CMDA: Cross-Modal and Domain Adversarial Adaptation for LiDAR-Based 3D Object Detection
AAAI 2024(2024)
摘要
Recent LiDAR-based 3D Object Detection (3DOD) methods show promising results, but they often do not generalize well to target domains outside the source (or training) data distribution. To reduce such domain gaps and thus to make 3DOD models more generalizable, we introduce a novel unsupervised domain adaptation (UDA) method, called CMDA, which (i) leverages visual semantic cues from an image modality (i.e., camera images) as an effective semantic bridge to close the domain gap in the cross-modal Bird's Eye View (BEV) representations. Further, (ii) we also introduce a self-training-based learning strategy, wherein a model is adversarially trained to generate domain-invariant features, which disrupt the discrimination of whether a feature instance comes from a source or an unseen target domain. Overall, our CMDA framework guides the 3DOD model to generate highly informative and domain-adaptive features for novel data distributions. In our extensive experiments with large-scale benchmarks, such as nuScenes, Waymo, and KITTI, those mentioned above provide significant performance gains for UDA tasks, achieving state-of-the-art performance.
更多查看译文
关键词
CV: 3D Computer Vision,CV: Vision for Robotics & Autonomous Driving,CV: Object Detection & Categorization,CV: Multi-modal Vision,ML: Adversarial Learning & Robustness,ML: Transfer, Domain Adaptation, Multi-Task Learning
AI 理解论文
溯源树
样例
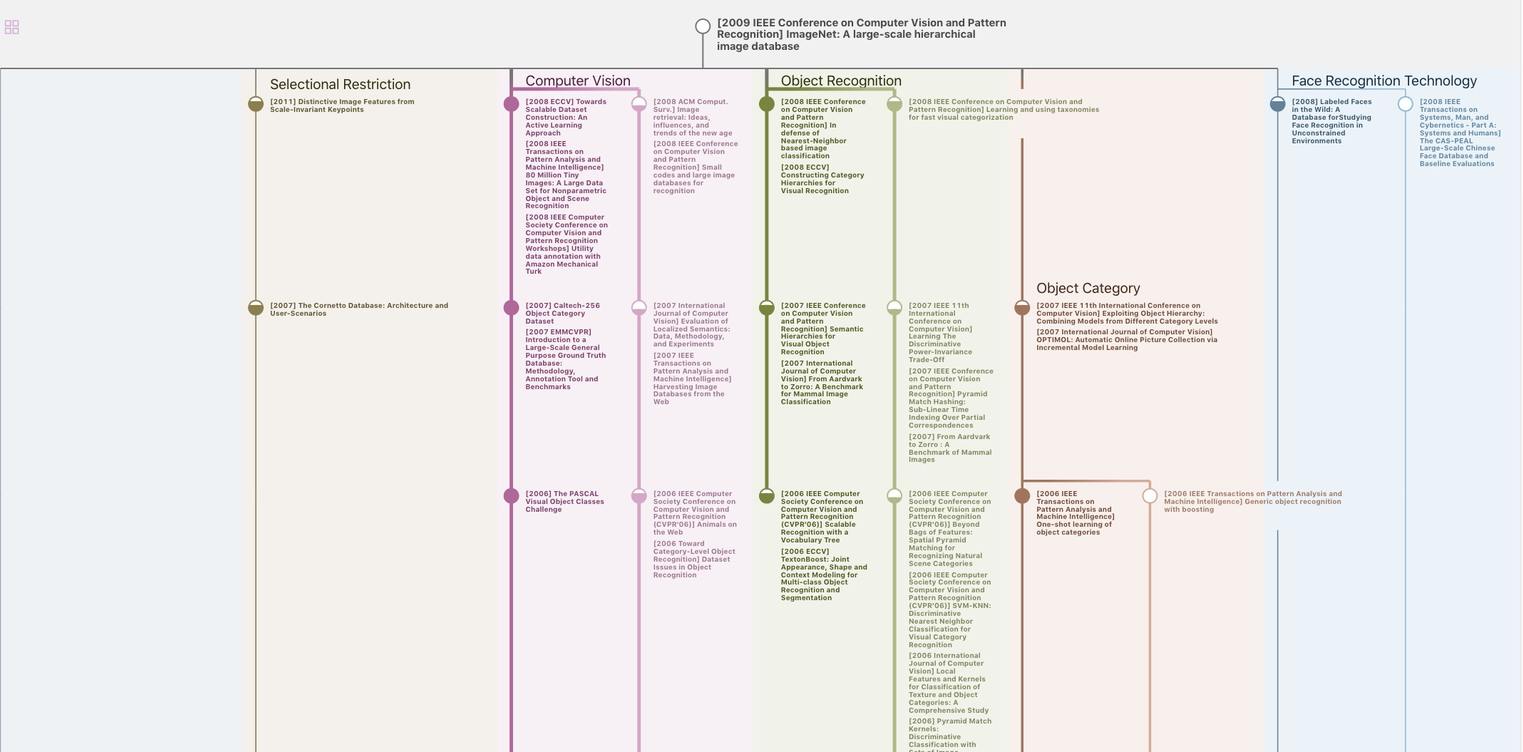
生成溯源树,研究论文发展脉络
Chat Paper
正在生成论文摘要