Improving Product Search with Season-Aware Qery-Product Semantic Similarity
COMPANION OF THE WORLD WIDE WEB CONFERENCE, WWW 2023(2023)
摘要
Product search for online shopping should be season-aware, i.e., presenting seasonally relevant products to customers. In this paper, we propose a simple yet effective solution to improve seasonal relevance in product search by incorporating seasonality into language models for semantic matching. We first identify seasonal queries and products by analyzing implicit seasonal contexts through time-series analysis over the past year. Then we introduce explicit seasonal contexts by enhancing the query representation with a season token according to when the query is issued. A new season-enhanced BERT model (SE-BERT) is also proposed to learn the semantic similarity between the resulting seasonal queries and products. SE-BERT utilizes Multi-modal Adaption Gate (MAG) to augment the season-enhanced semantic embedding with other contextual information such as product price and review counts for robust relevance prediction. To better align with the ranking objective, a listwise loss function (neural NDCG) is used to regularize learning. Experimental results validate the effectiveness of the proposed method, which outperforms existing solutions for query-product relevance prediction in terms of NDCG and Price Weighted Purchases (PWP).
更多查看译文
关键词
seasonal relevance,product search,language model
AI 理解论文
溯源树
样例
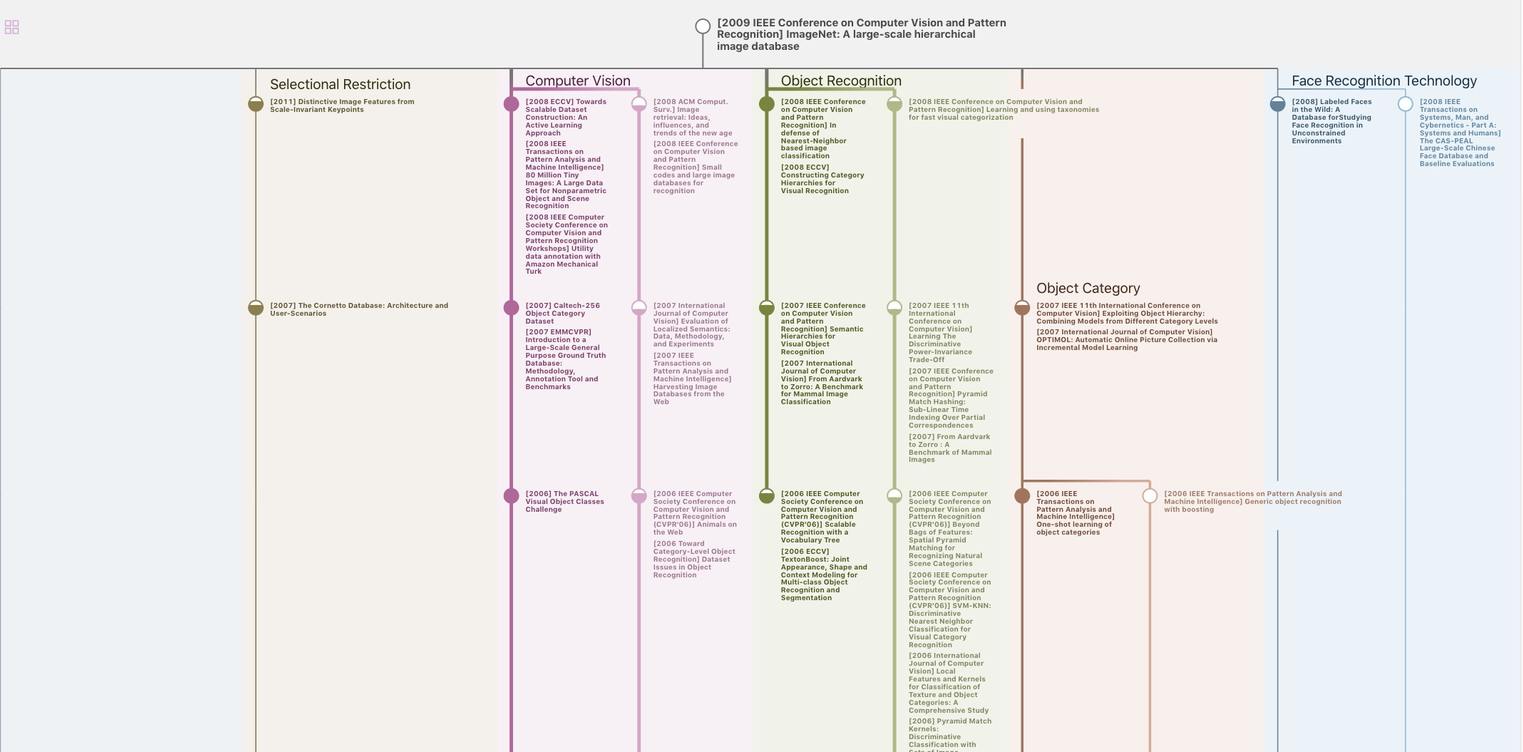
生成溯源树,研究论文发展脉络
Chat Paper
正在生成论文摘要