MMDB: Multimodal dual-branch model for multi-functional bioactive peptide prediction
Analytical Biochemistry(2024)
摘要
Bioactive peptides can hinder oxidative processes and microbial spoilage in foodstuffs and play important roles in treating diverse diseases and disorders. While most of the methods focus on single-functional bioactive peptides and have obtained promising prediction performance, it is still a significant challenge to accurately detect complex and diverse functions simultaneously with the quick increase of multi-functional bioactive peptides. In contrast to previous research on multi-functional bioactive peptide prediction based solely on sequence, we propose a novel multimodal dual-branch (MMDB) lightweight deep learning model that designs two different branches to effectively capture the complementary information of peptide sequence and structural properties. Specifically, a multi-scale dilated convolution with Bi-LSTM branch is presented to effectively model the different scales sequence properties of peptides while a multi-layer convolution branch is proposed to capture structural information. To the best of our knowledge, this is the first effective extraction of peptide sequence features using multi-scale dilated convolution without parameter increase. Multimodal features from both branches are integrated via a fully connected layer for multi-label classification. Compared to state-of-the-art methods, our MMDB model exhibits competitive results across metrics, with a 9.1% Coverage increase and 5.3% and 3.5% improvements in Precision and Accuracy, respectively.
更多查看译文
关键词
Multi-functional bioactive peptide prediction,Multimodal learning,Multi-scale dilated convolution,Peptide sequence,Peptide structural information
AI 理解论文
溯源树
样例
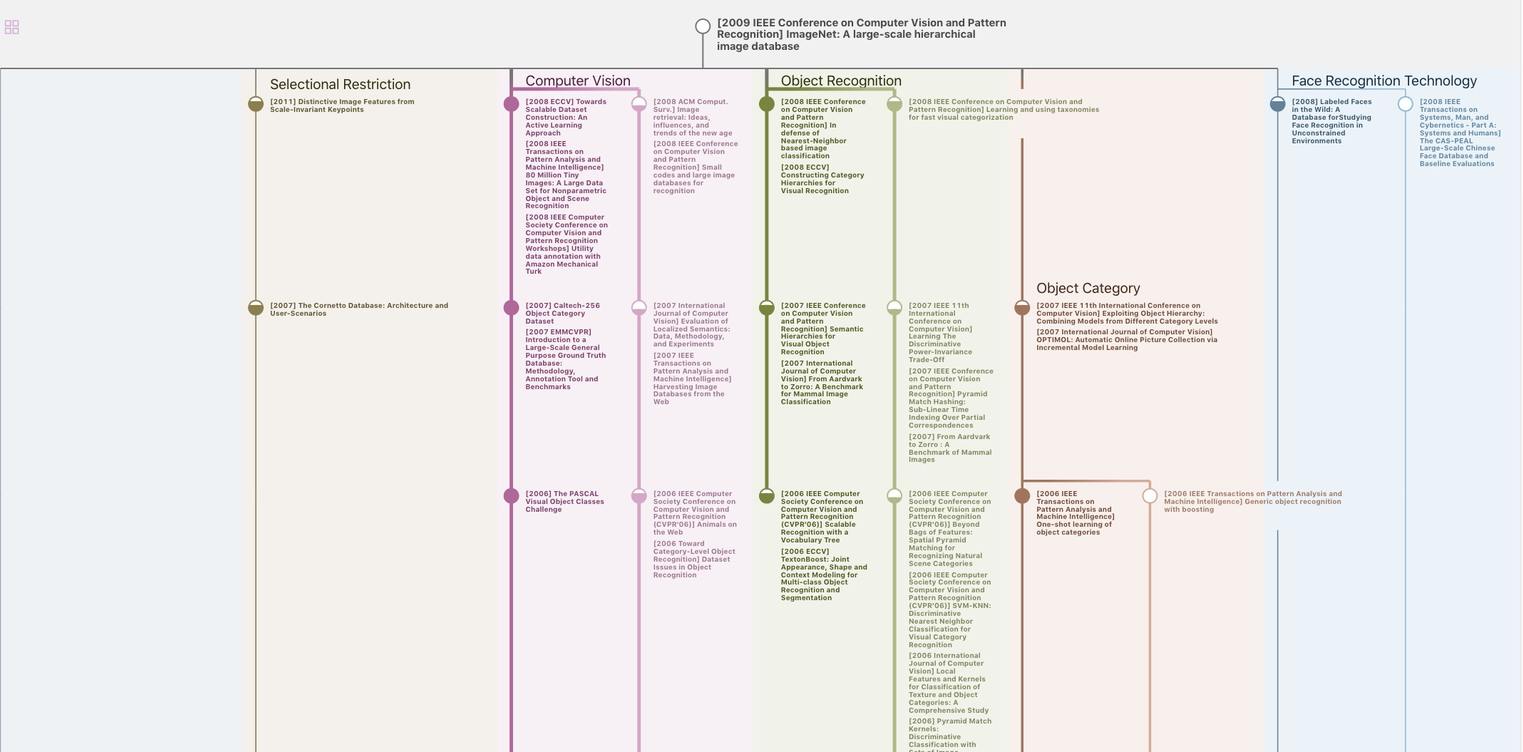
生成溯源树,研究论文发展脉络
Chat Paper
正在生成论文摘要