A machine learning paradigm for necessary observations to reduce uncertainties in aerosol climate forcing
crossref(2024)
摘要
Abstract Uncertainties in estimates of climate cooling by anthropogenic aerosols have not decreased significantly in the last two decades, largely because observational constraints on crucial aerosol properties simulated in Earth System Models (ESMs) are insufficient. We describe a new machine learning (ML) based paradigm for deriving vertically-resolved aerosol properties that will help address this insufficiency in aerosol observations. Our paradigm uses high-accuracy suborbital lidar and in situ measurements to train advanced ML algorithms to predict higher-level aerosol properties from lidar observations only, with reanalysis data providing additional constraints. We use simulated UV-only observations as input to our ML algorithms to preview their predictive capabilities in anticipation of the ATLID (ATmospheric LIDar) system on the EarthCARE (Earth Cloud, Aerosol and Radiation Explorer) satellite, which will be launched in May 2024. For the simulated ATLID data, we show that our algorithms predict two crucial aerosol properties, aerosol light absorption (ABS) and cloud condensation nuclei (CCN) concentrations with unprecedented accuracy, yielding mean relative errors of 25% and 23%, respectively. These errors represent significant improvements over conventional aerosol retrievals. Applied to future satellite missions, the new paradigm has great potential for constraining ESMs and reducing uncertainties in their estimates of aerosol climate forcing and future global warming.
更多查看译文
AI 理解论文
溯源树
样例
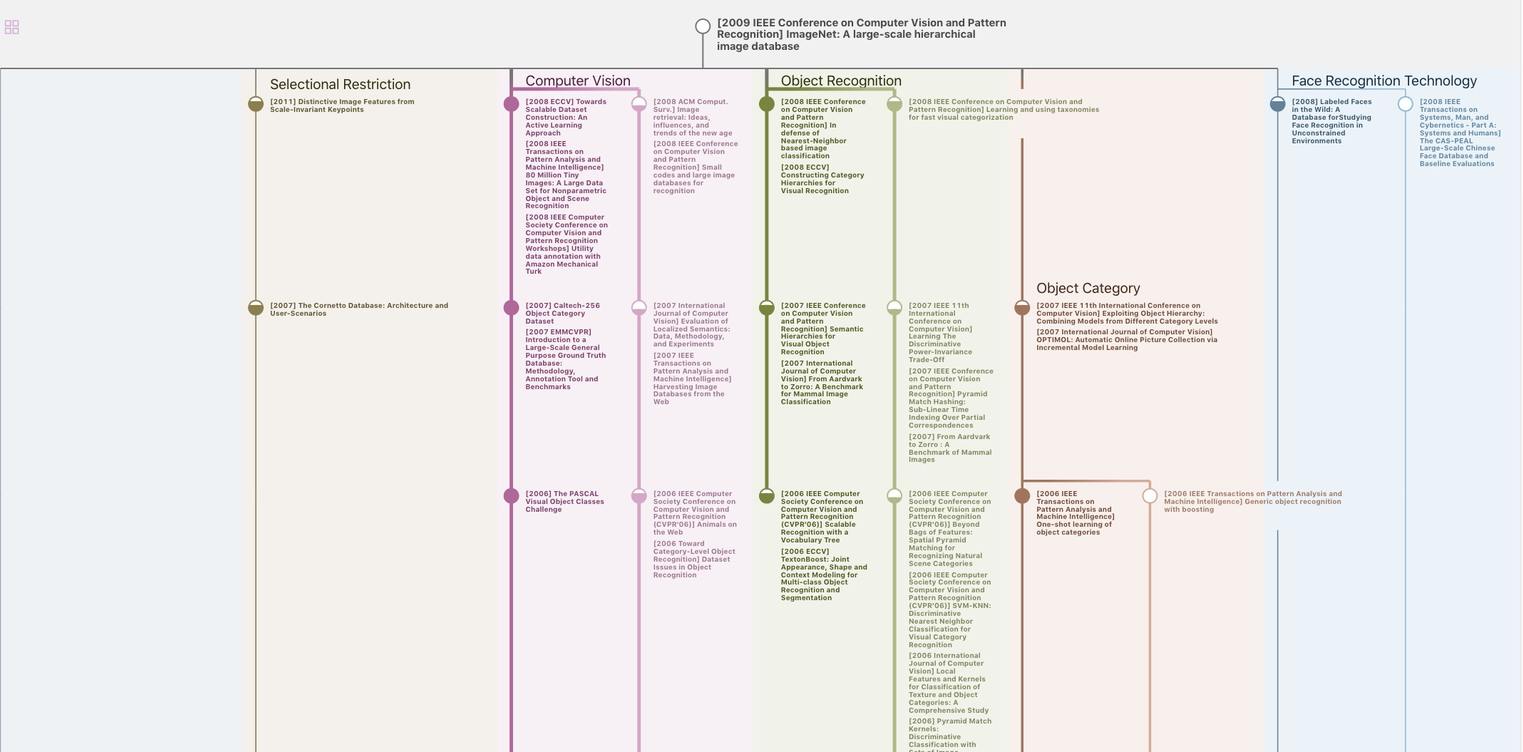
生成溯源树,研究论文发展脉络
Chat Paper
正在生成论文摘要