Hierarchical Graph Contrastive Learning
MACHINE LEARNING AND KNOWLEDGE DISCOVERY IN DATABASES: RESEARCH TRACK, ECML PKDD 2023, PT II(2023)
摘要
Unsupervised graph representation learning with GNNs is critically important due to the difficulty of obtaining graph labels in many real applications. Graph contrastive learning (GCL), a recently popular method for unsupervised learning on graphs, has achieved great success on many tasks. However, existing graph-level GCL models generally focus on comparing the graph-level representation or node-level representation. The hierarchical structure property, which is ubiquitous in many real world graphs such as social networks and molecular graphs, is largely ignored. To bridge this gap, this paper proposes a novel hierarchical graph contrastive learning model named HIGCL. HIGCL uses a multi-layered architecture and contains two contrastive objectives, inner-contrasting and hierarchical-contrasting. The former conducts inner-scale contrastive learning to learn the flat structural features in each layer, while the latter focuses on performing cross-scale contrastive learning to capture the hierarchical features across layers. Extensive experiments are conducted on graph-level tasks to show the effectiveness of the proposed method.
更多查看译文
关键词
Graph Contrastive Learning,Graph Neural Network,Unsupervised Learning
AI 理解论文
溯源树
样例
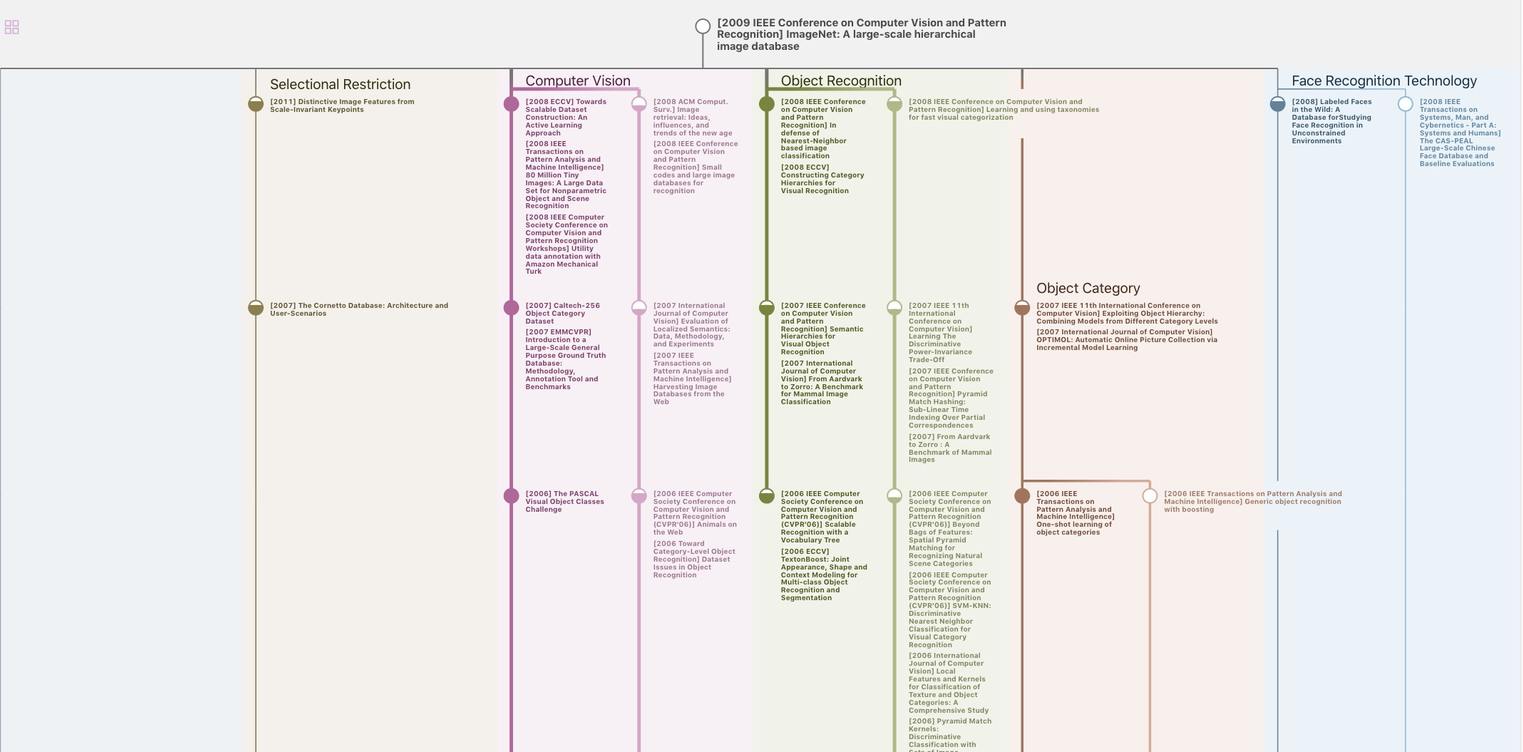
生成溯源树,研究论文发展脉络
Chat Paper
正在生成论文摘要