Vehicle Trajectory Reconstruction Using Lagrange-Interpolation-Based Framework
APPLIED SCIENCES-BASEL(2024)
摘要
Featured Application This research contributes to the development of a technological method to obtain highly accurate vehicle trajectory data. The reconstructed trajectory data play a key role in traffic state prediction, traffic management and the decision making of autonomous vehicles and robots.Abstract Vehicle trajectory usually suffers from a large number of outliers and observation noises. This paper proposes a novel framework for reconstructing vehicle trajectories. The framework integrates the wavelet transform, Lagrange interpolation and Kalman filtering. The wavelet transform based on waveform decomposition in the time and frequency domain is used to identify the abnormal frequency of a trajectory. Lagrange interpolation is used to estimate the value of data points after outliers are removed. This framework improves computation efficiency in data segmentation. The Kalman filter uses normal and predicted data to obtain reasonable results, and the algorithm makes an optimal estimation that has a better denoising effect. The proposed framework is compared with a baseline framework on the trajectory data in the NGSIM dataset. The experimental results showed that the proposed framework can achieve a 45.76% lower root mean square error, 26.43% higher signal-to-noise ratio and 25.58% higher Pearson correlation coefficient.
更多查看译文
关键词
vehicle trajectory reconstruction,outlier detection,Lagrange interpolation,filter denoising,NGSIM
AI 理解论文
溯源树
样例
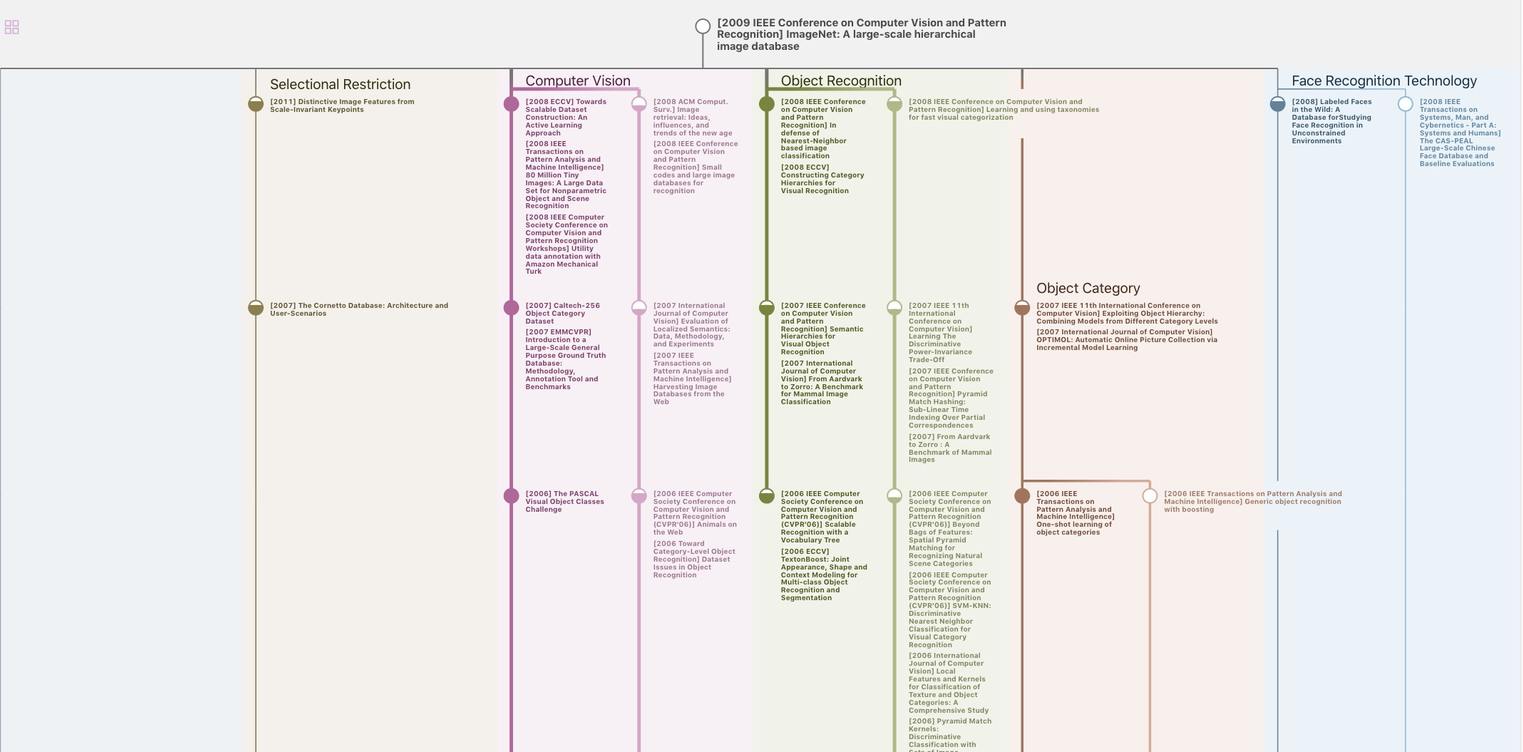
生成溯源树,研究论文发展脉络
Chat Paper
正在生成论文摘要