CL-BPUWM: continuous learning with Bayesian parameter updating and weight memory
Complex & Intelligent Systems(2024)
摘要
Catastrophic forgetting in neural networks is a common problem, in which neural networks lose information from previous tasks after training on new tasks. Although adopting a regularization method that preferentially retains the parameters important to the previous task to avoid catastrophic forgetting has a positive effect; existing regularization methods cause the gradient to be near zero because the loss is at the local minimum. To solve this problem, we propose a new continuous learning method with Bayesian parameter updating and weight memory (CL-BPUWM). First, a parameter updating method based on the Bayes criterion is proposed to allow the neural network to gradually obtain new knowledge. The diagonal of the Fisher information matrix is then introduced to significantly minimize computation and increase parameter updating efficiency. Second, we suggest calculating the importance weight by observing how changes in each network parameter affect the model prediction output. In the process of model parameter updating, the Fisher information matrix and the sensitivity of the network are used as the quadratic penalty terms of the loss function. Finally, we apply dropout regularization to reduce model overfitting during training and to improve model generalizability. CL-BPUWM performs very well in continuous learning for classification tasks on CIFAR-100 dataset, CIFAR-10 dataset, and MNIST dataset. On CIFAR-100 dataset, it is 0.8
更多查看译文
关键词
Catastrophic forgetting,Regularization,Bayesian criterion,Fisher's information matrix
AI 理解论文
溯源树
样例
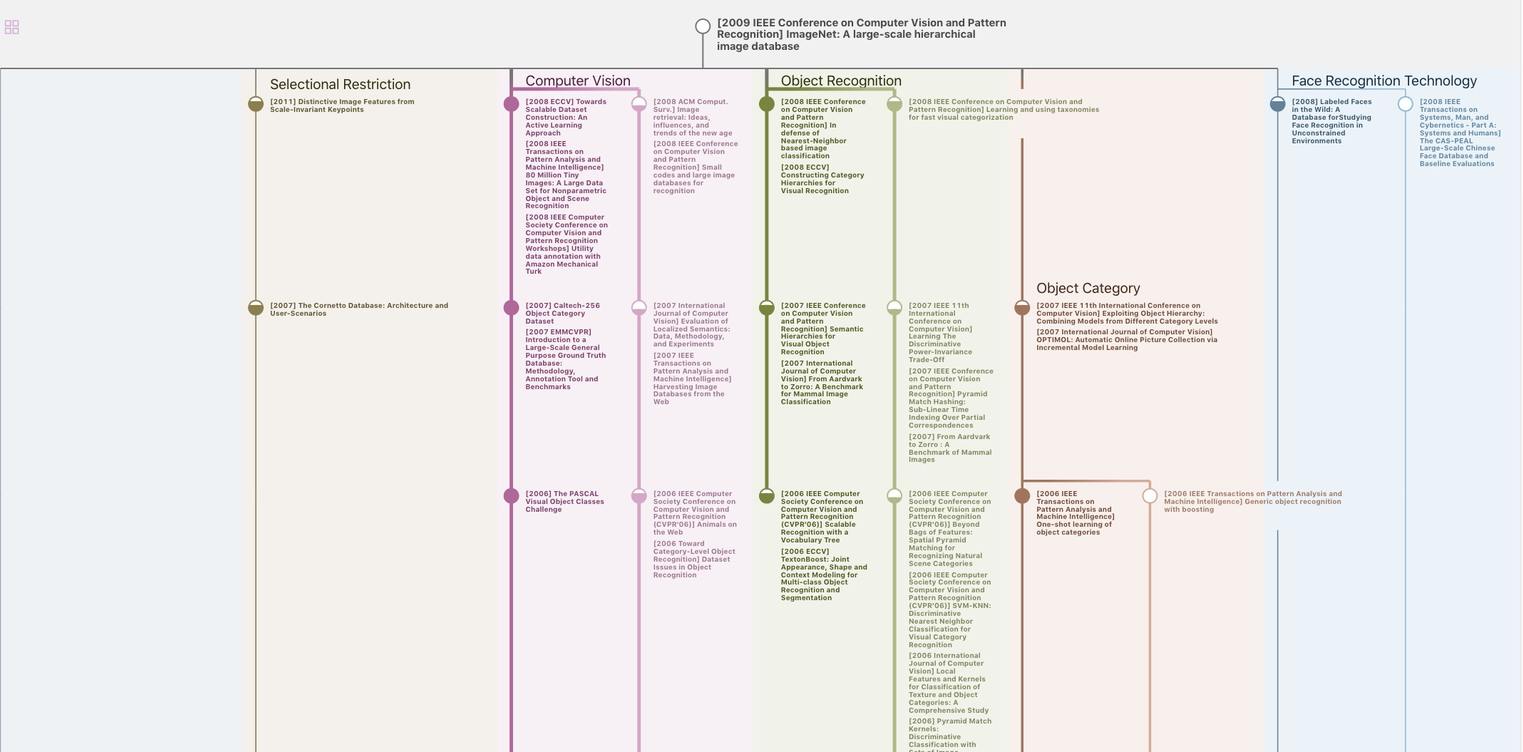
生成溯源树,研究论文发展脉络
Chat Paper
正在生成论文摘要