Progressive Smoothing for Motion Planning in Real-Time NMPC
arxiv(2024)
摘要
Nonlinear model predictive control (NMPC) is a popular strategy for solving
motion planning problems, including obstacle avoidance constraints, in
autonomous driving applications. Non-smooth obstacle shapes, such as
rectangles, introduce additional local minima in the underlying optimization
problem. Smooth over-approximations, e.g., ellipsoidal shapes, limit the
performance due to their conservativeness. We propose to vary the smoothness
and the related over-approximation by a homotopy. Instead of varying the
smoothness in consecutive sequential quadratic programming iterations, we use
formulations that decrease the smooth over-approximation from the end towards
the beginning of the prediction horizon. Thus, the real-time iterations
algorithm is applicable to the proposed NMPC formulation. Different
formulations are compared in simulation experiments and shown to successfully
improve performance indicators without increasing the computation time.
更多查看译文
AI 理解论文
溯源树
样例
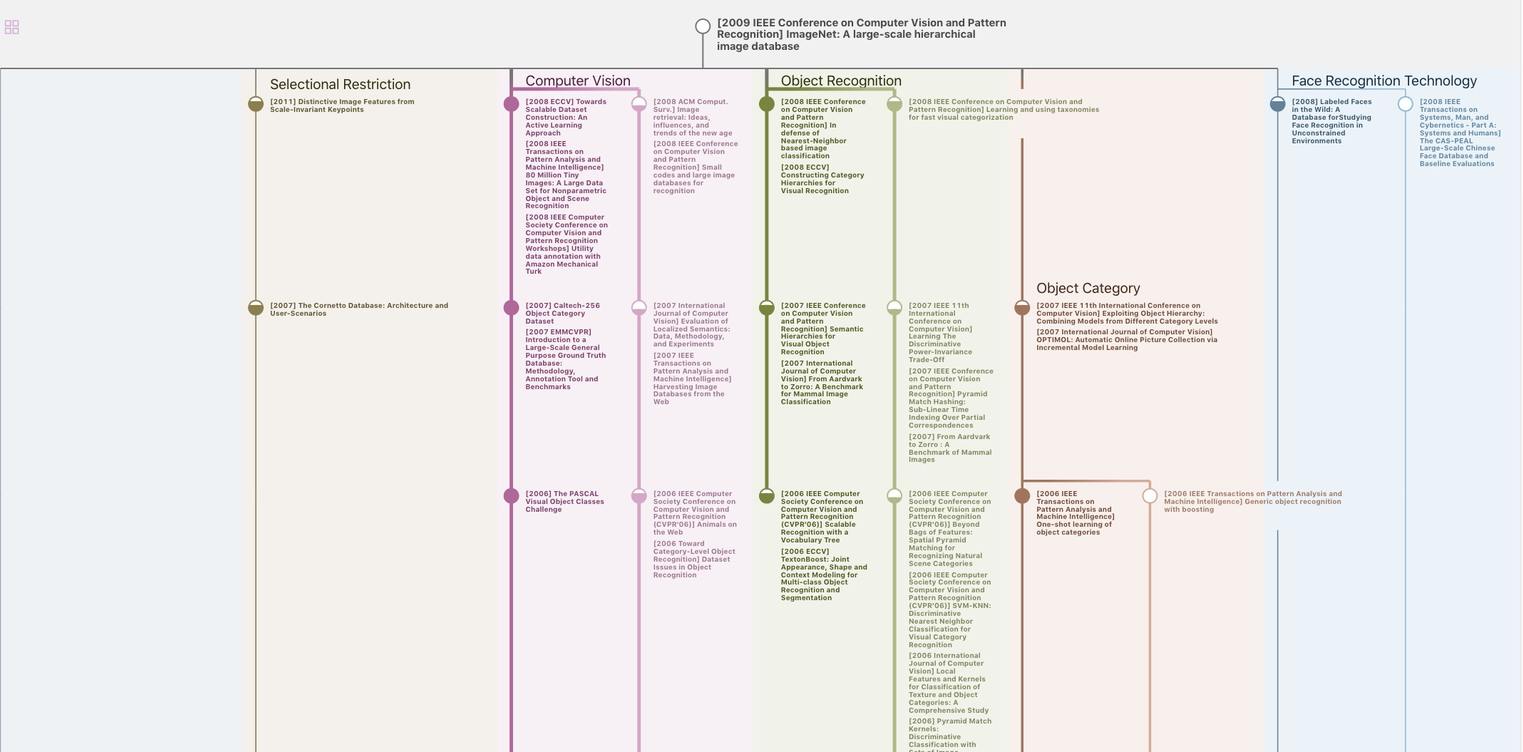
生成溯源树,研究论文发展脉络
Chat Paper
正在生成论文摘要