A Heart Disease Prognosis Pipeline for the Edge using Federated Learning
e-Prime - Advances in Electrical Engineering, Electronics and Energy(2024)
摘要
Cloud computing and edge computing have revolutionized deployments by giving virtually unlimited computing and storage to ensure the scalability and availability of applications. This paper explores an application that can be used as a decision support system for heart disease prognosis. It discusses deployment strategies on a cloud-native model and an edge-optimized model. The application contains a customized prediction pipeline named ClassifyIT with a custom neural network architecture called IPANN, supported by a feature selector named MIST-CC and a regularizer named STIR. ClassifyIT was observed to give an accuracy of 87.16% on the Cleveland dataset, compared to 78.80% for a regular deep network. The addition of the MIST-CC feature selection algorithm to the deep network was shown to improve its accuracy to 81.97%, and it is further enhanced to 85.54% by adding STIR. This pipeline is then deployed on an application based on a cloud-native architecture that uses microservices. The design is expanded to an edge-optimized architecture that improves scalability by moving part of the computation to the user device. The machine learning pipeline is further enhanced using federated learning to improve localization and collaborative learning. Both architectures are compared in a subjective fashion based on various parameters.
更多查看译文
关键词
Cloud computing,Machine learning,Serverless architectures,Edge computing,Heart diseases,Neural Networks,Internet of Things
AI 理解论文
溯源树
样例
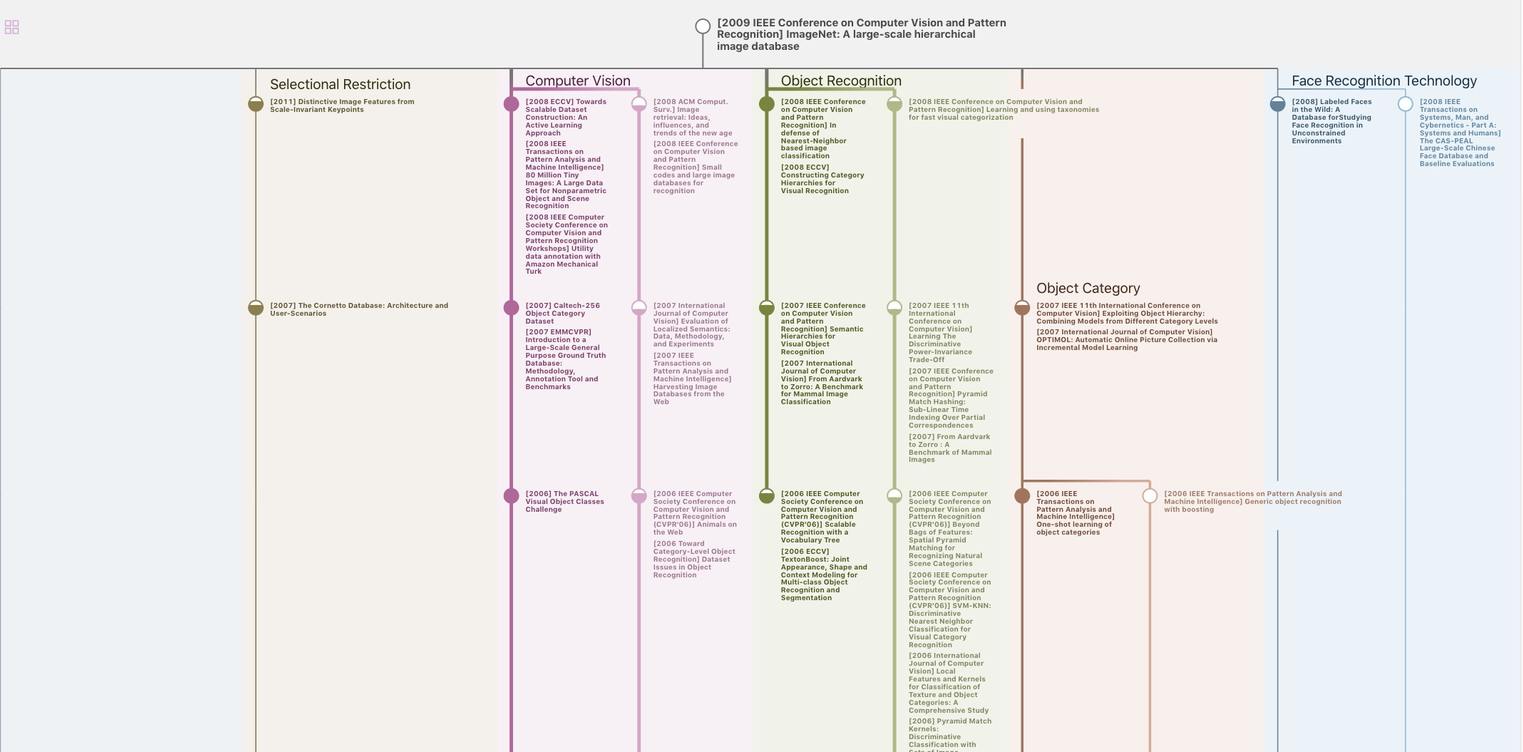
生成溯源树,研究论文发展脉络
Chat Paper
正在生成论文摘要