A Latent Variable-Based Multitask Learning Approach for Degradation Modeling of Machines With Dependency and Heterogeneity.
IEEE Trans. Instrum. Meas.(2024)
摘要
Remaining useful lifetime (RUL) prediction based on degradation signals of sensors is of critical importance in prognostics and health management (PHM) of machines in manufacturing systems. The machines are often under the same operational and environmental condition and thus show dependent but heterogeneous degradation profiles. However, many existing studies ignored these characteristics among machines, leading to poor RUL prediction results especially when missing data exists. To address this issue, this paper develops a latent variable-based multi-task learning (LVMTL) approach for degradation modeling and prognostics of machines based on multiple sensor signals. Specifically, we first adopt a polynomial model to fit the degradation processes of machines’ health indices (HIs), which are estimated based on multiple sensor signals. Taking the HIs as latent variables, we develop an LVMTL model aiming to capture the dependency and heterogeneity among machines. For parameter estimation, we develop a unique iterated quadratic optimization-integrated expectation-maximum (QOIEM) algorithm that updates model parameters via quadratic programming (QP) and maximum a posteriori-based expectation-maximum (MAP-EM) considering the principles for estimating an HI. The QOIEM has been proven to have satisfactory convergence performance in theory in this study. Numerical experiments and a real case study on aircraft turbine engines have been implemented to compare the prognosis performance and robustness of incomplete datasets for the proposed and benchmark methods.
更多查看译文
关键词
Dependent but heterogeneous degradation profiles,latent variable-based multitask learning,missing data,prognostics and health management,quadratic optimization-integrated expectation-maximum algorithm
AI 理解论文
溯源树
样例
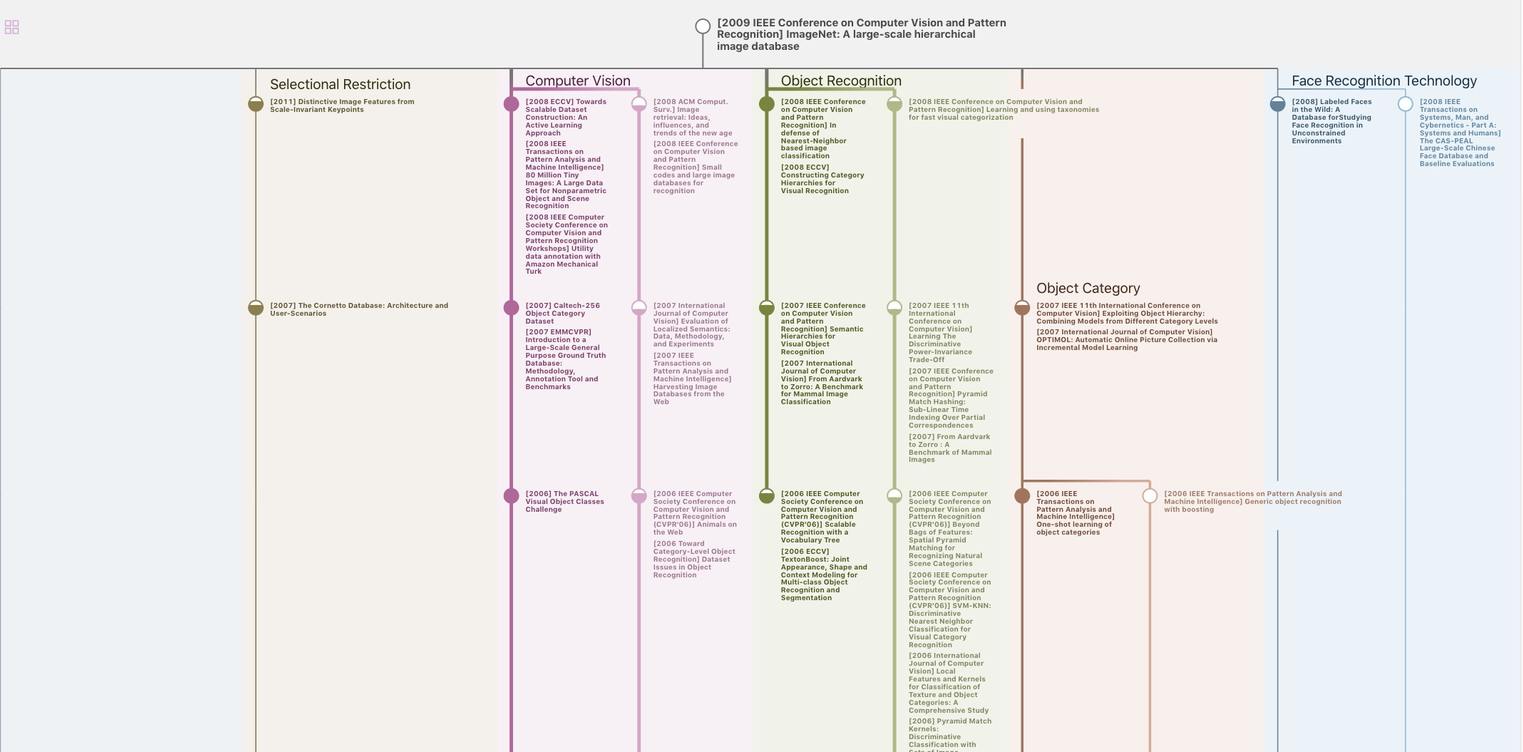
生成溯源树,研究论文发展脉络
Chat Paper
正在生成论文摘要