Mask-Guided Local-2013;Global Attentive Network for Change Detection in Remote Sensing Images
IEEE JOURNAL OF SELECTED TOPICS IN APPLIED EARTH OBSERVATIONS AND REMOTE SENSING(2024)
摘要
Change detection in remote sensing images is a challenging task due to object appearance diversity and the interference of complex backgrounds. Self-attention- and spatial-attention-based solutions face limitations, such as high memory consumption and an inadequate ability to capture long-range relations, leading to imprecise contextual information and restricted performance. To address these challenges, this article introduces a novel mask-guided local-global attentive network (MLA-Net). The MLA-Net incorporates a memory-efficient local-global attention module that leverages the benefits of both self-attention and spatial attention to accurately capture the local-global context. Through simultaneous exploitation of context within inter- and intrapatches and information refinement, the feature representation capability is significantly enhanced. In addition, we introduce a change mask to refine feature differences and eliminate interference from irrelevant changes caused by complex backgrounds. Accordingly, a mask loss is defined to guide the generation of the mask. Extensive experiments on the LEVIR-CD, WHU-CD, and CLCD datasets show that our MLA-Net performs better than state-of-the-art methods.
更多查看译文
关键词
Attention mechanism,change detection (CD),change mask,convolutional neural network (CNN),remote sensing image
AI 理解论文
溯源树
样例
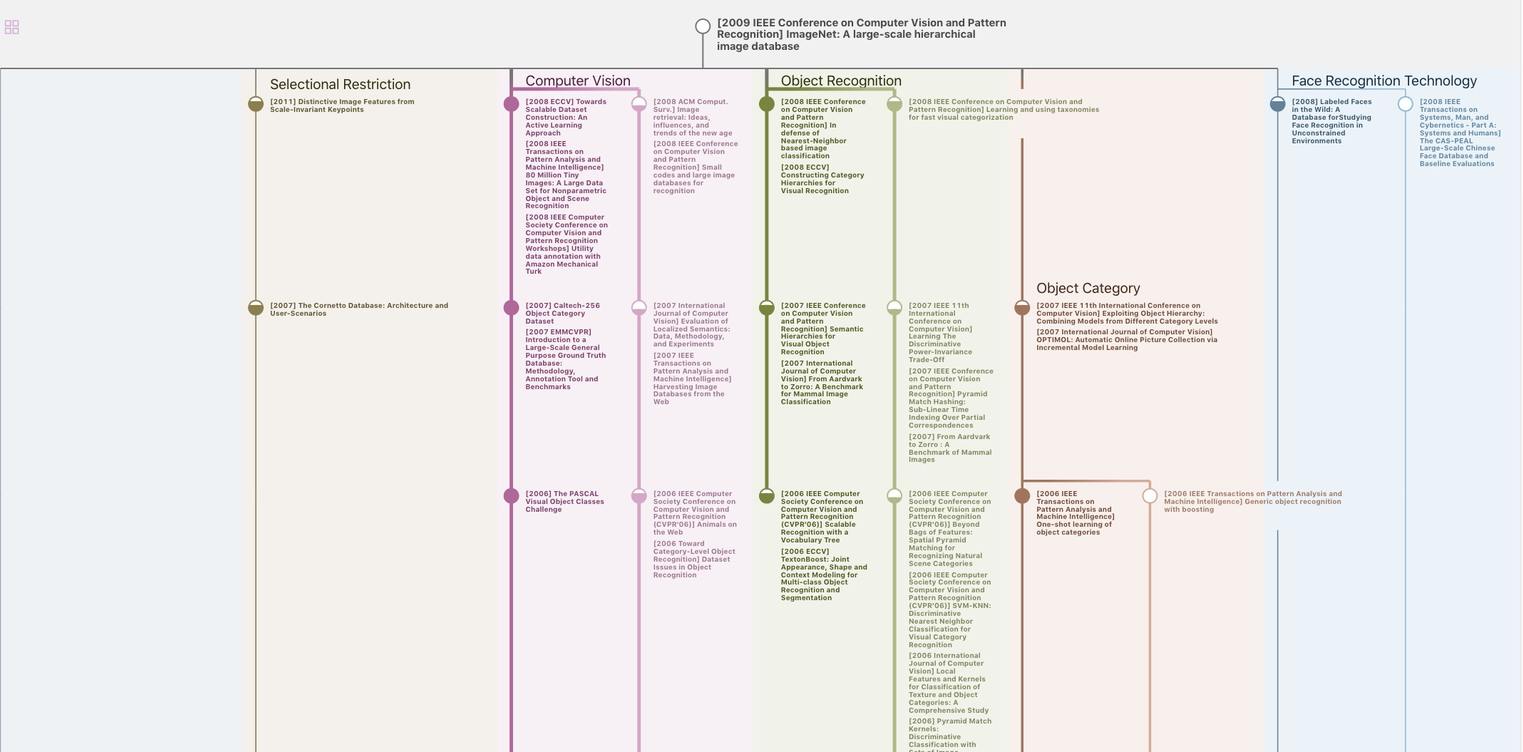
生成溯源树,研究论文发展脉络
Chat Paper
正在生成论文摘要