Pushing the Limits of WiFi Sensing with Low Transmission Rates
IEEE Transactions on Mobile Computing(2024)
摘要
Existing WiFi sensing systems transmit dedicated high-rate packets for accurate sensing. These “sensing packets” greatly affect the main data communication function of WiFi and significantly counteract the promised benefit of reusing WiFi communication for sensing. In this work, we propose WiImg2.0, a lightweight system which involves machine learning techniques to enable WiFi sensing under low packet rate, pushing WiFi sensing one step towards real-life adoption. The key idea is to convert the WiFi CSI samples into images and employ the Generative Adversarial Network (GAN) for CSI image inpainting, relaxing the requirement of high sample rate for sensing. We first recover the sensing data from the antenna spatial domain and then from the sample time domain. To avoid the large training overhead of GAN, we design a lightweight GAN that leverages samples of only three rates in a fixed window to recover the CSI traces of arbitrary rates and varying duration. Experiments show that with just 25 packets per second, WiImg2.0 is able to increase the recognition accuracy for hand gesture recognition and daily activity tracking from the state-of-the-art 59.1% and 65.9% to 86.7% and 96.4%, respectively.
更多查看译文
关键词
Channel State Information,Activity Recognition,Low Transmission Rates,GAN,WiFi
AI 理解论文
溯源树
样例
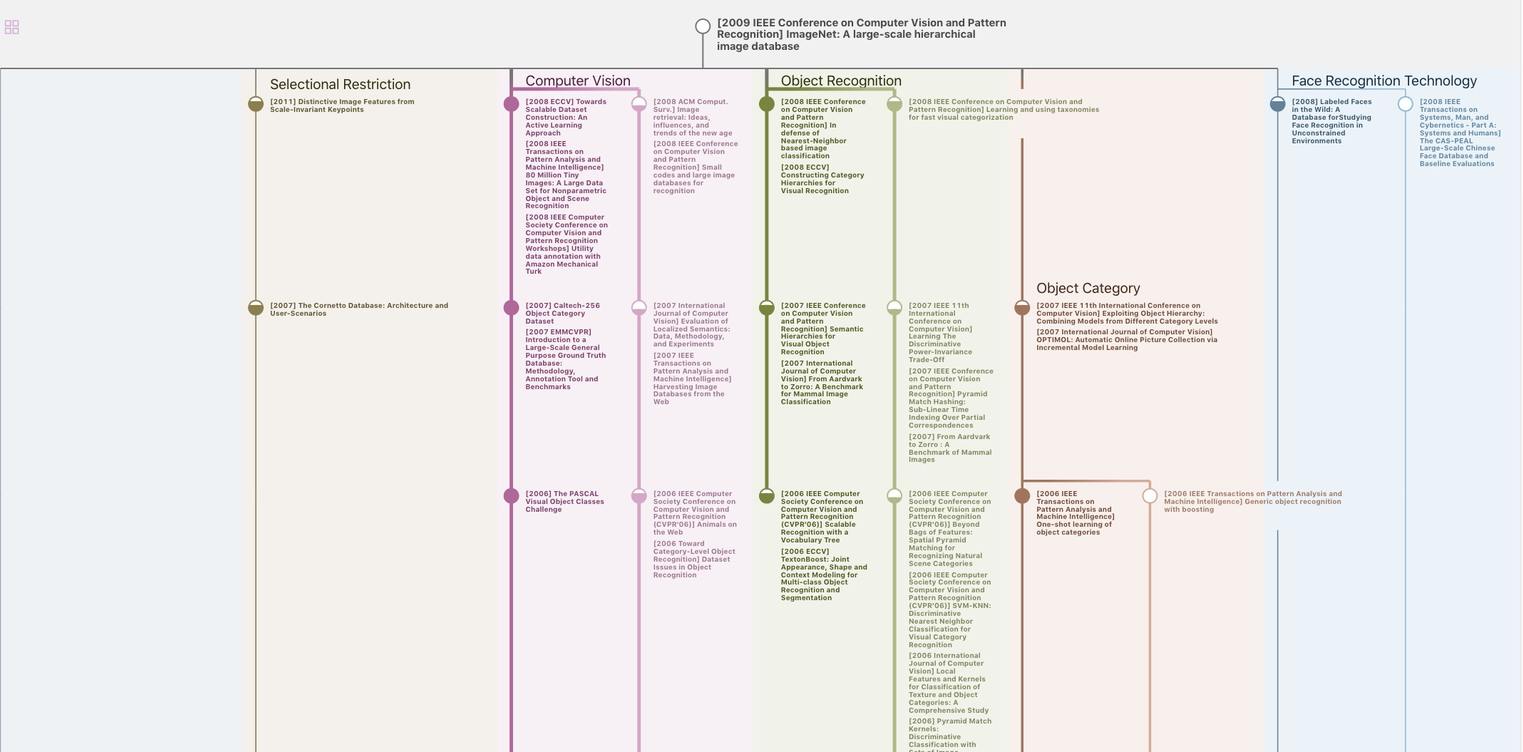
生成溯源树,研究论文发展脉络
Chat Paper
正在生成论文摘要