Coexistence of Cyclic Sequential Pattern Recognition and Associative Memory in Neural Networks by Attractor Mechanisms
IEEE TRANSACTIONS ON NEURAL NETWORKS AND LEARNING SYSTEMS(2024)
摘要
Neural networks are developed to model the behavior of the brain. One crucial question in this field pertains to when and how a neural network can memorize a given set of patterns. There are two mechanisms to store information: associative memory and sequential pattern recognition. In the case of associative memory, the neural network operates with dynamical attractors that are point attractors, each corresponding to one of the patterns to be stored within the network. In contrast, sequential pattern recognition involves the network memorizing a set of patterns and subsequently retrieving them in a specific order over time. From a dynamical perspective, this corresponds to the presence of a continuous attractor or a cyclic attractor composed of the sequence of patterns stored within the network in a given order. Evidence suggests that the brain is capable of simultaneously performing both associative memory and sequential pattern recognition. Therefore, these types of attractors coexist within the neural network, signifying that some patterns are stored as point attractors, while others are stored as continuous or cyclic attractors. This article investigates the coexistence of cyclic attractors and continuous or point attractors in certain nonlinear neural networks, enabling the simultaneous emergence of various memory mechanisms. By selectively grouping neurons, conditions are established for the existence of cyclic attractors, continuous attractors, and point attractors, respectively. Furthermore, each attractor is explicitly represented, and a competitive dynamic emerges among these coexisting attractors, primarily regulated by adjustments to external inputs.
更多查看译文
关键词
Associative memory,coexistence,competition,continuous attractors,cyclic attractors,cyclic sequential pattern recognition,point attractors,selectively grouping neurons
AI 理解论文
溯源树
样例
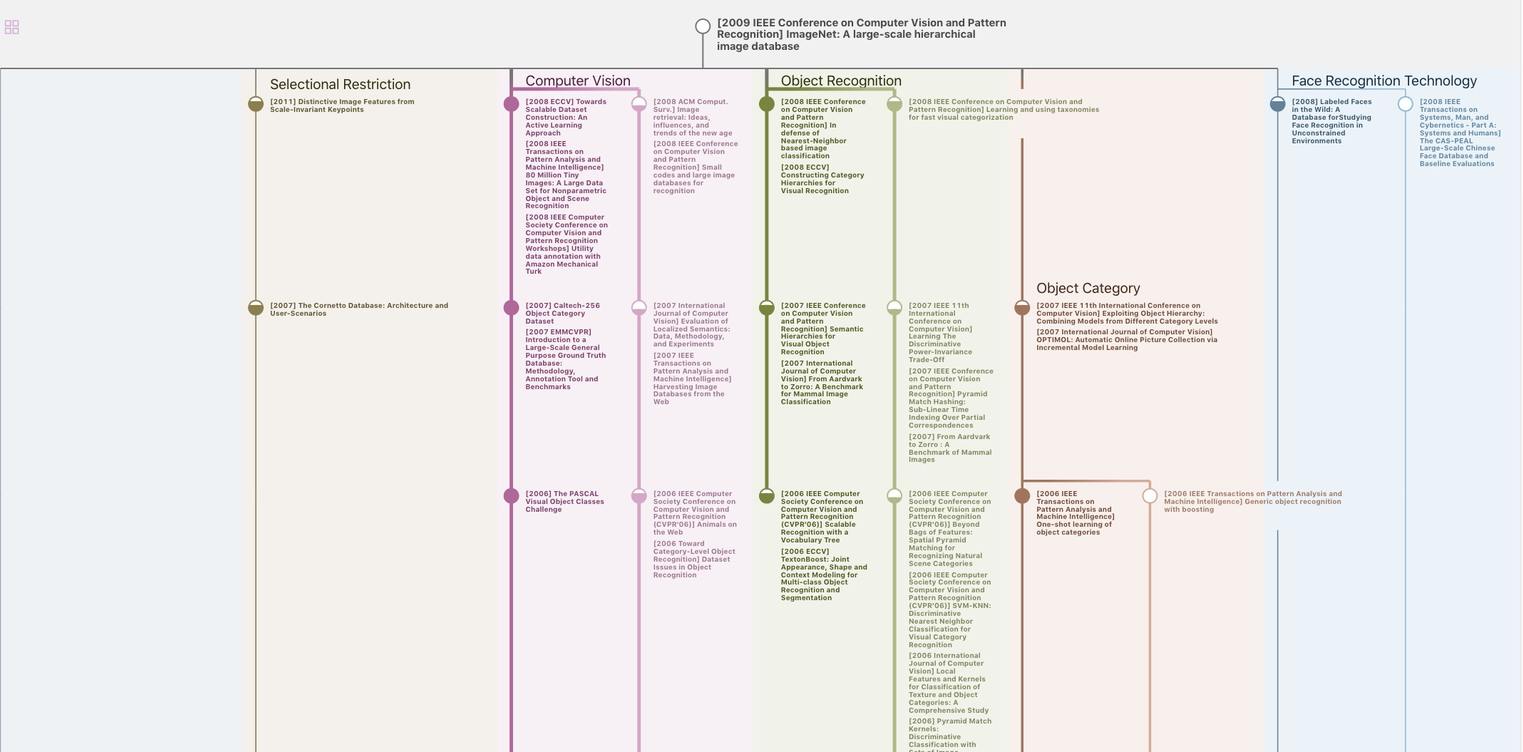
生成溯源树,研究论文发展脉络
Chat Paper
正在生成论文摘要