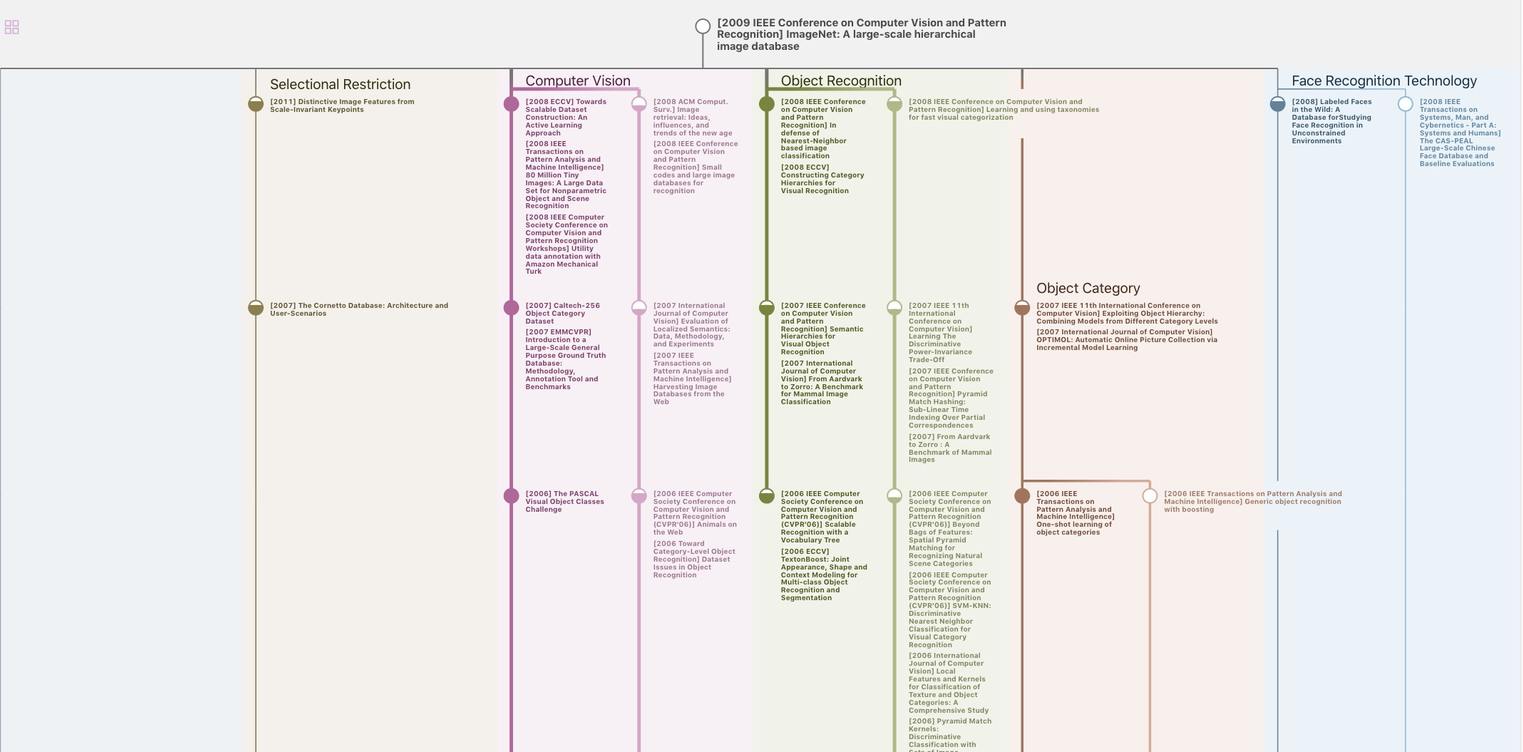
生成溯源树,研究论文发展脉络
Chat Paper
正在生成论文摘要