Spectral normalization generative adversarial networks for photovoltaic power scenario generation
IET RENEWABLE POWER GENERATION(2024)
摘要
The large-scale integration of new energy generation into the power transmission network introduces uncertainty and fluctuations, posing a threat to the secure operation of the transmission network. Modelling and analysing the uncertainty in photovoltaic (PV) generation is essential. This paper introduces a method for generating scenarios for centralized PV station based on spectral normalization generative adversarial networks (SNGAN). Data-driven scenario generation methods, with generative adversarial networks (GAN) as representatives, are commonly used today to analyse the uncertainty of new energy generation. In addressing the uncertainty of centralized PV power generation, this paper introduces SNGAN, makes improvements to the discriminator, enhances training stability, and generates PV power generation scenarios. The incorporation of spectral normalization as a regularization technique contributes to the stability and convergence of the proposed GAN model. The effectiveness of the selected method is validated through comparisons with actual data. This paper integrates the spectral normalization method into the discriminator network to enhance the training stability of the network. Through simulations of centralized photovoltaic power station scenarios, the effectiveness of the selected methods is validated. image
更多查看译文
关键词
learning (artificial intelligence),photovoltaic cells,photovoltaic power systems,uncertain systems
AI 理解论文
溯源树
样例
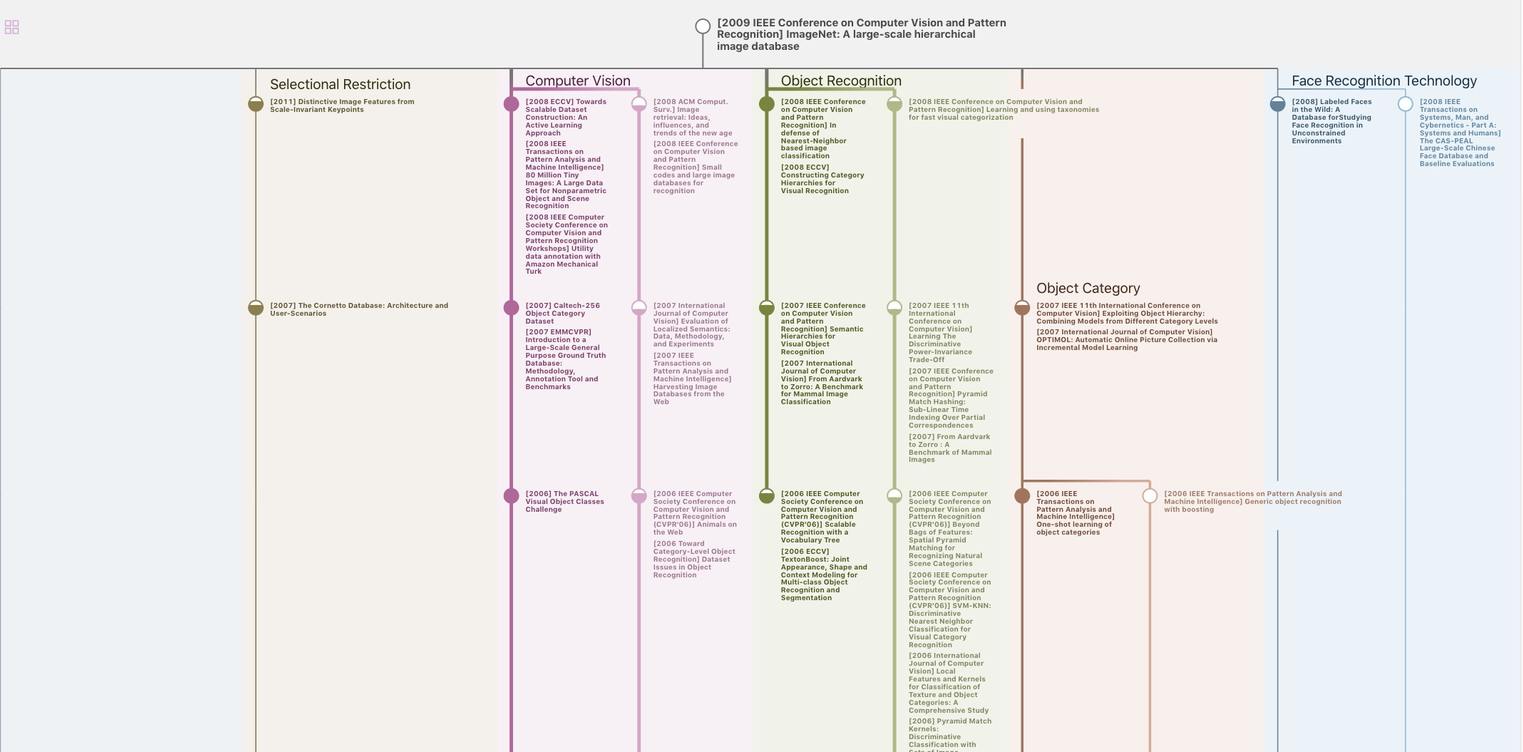
生成溯源树,研究论文发展脉络
Chat Paper
正在生成论文摘要