Incremental Quasi-Newton Methods with Faster Superlinear Convergence Rates
AAAI 2024(2024)
摘要
We consider the finite-sum optimization problem, where each component function is strongly convex and has Lipschitz continuous gradient and Hessian. The recently proposed incremental quasi-Newton method is based on BFGS update and achieves a local superlinear convergence rate that is dependent on the condition number of the problem. This paper proposes a more efficient quasi-Newton method by incorporating the symmetric rank-1 update into the incremental framework, which results in the condition-number-free local superlinear convergence rate. Furthermore, we can boost our method by applying the block update on the Hessian approximation, which leads to an even faster local convergence rate. The numerical experiments show the proposed methods significantly outperform the baseline methods.
更多查看译文
关键词
ML: Optimization
AI 理解论文
溯源树
样例
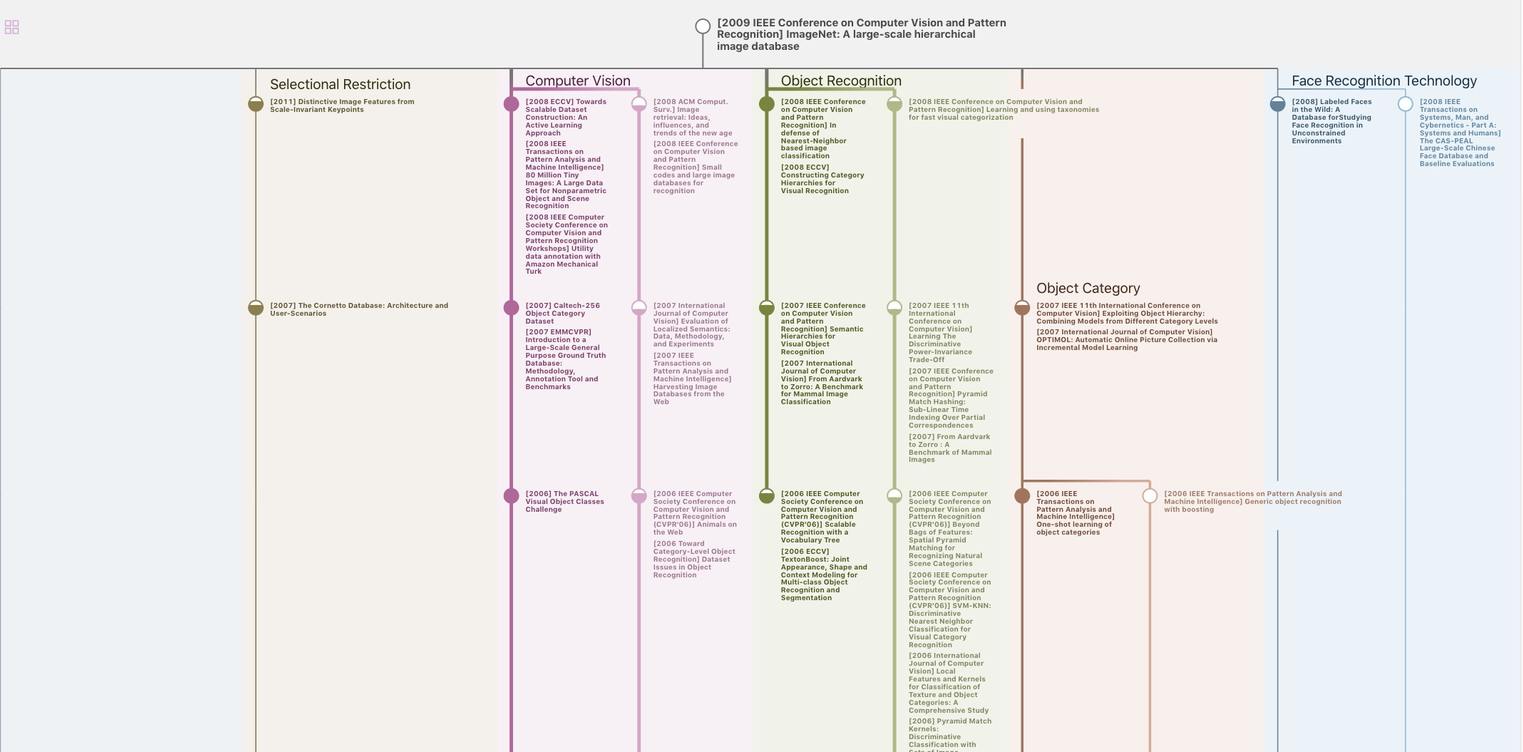
生成溯源树,研究论文发展脉络
Chat Paper
正在生成论文摘要