Spintronic Implementation of UNet for Image Segmentation
CoRR(2024)
摘要
Image segmentation plays a crucial role in computer vision applications like
self-driving cars, satellite imagery analysis, and medical diagnosis.
Implementing these complex deep neural networks on conventional hardware is
highly inefficient. In this work, we propose hardware implementation of UNet
for segmentation tasks, using spintronic devices. Our approach involves
designing hardware for convolution, deconvolution, ReLU, and max pooling layers
of the UNet architecture. We demonstrate the synaptic behavior of the domain
wall MTJ, and design convolution and deconvolution layers using the domain
wall-based crossbar array. We utilize the orthogonal current injected MTJ with
its continuous resistance change and showcase the ReLU and max pooling
functions. We employ a hybrid simulation setup by coupling micromagnetic
simulation, non-equilibrium Green's function,
Landau-Lifshitz-Gilbert-Slonczewski equations, and circuit simulation with
Python programming to incorporate the diverse physics of spin-transport,
magnetization dynamics, and CMOS elements in our proposed designs. We evaluate
our UNet design on the CamVid dataset and achieve segmentation accuracies that
are comparable to software implementation. During training, our design consumes
43.59pJ of energy for synaptic weight updates.
更多查看译文
AI 理解论文
溯源树
样例
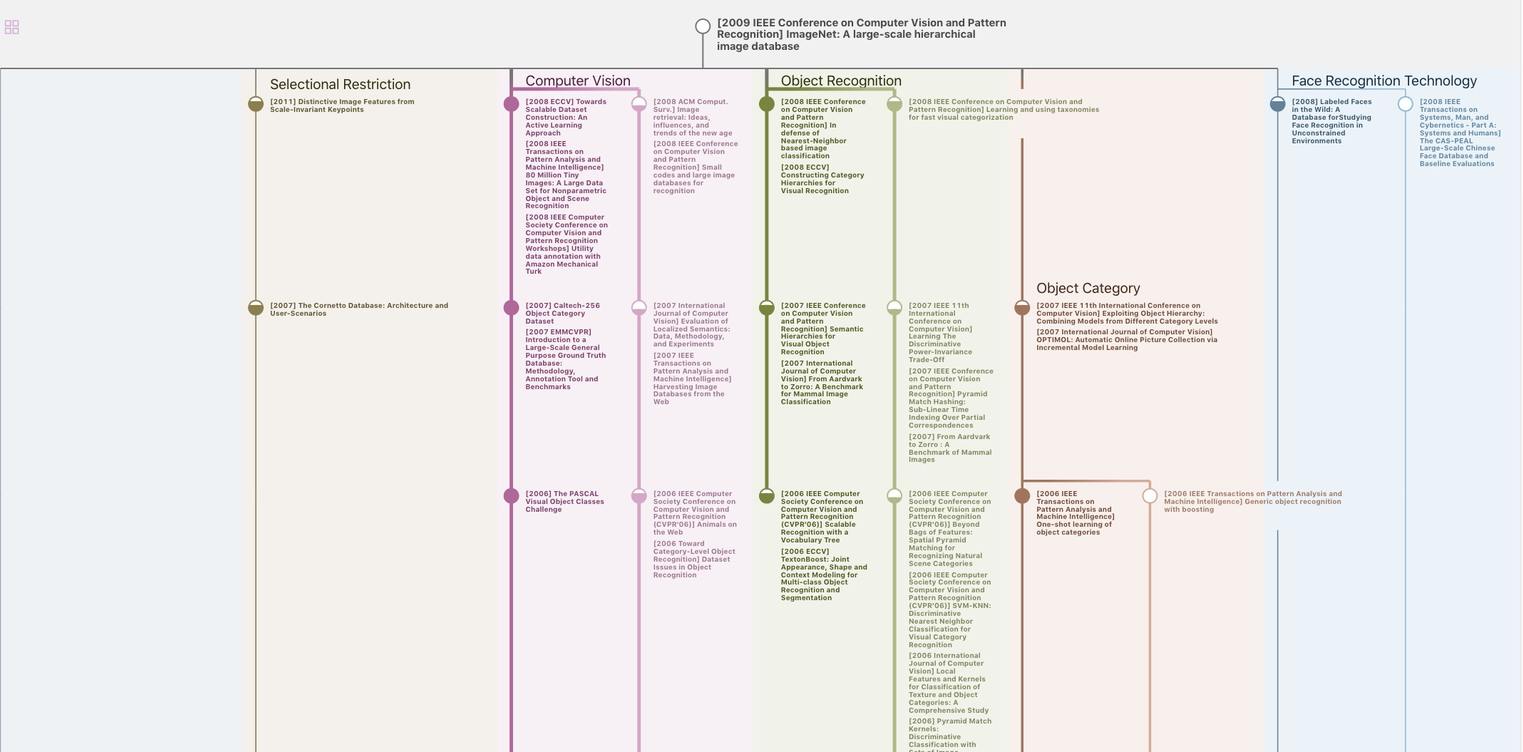
生成溯源树,研究论文发展脉络
Chat Paper
正在生成论文摘要