Low-rank Tensor Autoregressive Predictor for Third-Order Time-Series Forecasting
arxiv(2024)
摘要
Recently, tensor time-series forecasting has gained increasing attention,
whose core requirement is how to perform dimensionality reduction. Among all
multidimensional data, third-order tensor is the most prevalent structure in
real-world scenarios, such as RGB images and network traffic data. Previous
studies in this field are mainly based on tensor Tucker decomposition and such
methods have limitations in terms of computational cost, with iteration
complexity of approximately O(2n^3r), where n and r are the dimension and
rank of original tensor data. Moreover, many real-world data does not exhibit
the low-rank property under Tucker decomposition, which may fail the
dimensionality reduction. In this paper, we pioneer the application of tensor
singular value decomposition (t-SVD) to third-order time-series, which builds
an efficient forecasting algorithm, called Low-rank Tensor Autoregressive
Predictor (LOTAP). We observe that tensor tubal rank in t-SVD is always less
than Tucker rank, which leads to great benefit in computational complexity. By
combining it with the autoregressive (AR) model, the forecasting problem is
formulated as a least squares optimization. We divide such an optimization
problem by fast Fourier transformation into four decoupled subproblems, whose
variables include regressive coefficient, f-diagonal tensor, left and right
orthogonal tensors. The alternating minimization algorithm is proposed with
iteration complexity of about O(n^3 + n^2r^2), in which each subproblem has a
closed-form solution. Numerical experiments show that, compared to
Tucker-decomposition-based algorithms, LOTAP achieves a speed improvement
ranging from 2 to 6 times while maintaining accurate forecasting performance in
all four baseline tasks. In addition, LOTAP is applicable to a wider range of
tensor forecasting tasks due to its more effective dimensionality reduction
ability.
更多查看译文
AI 理解论文
溯源树
样例
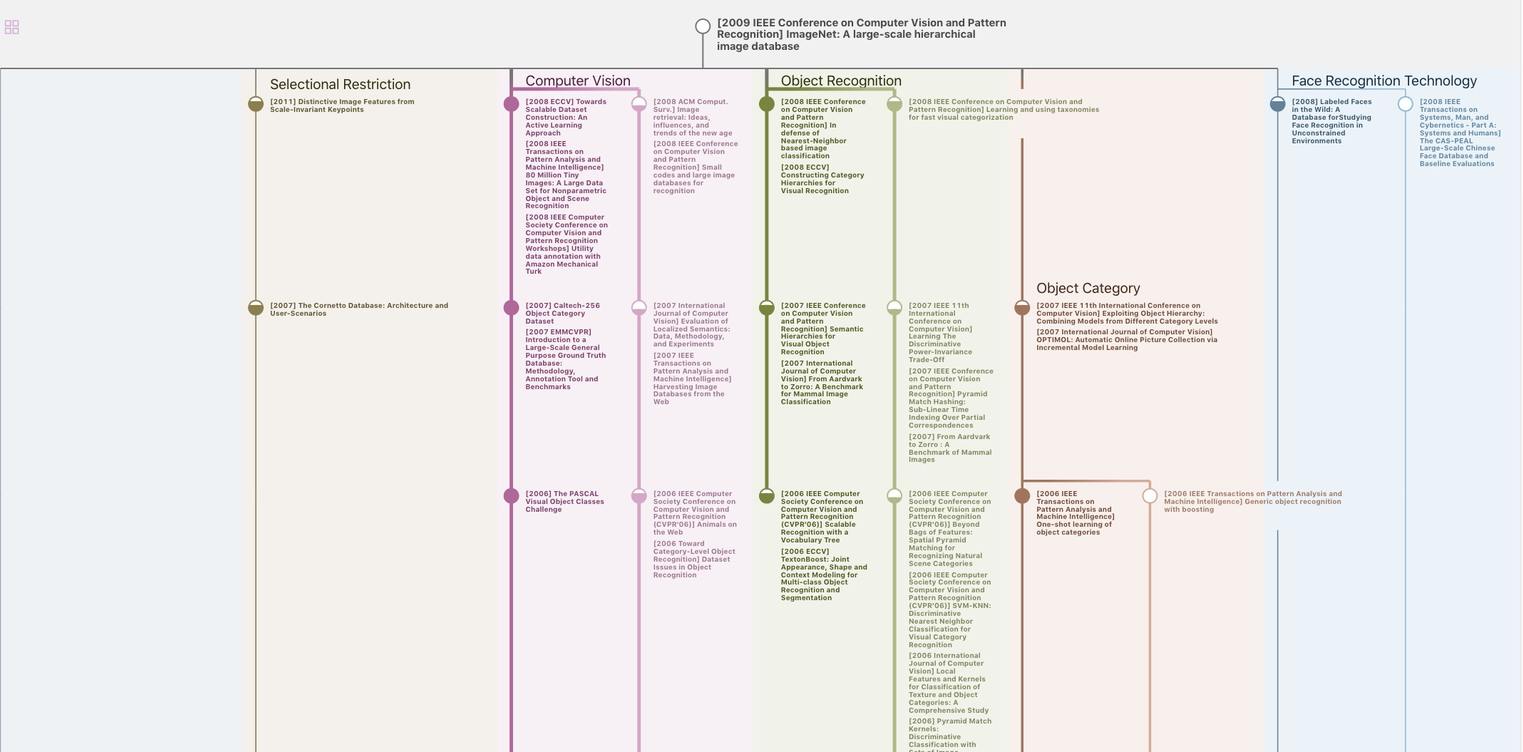
生成溯源树,研究论文发展脉络
Chat Paper
正在生成论文摘要