The Discovery of Binding Modes Requires Rethinking Docking Generalization
ICLR 2024(2024)
摘要
Accurate blind docking has the potential to lead to new biological breakthroughs, but for this promise to be realized, it is critical that docking methods generalize well across the proteome. However, existing benchmarks fail to rigorously assess generalizability. Therefore, we develop DockGen, a new benchmark based on the ligand-binding domains of proteins, and we show that machine learning-based docking models have very weak generalization abilities even when combined with various data augmentation strategies. Instead, we propose Confidence Bootstrapping, a new training paradigm that solely relies on the interaction between a diffusion and a confidence model. Unlike previous self-training methods from other domains, we directly exploit the multi-resolution generation process of diffusion models using rollouts and confidence scores to reduce the generalization gap. We demonstrate that Confidence Bootstrapping significantly improves the ability of ML-based docking methods to dock to unseen protein classes, edging closer to accurate and generalizable blind docking methods.
更多查看译文
关键词
generalization,molecular docking,protein-ligand binding,diffusion models,benchmark,bootstrapping,self-training
AI 理解论文
溯源树
样例
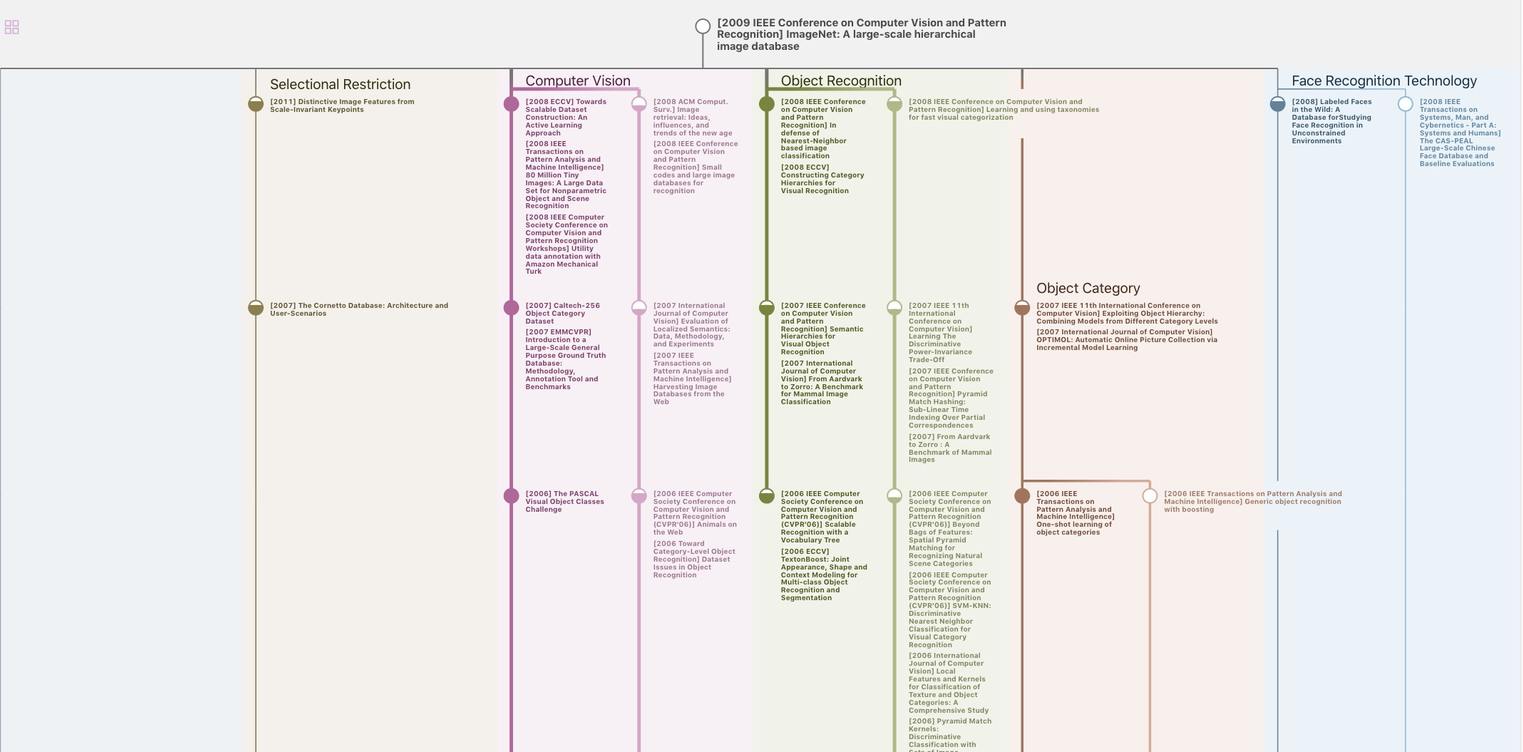
生成溯源树,研究论文发展脉络
Chat Paper
正在生成论文摘要