AFBT GAN: enhanced explainability and diagnostic performance for cognitive decline by counterfactual generative adversarial network
arxiv(2024)
摘要
Existing explanation results of functional connectivity (FC) are normally
generated by using classification result labels and correlation analysis
methods such as Pearson's correlation or gradient backward. However, the
diagnostic model is still trained on the black box model and might lack the
attention of FCs in important regions during the training. To enhance the
explainability and improve diagnostic performance, providing prior knowledge on
neurodegeneration-related regions when healthy subjects (HC) develop into
subject cognitive decline (SCD) and mild cognitive impairment (MCI) for the
diagnostic model is a key step. To better determine the
neurodegeneration-related regions, we employ counterfactual reasoning to
generate the target label FC matrices derived from source label FC and then
subtract source label FC with target label FC. The counterfactual reasoning
architecture is constructed by adaptive forward and backward transformer
generative adversarial network (AFBT GAN), which is specifically designed by
network property in FC and inverse patch embedding operation in the
transformer. The specific design can make the model focus more on the current
network correlation and employ the global insight of the transformer to
reconstruct FC, which both help the generation of high-quality target label FC.
The validation experiments are conducted on both clinical and public datasets,
the generated attention map are both vital correlated to cognitive function and
the diagnostic performance is also significant. The code is available at
https://github.com/SXR3015/AFBT-GAN.
更多查看译文
AI 理解论文
溯源树
样例
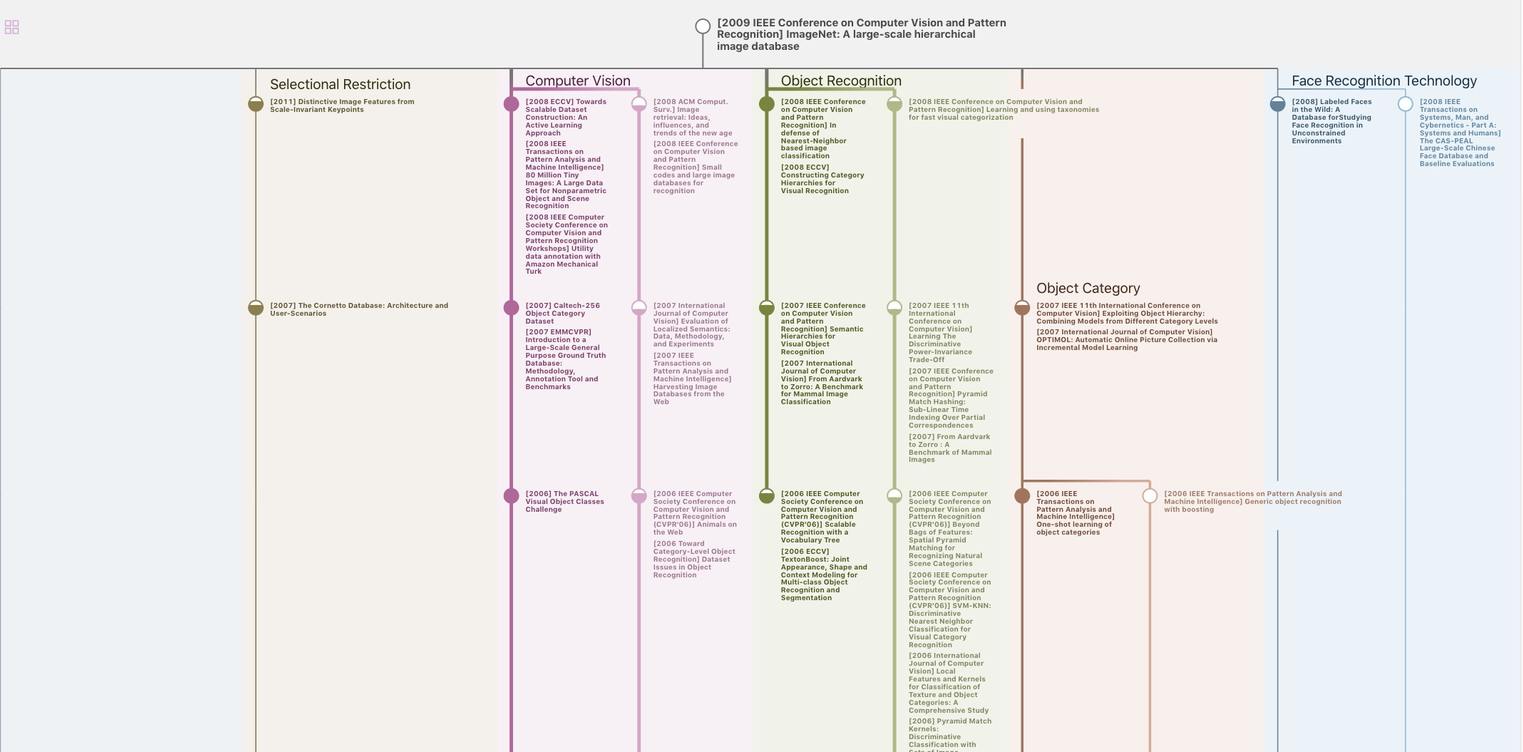
生成溯源树,研究论文发展脉络
Chat Paper
正在生成论文摘要