Machine Learning Assisted Adjustment Boosts Inferential Efficiency of Randomized Controlled Trials
arxiv(2024)
摘要
In this work, we proposed a novel inferential procedure assisted by machine
learning based adjustment for randomized control trials. The method was
developed under the Rosenbaum's framework of exact tests in randomized
experiments with covariate adjustments. Through extensive simulation
experiments, we showed the proposed method can robustly control the type I
error and can boost the inference efficiency for a randomized controlled trial
(RCT). This advantage was further demonstrated in a real world example. The
simplicity and robustness of the proposed method makes it a competitive
candidate as a routine inference procedure for RCTs, especially when the number
of baseline covariates is large, and when nonlinear association or interaction
among covariates is expected. Its application may remarkably reduce the
required sample size and cost of RCTs, such as phase III clinical trials.
更多查看译文
AI 理解论文
溯源树
样例
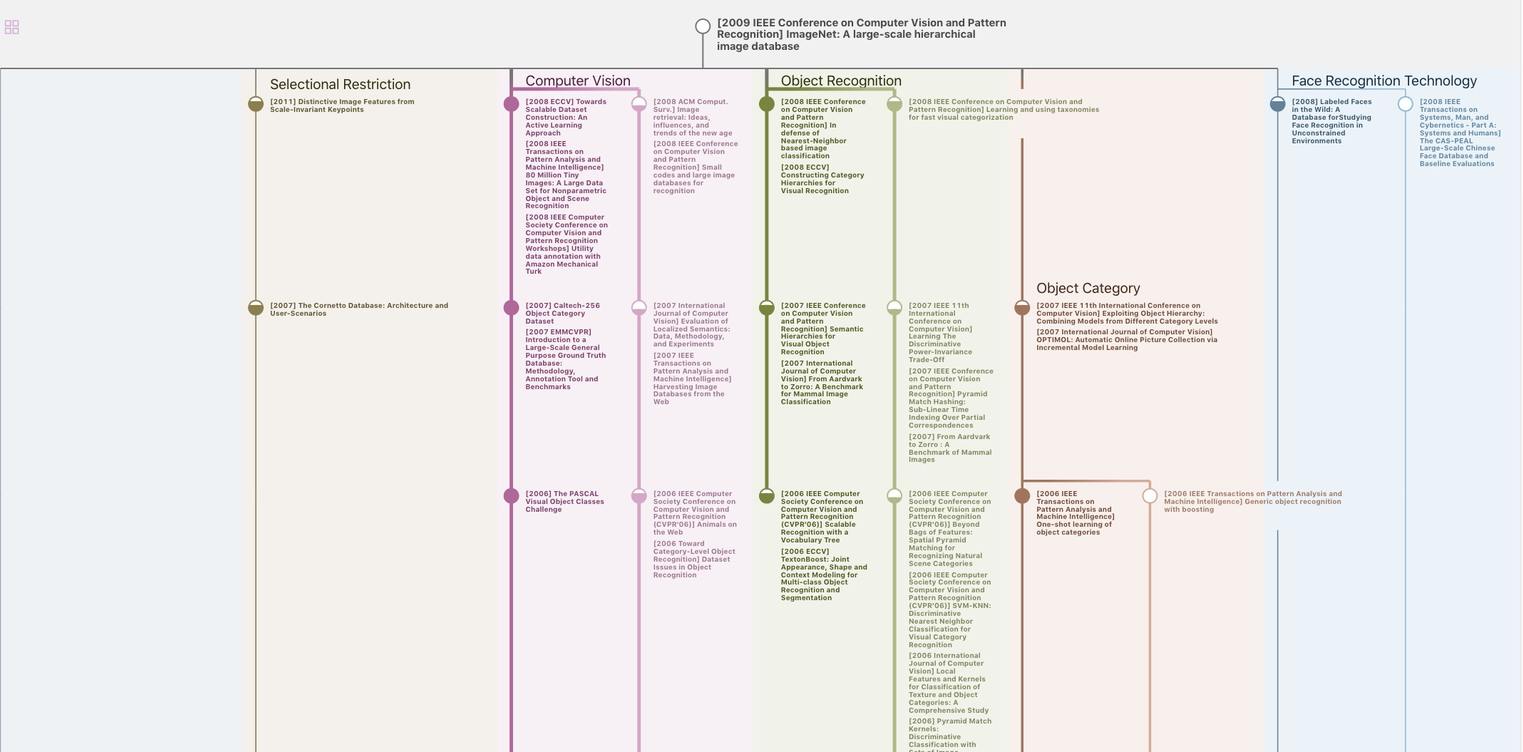
生成溯源树,研究论文发展脉络
Chat Paper
正在生成论文摘要