Motion Planning for Autonomous Vehicles in Unanticipated Obstacle Scenarios at Intersections Based on Artificial Potential Field
APPLIED SCIENCES-BASEL(2024)
摘要
Featured Application This work designed a motion planning algorithm for autonomous vehicles in unanticipated obstacle scenarios. In standard driving scenarios, the proposed motion planning algorithm plans a trajectory that complies with intersection regulations, including lane-marking, recommended turning lane, traffic light, right-of-way, and no-parking rules. In unanticipated obstacle scenarios, after the necessity of obstacle avoidance is identified, the ego vehicle would break the rules temporarily to ensure the safety and mobility of autonomous vehicles.Abstract In unanticipated obstacle scenarios at intersections, the safety and mobility of autonomous vehicles (AVs) are negatively impacted due to the conflict between traffic law compliance and obstacle avoidance. To solve this problem, an obstacle avoidance motion planning algorithm based on artificial potential field (APF) is proposed. An APF-switching logic is utilized to design the motion planning framework. Collision risk and travel delay are quantified as the switching triggers. The intersection traffic laws are digitalized and classified to construct compliance-oriented potential fields. A potential violation cost index (PVCI) is designed according to theories of autonomous driving ethics. The compliance-oriented potential fields are reconfigured according to the PVCI, forming violation cost potential fields. A cost function is designed based on compliance-oriented and violation cost potential fields, integrated with model predictive control (MPC) for trajectory optimization and tracking. The effectiveness of the proposed algorithm is verified through simulation experiments comparing diverse traffic law constraint strategies. The results indicate that the algorithm can help AVs avoid obstacles safely in unanticipated obstacle scenarios at intersections.
更多查看译文
关键词
autonomous vehicles,motion planning,traffic law,violation cost,artificial potential field,model predictive control
AI 理解论文
溯源树
样例
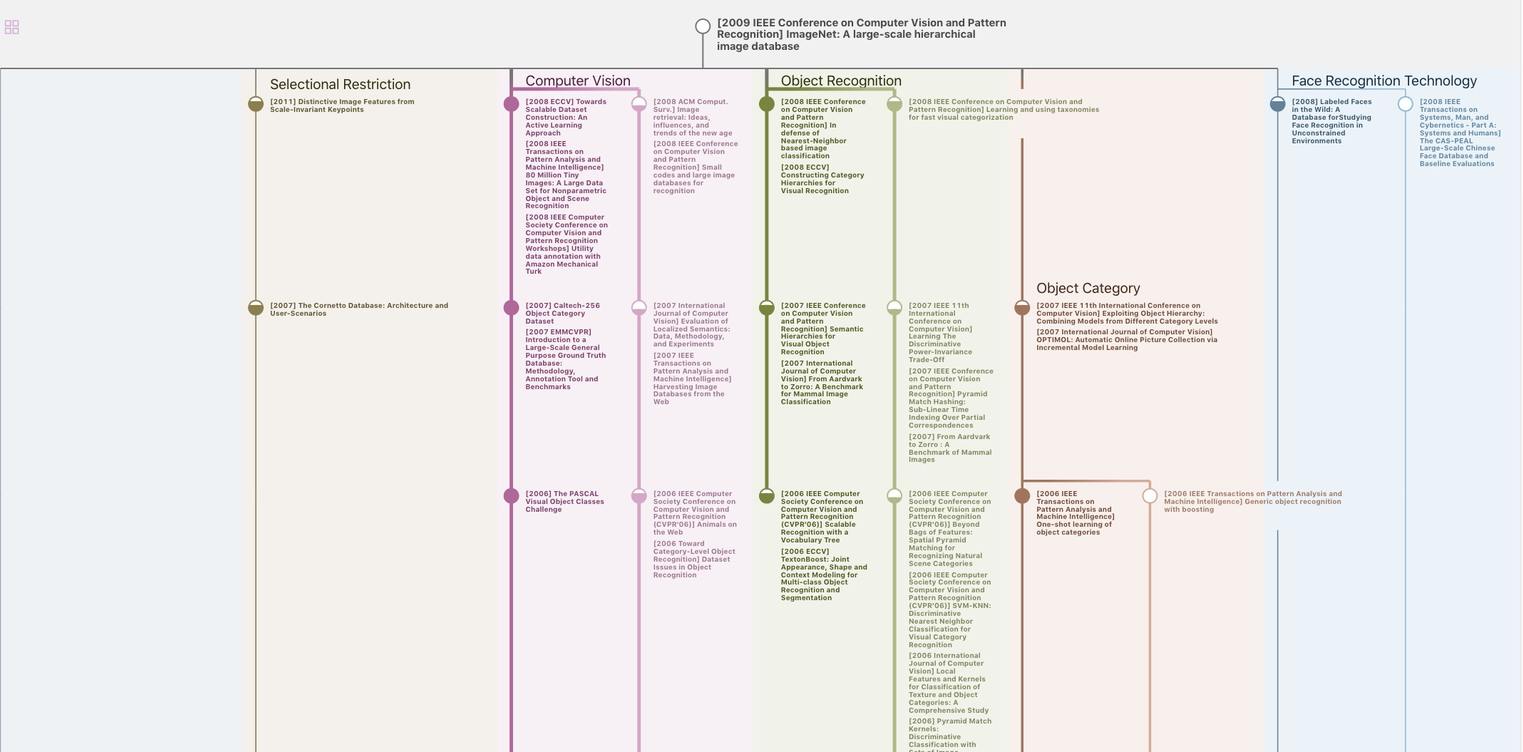
生成溯源树,研究论文发展脉络
Chat Paper
正在生成论文摘要