Lessons learned from the student dropout patterns on COVID-19 pandemic: An analysis supported by machine learning
BRITISH JOURNAL OF EDUCATIONAL TECHNOLOGY(2024)
摘要
During the COVID-19 pandemic, the challenges associated with the transition from face-to-face to emergency remote education increased concerns about student dropout. Aligned with this concern, this study investigates the impact of the pandemic on the dropout patterns of 3371 undergraduate students from a Brazilian institution. Using data mining and machine learning techniques, we developed predictive dropout models based on student data preceding and succeeding the onset of the pandemic. Through the interpretation and comparison of these models and with the support of statistical and graphical analyses, we identify that the patterns persistently indicate that young students in their initial semesters, characterized by lower income, academic performance, and interaction, remain most susceptible to dropping out. Despite the pandemic leading to an enhanced predictive capability of data regarding student interaction within the virtual learning environment, our analysis revealed a lack of significant variation in dropout patterns. Institutionally, this indicates that a considerable number of dropouts likely encountered challenges in adapting to higher education, both before and throughout the pandemic.
更多查看译文
关键词
COVID-19,educational data mining,machine learning,student dropout
AI 理解论文
溯源树
样例
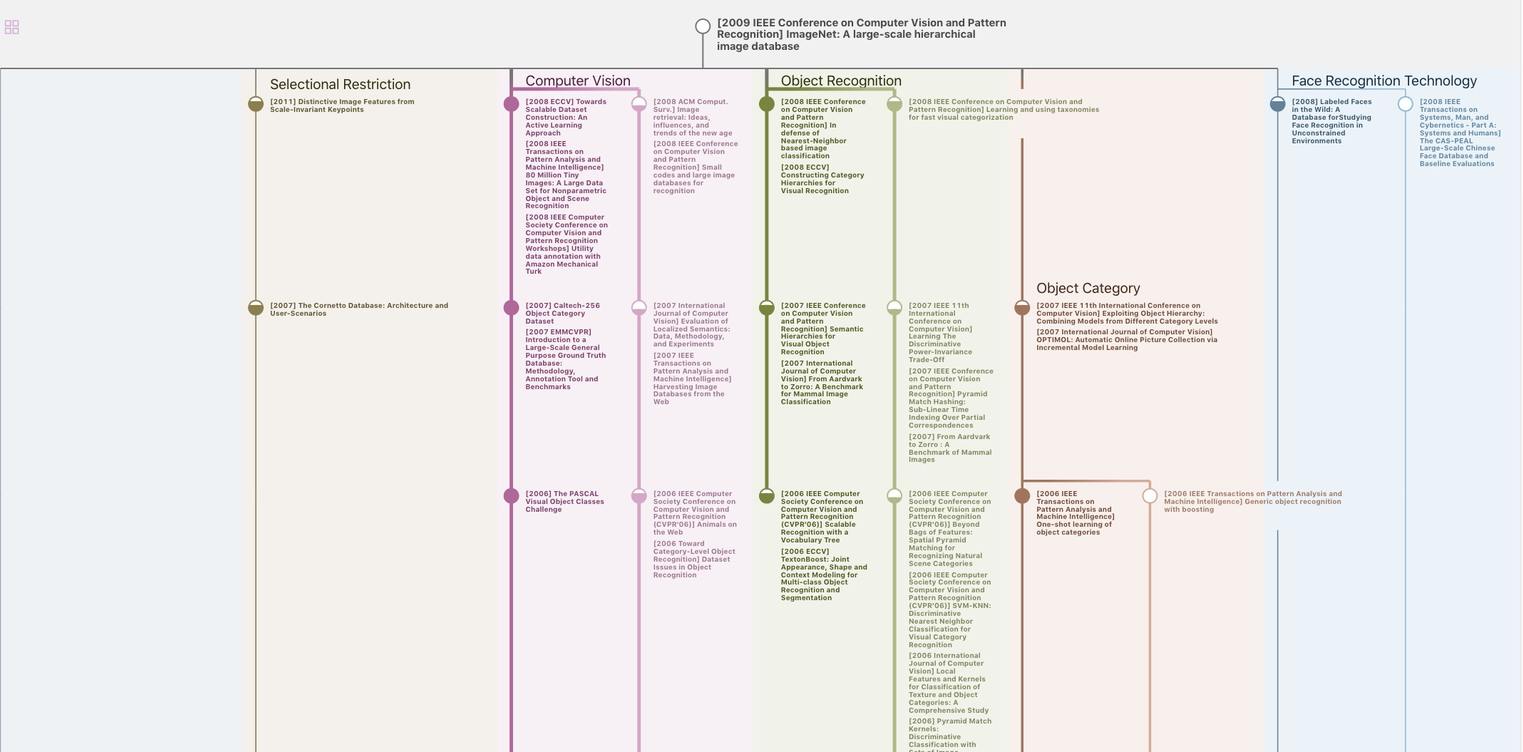
生成溯源树,研究论文发展脉络
Chat Paper
正在生成论文摘要