Efficient feature redundancy reduction for image denoising
World Wide Web(2024)
摘要
It is challenging to deploy convolutional neural networks (CNNs) for image denoising on low-power devices which can suffer from computational and memory constraints. To address this limitation, a simple yet effective and efficient feature redundancy reduction-based network (FRRN) is proposed in this paper, which integrates a feature refinement block (FRB), an attention fusion block (AFB), and an enhancement block (EB). Specifically, the FRB distills structural information via two parallel sub-networks, selecting representative feature representations while suppressing spatial-channel redundancy. The AFB absorbs an attentive fusion mechanism to facilitate diverse features extracted from two sub-networks, emphasizing texture and structure details but alleviating harmful features from problematic regions. The subsequent EB further boosts the feature representation abilities. Aiming to enhance denoising performance at both pixel level and semantic level, a multi-loss scheme comprising three popular loss functions is leveraged to improve the robustness of the denoiser. Comprehensive quantitative and qualitative analyses demonstrate the superiority of the proposed FRRN.
更多查看译文
关键词
Spatial-channel adaptive,Attentive fusion mechanism,Multi-loss scheme,Image denoising
AI 理解论文
溯源树
样例
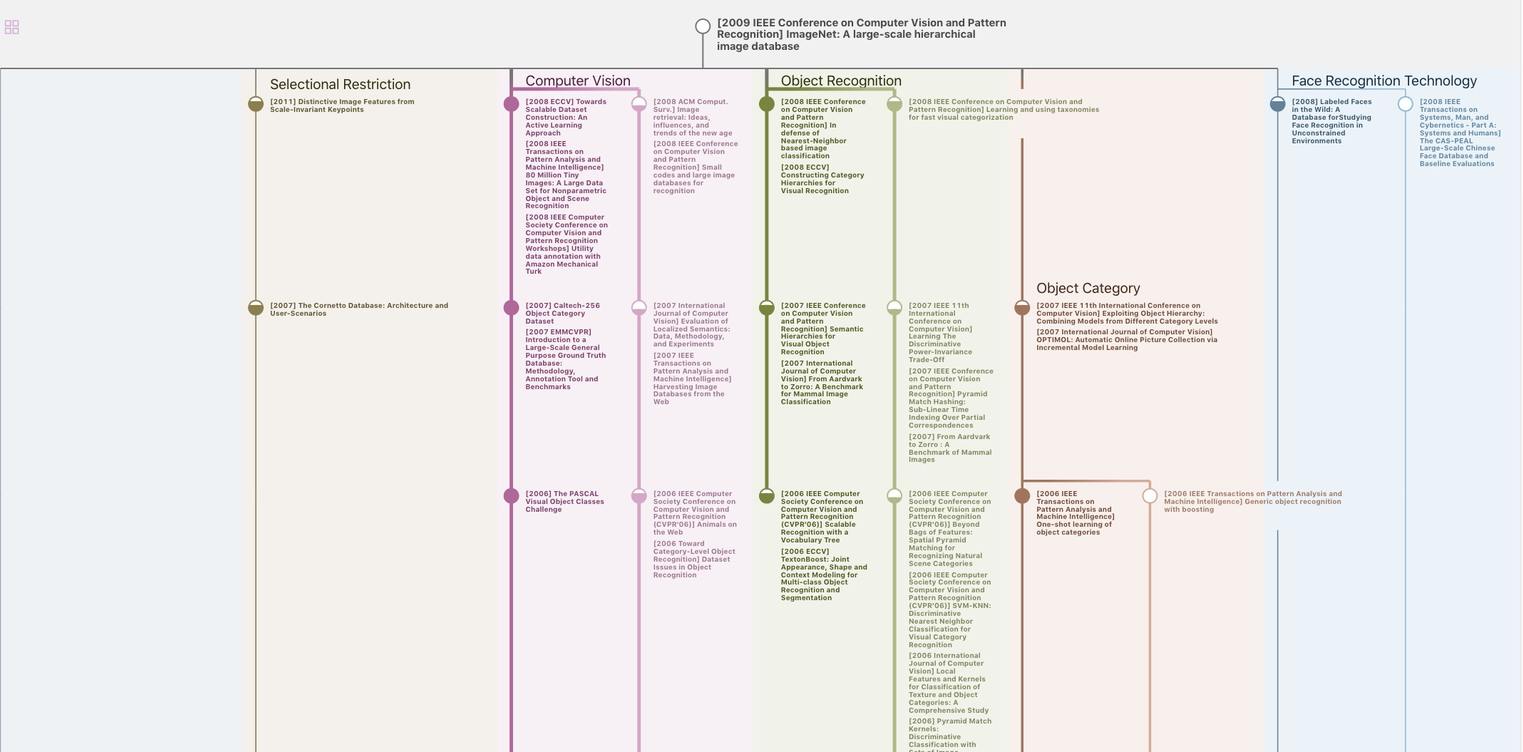
生成溯源树,研究论文发展脉络
Chat Paper
正在生成论文摘要