Convolutional Neural Network Assisted Transformer for Automatic Modulation Recognition Under Large CFOs and SROs
IEEE SIGNAL PROCESSING LETTERS(2024)
摘要
Automatic modulation recognition (AMR) has received widespread attention as a crucial aspect of non-cooperative communication. Despite this, large carrier frequency offsets (CFOs) and sample rate offsets (SROs) caused by inaccurate parameter estimation at the receiver are harmful to the recognition accuracy, which is still to be addressed. In this letter, we focus on intelligent modulation recognition tasks under such offsets. A novel transformer-based method named TransGroupNet is designed that can extract deep features of signals from the instantaneous amplitude, phase, and frequency (APF) domain. In addition, lightweight group convolution layers are added ahead of the transformer blocks for better feature preprocessing. Simulations demonstrate that the proposed TransGroupNet achieves better recognition accuracy under large offsets compared with the previous state-of-the-art methods, even though these methods adopt correction modules (CM) to address such offsets.
更多查看译文
关键词
Transformers,Convolution,Modulation,Feature extraction,Task analysis,Receivers,Frequency modulation,Automatic modulation recognition,carrier frequency offsets,sample rate offsets,transformer,group convolution
AI 理解论文
溯源树
样例
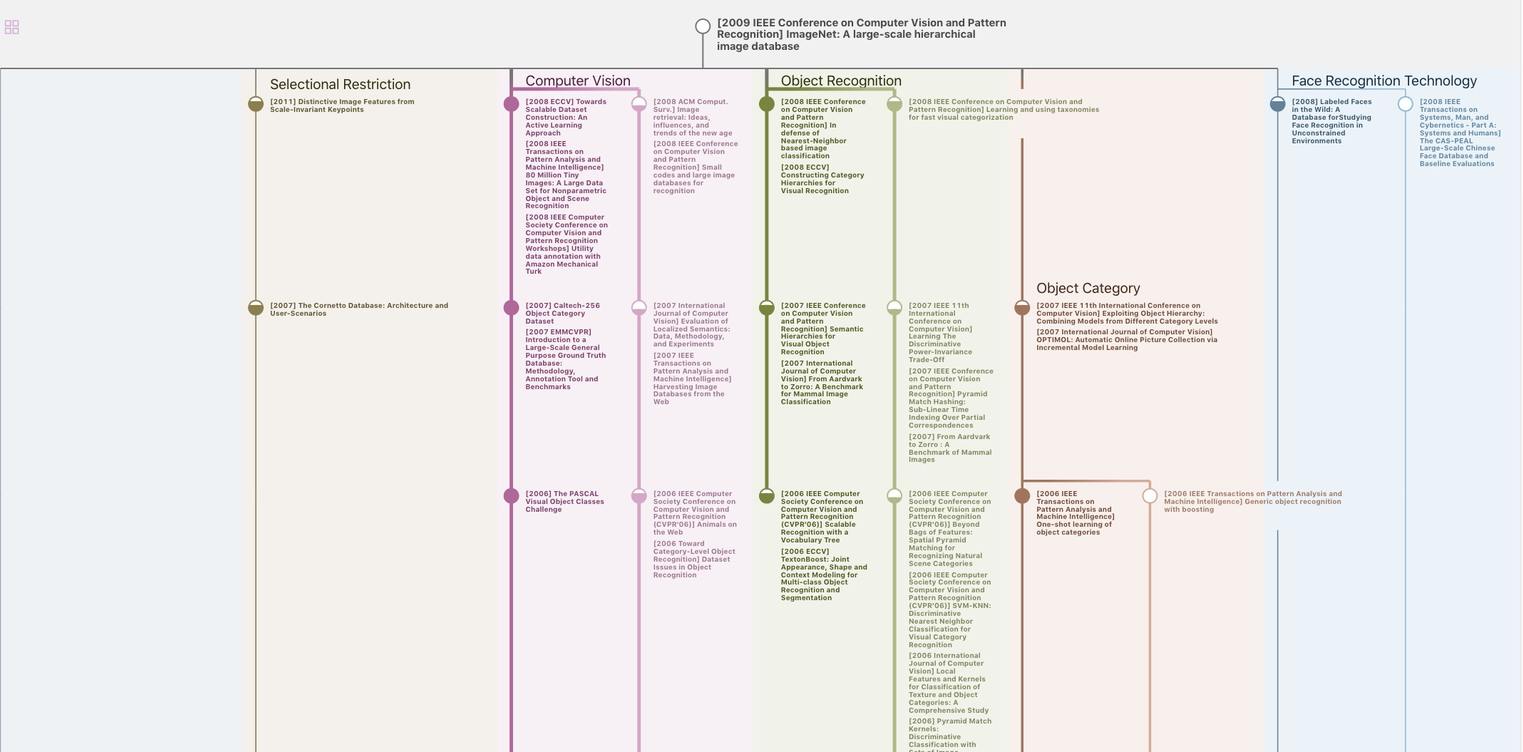
生成溯源树,研究论文发展脉络
Chat Paper
正在生成论文摘要