Multiple Time Series Forecasting with Dynamic Graph Modeling
PROCEEDINGS OF THE VLDB ENDOWMENT(2023)
摘要
Multiple time series forecasting plays an essential role in many applications. Solutions based on graph neural network (GNN) that deliver state-of-the-art forecasting performance use the relation graph which can capture historical correlations among time series. However, in real world, it is common that correlations among time series evolve across time, resulting in dynamic relation graph, where the future correlations may be different from those in history. To address this problem, we propose multiple time series forecasting with dynamic graph modeling (MTSF-DG) that is able to learn historical relation graphs and predicting future relation graphs to capture the dynamic correlations. We also propose a causal GNN to extract features from both kinds of relation graphs efficiently. Then we propose a reasoning network to explicitly learn the variant influence from historical timestamps to future timestamps for final forecasting. Extensive experiments on six benchmark datasets show that MTSF-DG consistently outperforms state-of-the-art baselines, and justify our design with dynamic relation graph modeling.
更多查看译文
AI 理解论文
溯源树
样例
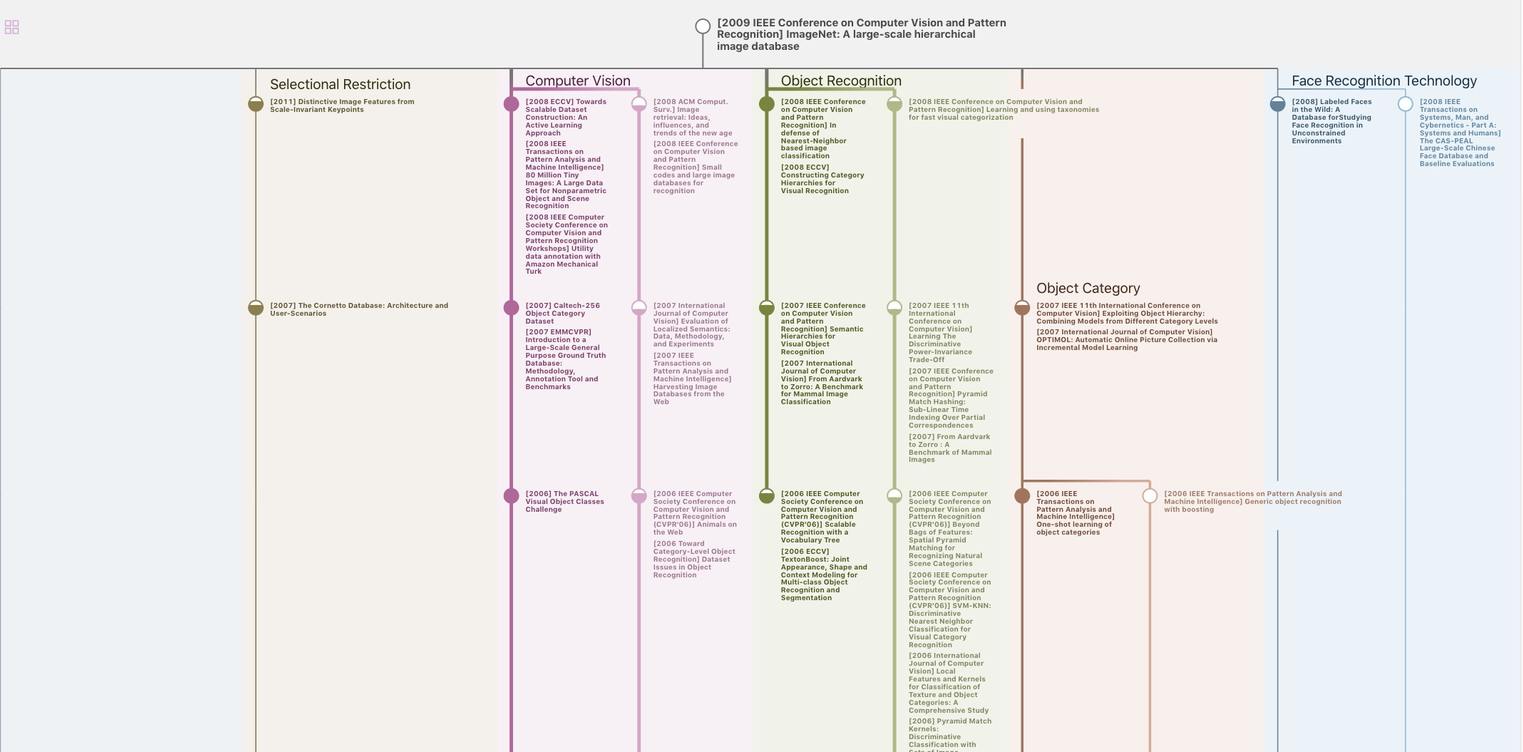
生成溯源树,研究论文发展脉络
Chat Paper
正在生成论文摘要