Evolutionary Large-Scale Multiobjective Optimization via Autoencoder-Based Problem Transformation
IEEE TRANSACTIONS ON EMERGING TOPICS IN COMPUTATIONAL INTELLIGENCE(2024)
摘要
Addressing the challenge of efficiently handling high-dimensional search spaces in solving large-scale multiobjective optimization problems (LMOPs) becomes an emerging research topic in evolutionary computation. In response, this paper proposes a new evolutionary optimizer with a tactic of autoencoder-based problem transformation (APT). The APT involves creating an autoencoder to learn the relative importance of each variable by competitively reconstructing the dominated and non-dominated solutions. Using the learned importance, all variables are divided into multiple groups without consuming any function evaluations. The number of groups dynamically increases according to the population's evolutionary status. Each variable group has an associated autoencoder, transforming the search space into an adaptable small-scale representation space. Thus, the search process occurs within these dynamic representation spaces, leading to effective production of offspring solutions. To assess the effectiveness of APT, extensive testing is performed on benchmark suites and real-world LMOPs, encompassing variable sizes ranging from 10(3) to 10(4). The comparative results demonstrate the advantages of our proposed optimizer in solving these LMOPs with a limited budget of 10(5) function evaluations.
更多查看译文
关键词
Evolutionary algorithm,large-scale multiobjective optimization,autoencoder,problem transformation
AI 理解论文
溯源树
样例
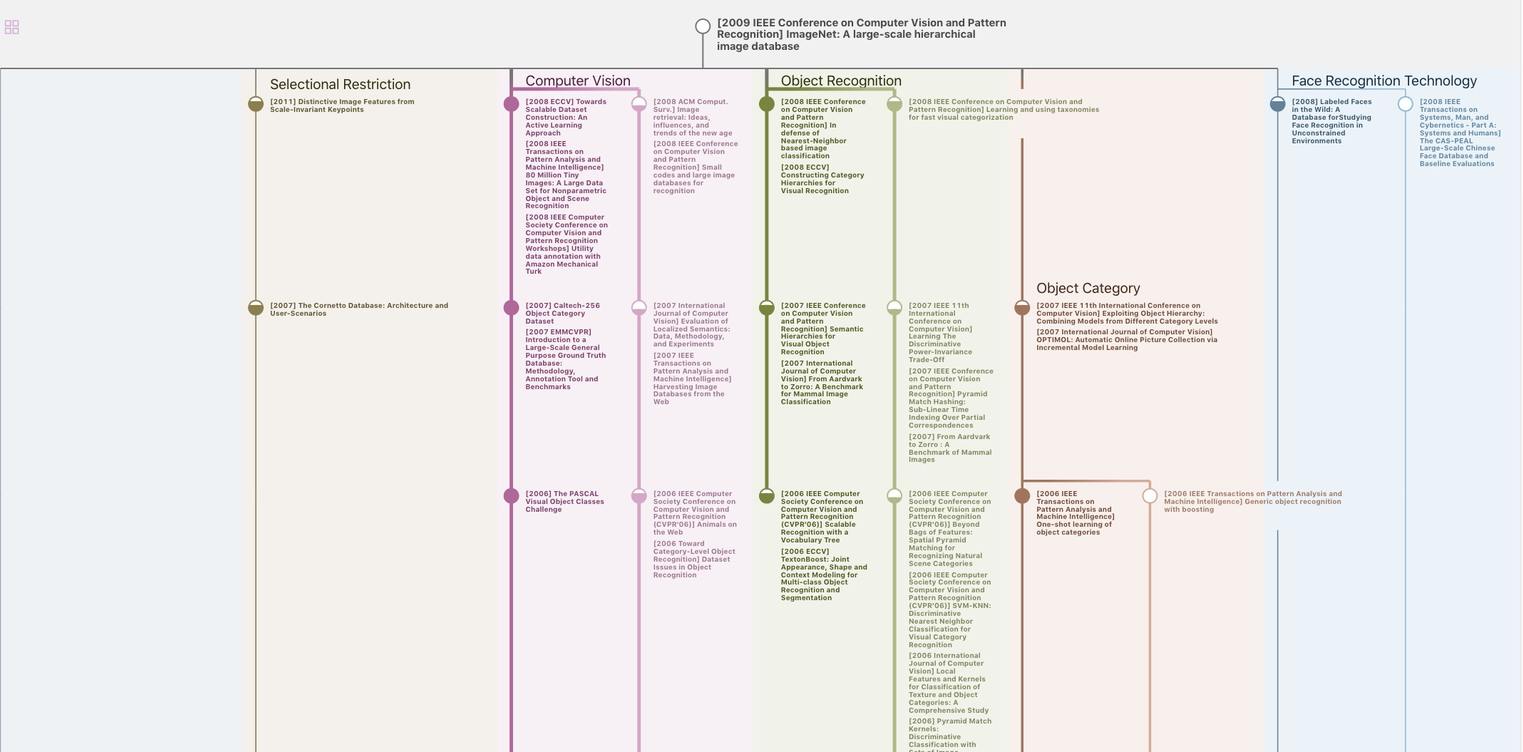
生成溯源树,研究论文发展脉络
Chat Paper
正在生成论文摘要