Reduced-order modeling of two-dimensional turbulent Rayleigh-Bnard flow by hybrid quantum-classical reservoir computing
PHYSICAL REVIEW RESEARCH(2023)
摘要
Two hybrid quantum-classical reservoir computing models are presented to reproduce the low-order statistical properties of a two-dimensional turbulent Rayleigh-Benard convection flow at a Rayleigh number Ra = 105 and Prandtl number Pr = 10. These properties comprise the mean vertical profiles of the root mean square velocity and temperature and the turbulent convective heat flux. The latter is composed of vertical velocity and temperature and measures the global turbulent heat transfer across the convection layer; it manifests locally in coherent hot and cold thermal plumes that rise from the bottom and fall from the top boundaries. Both quantum algorithms differ by the arrangement of the circuit layers of the quantum reservoir, in particular the entanglement layers. The second of the two quantum circuit architectures, denoted H2, enables a complete execution of the reservoir update inside the quantum circuit without the usage of external memory. Their performance is compared with that of a classical reservoir computing model. Therefore, all three models have to learn the nonlinear and chaotic dynamics of the turbulent flow at hand in a lower-dimensional latent data space which is spanned by the time-dependent expansion coefficients of the 16 most energetic proper orthogonal decomposition (POD) modes. These training data are generated by a POD snapshot analysis from direct numerical simulations of the original turbulent flow. All reservoir computing models are operated in the reconstruction or open-loop mode, i.e., they receive three POD modes as an input at each step and reconstruct the 13 missing modes. We analyze different measures of the reconstruction error in dependence on the hyperparameters which are specific for the quantum cases or shared with the classical counterpart, such as the reservoir size and the leaking rate. We show that both quantum algorithms are able to reconstruct the essential statistical properties of the turbulent convection flow successfully with similar performance compared with the classical reservoir network. Most importantly, the quantum reservoirs are by a factor of four to eight smaller in comparison with the classical case.
更多查看译文
AI 理解论文
溯源树
样例
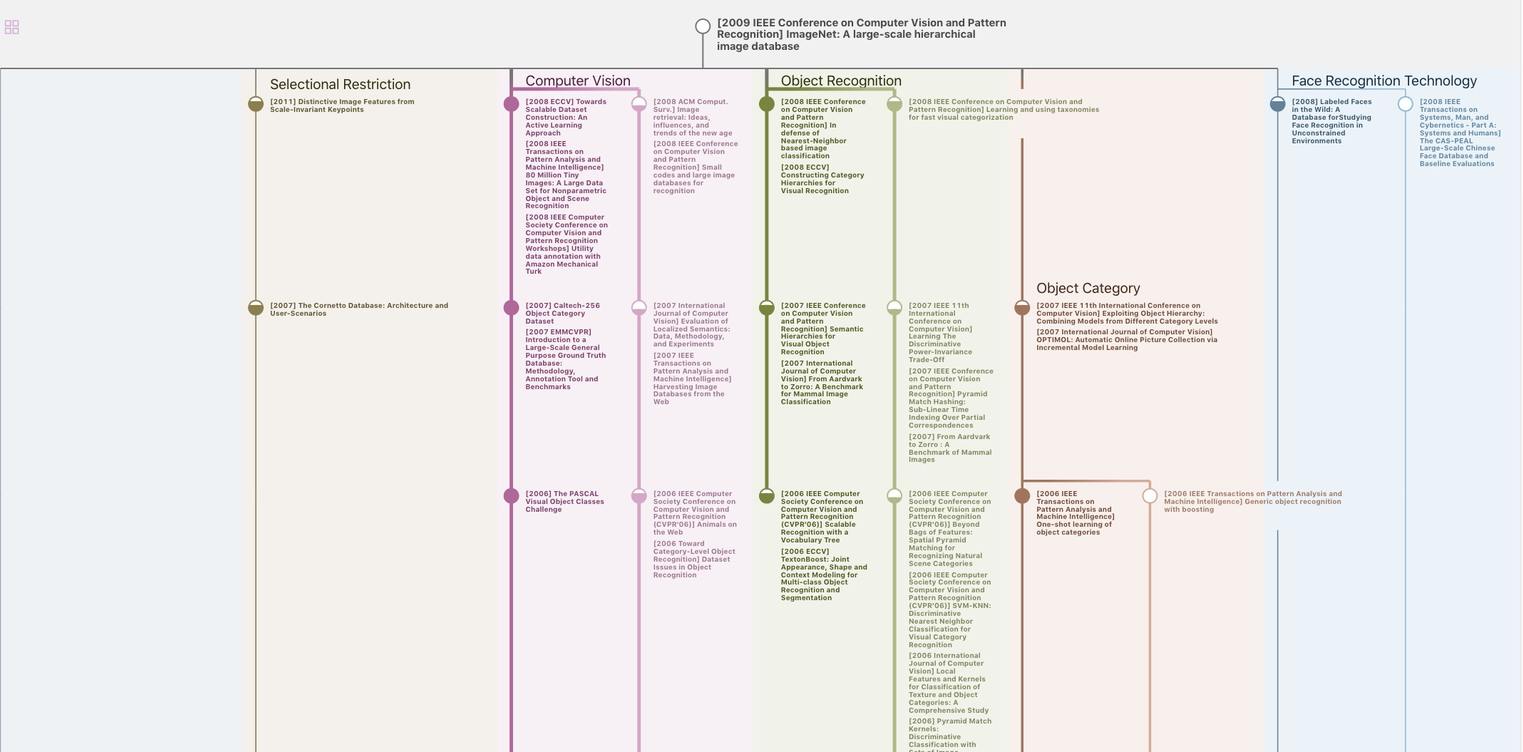
生成溯源树,研究论文发展脉络
Chat Paper
正在生成论文摘要