Predicting Imminent Cyanobacterial Blooms in Lakes Using Incomplete Timely Data
WATER RESOURCES RESEARCH(2024)
摘要
Toxic cyanobacterial blooms (CBs) are becoming more frequent globally, posing a threat to freshwater ecosystems. While making long-range forecasts is overly challenging, predicting imminent CBs is possible from precise monitoring data of the underlying covariates. It is, however, infeasibly costly to conduct precise monitoring on a large scale, leaving most lakes unmonitored or only partially monitored. The challenge is hence to build a predictive model that can use the incomplete, partially-monitored data to make near-future CB predictions. By using 30 years of monitoring data for 78 water bodies in Alberta, Canada, combined with data of watershed characteristics (including natural land cover and anthropogenic land use) and meteorological conditions, we train a Bayesian network that predicts future 2-week CB with an area under the curve (AUC) of 0.83. The only monitoring data that the model needs to reach this level of accuracy are whether the cell count and Secchi depth are low, medium, or high, which can be estimated by advanced high-resolution imaging technology or trained local citizens. The model is robust against missing values as in the absence of any single covariate, it performs with an AUC of at least 0.78. While taking a major step toward reduced-cost, less data-intensive CB forecasting, our results identify those key covariates that are worth the monitoring investment for highly accurate predictions.
更多查看译文
关键词
cyanobacterial bloom,Bayesian network,predictive ecology,machine learning,environmental covariates
AI 理解论文
溯源树
样例
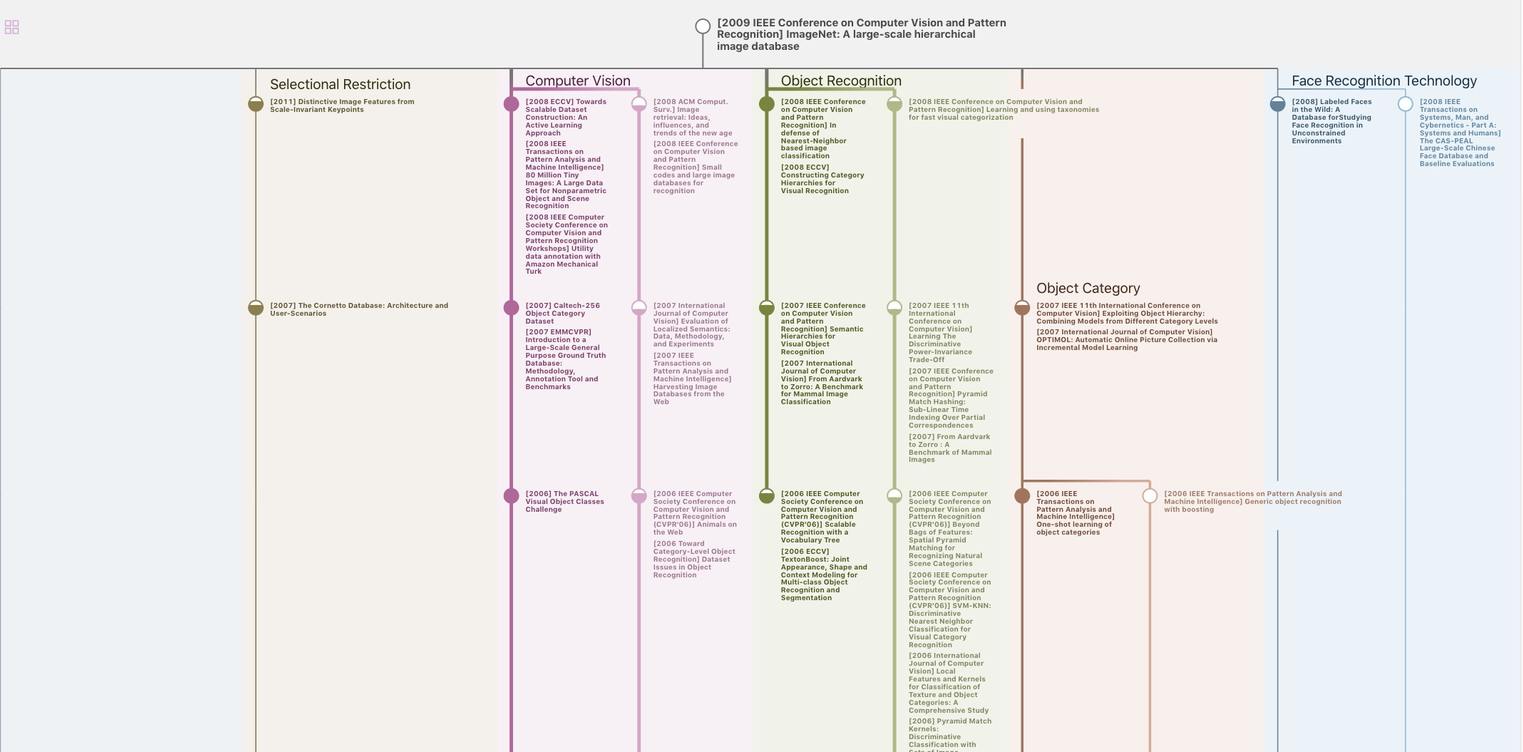
生成溯源树,研究论文发展脉络
Chat Paper
正在生成论文摘要