A graph-based approach for integrating massive data in container terminals with application to scheduling problem
INTERNATIONAL JOURNAL OF PRODUCTION RESEARCH(2024)
摘要
The deployment of the Industrial Internet of Things (IIoT) in smart container terminals provides a foundation for sensing and recording all operational processes. However, little effort has been devoted to integrating the massive data regarding interoperability challenges, thus limiting the value of data in advancing the intelligent evolution of ports. In this research, we propose a graph-based approach to organise operational records semantically, thereby facilitating data-driven decision-making in container terminals. We first construct a knowledge graph for operational processes in container terminals, employing a tailored procedure for the automatic conversion of operational records into triples. By utilising the graph information, we propose a novel method that integrates reinforcement learning (RL) with a mathematical solver for optimising scheduling problems. The quay crane scheduling problem (QCSP) is illustrated as an example to elaborate on the technical details. Based on a dataset from a real-world container terminal, numerical studies demonstrate the superiority of the proposed framework in terms of information retrieval efficiency and solution quality compared with the traditional data organisation approach.
更多查看译文
关键词
Smart container terminal,knowledge graph,operational data,mixed-integer linear programming,reinforcement learning
AI 理解论文
溯源树
样例
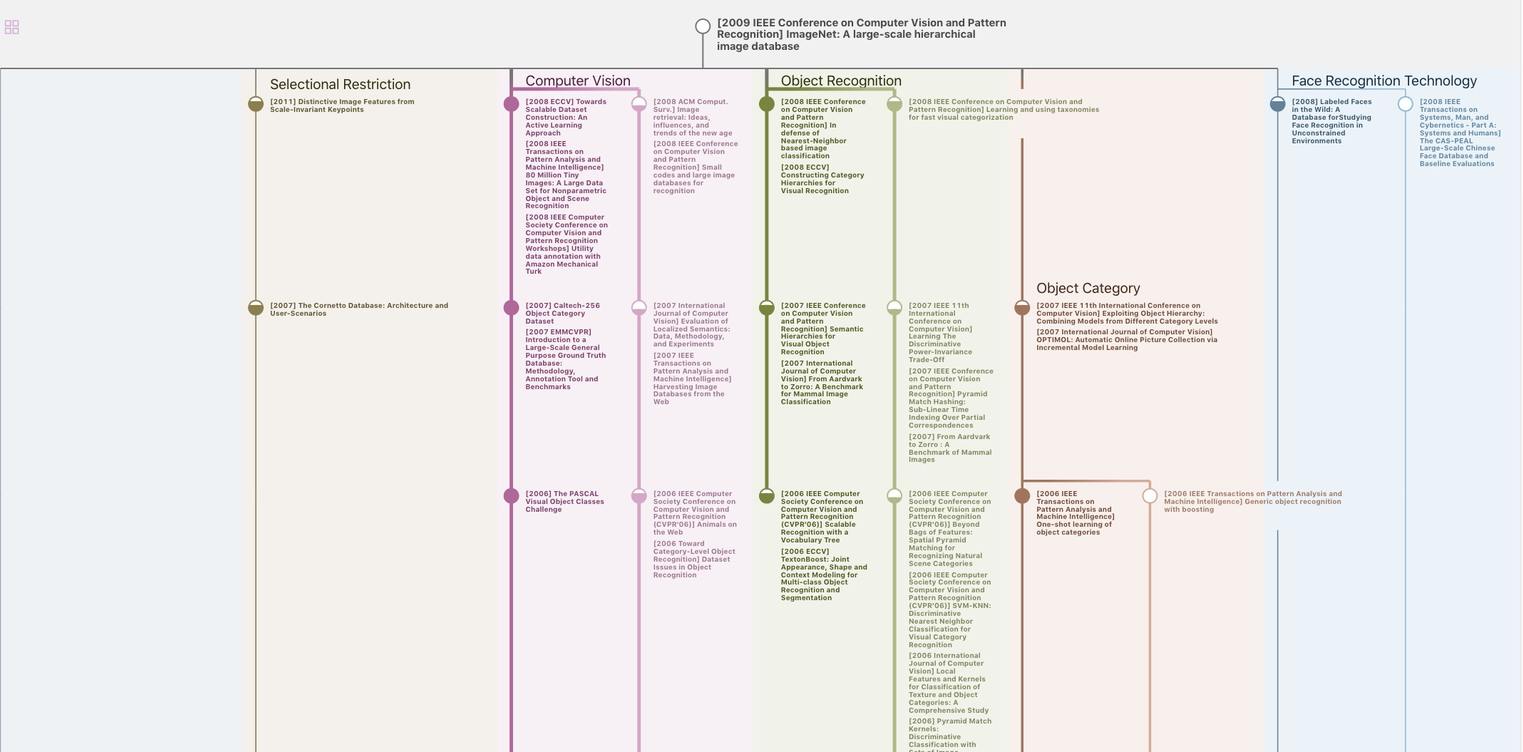
生成溯源树,研究论文发展脉络
Chat Paper
正在生成论文摘要