Analysis of Complete Blood Count Data and Use in Classification Machine Learning Models to Predict Icans Incidence Following CAR-T Cell Therapy
Transplantation and Cellular Therapy(2024)
摘要
Background
CD19 directed CAR-T cell therapies are increasingly used for treatment of hematological malignancies, however immune cell-associated neurotoxicity syndrome (ICANS), remains a challenge to predict and treat. We retrieved demographic and complete blood count (CBC) data from electronic health records (EHR) to evaluate whether this simple, accessible, and routinely collected data could be used to train classification machine learning (ML) models for prediction of ICANS.
Methods
Demographic and CBC measures from n=96 patients treated for B-cell malignancies at the University of Michigan (UM) were analyzed for univariate significance at baseline (prior to lymphodepletion) and through day (D) 3 post CAR-T infusion. Complete datasets were available for n=76 patients. These were then used for feature selection and ML model training. Features found significant were used as inputs in binary classification models to predict ICANS incidence within 20 days post-infusion. Models were validated using data from a test set of n=29 patients treated at Johns Hopkins Hospital (JHH). Data analysis was completed in RStudio-4.1.3, R Core Team 2022.
Results
Overall incidence of ICANS was 45% in the training set (UM) and 34% in the test set (JHH). While baseline data alone was not predictive, between D0 and D3, univariate analysis revealed lymphocyte count, monocyte count, red blood cell count, hemoglobin, hematocrit, C-reactive protein, ferritin, and white blood cell count as differentially associated with patients that developed Grade 3 ICANS versus those that developed Grade 1-2 or No ICANS. On D0 (after lymphodepletion and prior to CAR-T cell infusion), the combination of C-reactive protein, monocyte count, red blood cell count, and age used in ML models were able to predict incidence of any grade ICANS with an AU-ROC of 0.80-0.82, MCC=0.51-0.54, and F1=0.74-0.76. This model was robust to validation in the independent test set cohort (i.e., JHH patients), with an AU-ROC of 0.92-0.95, MCC=0.63-0.85, and F1=0.67-0.90.
Conclusions
ML-based classification using CBC data and commonly measured clinical blood analytes holds promise for ICANS prediction. Future work may integrate additional blood- clinical-, and/or digital-based biomarkers from other predictive models of ICANS to develop further improved models. Our findings suggest that ML-based models may be able to risk-stratify patients receiving CAR-T therapy, to identify those who may benefit from close monitoring and/or early therapeutic intervention to prevent or mitigate severity of ICANS.
更多查看译文
AI 理解论文
溯源树
样例
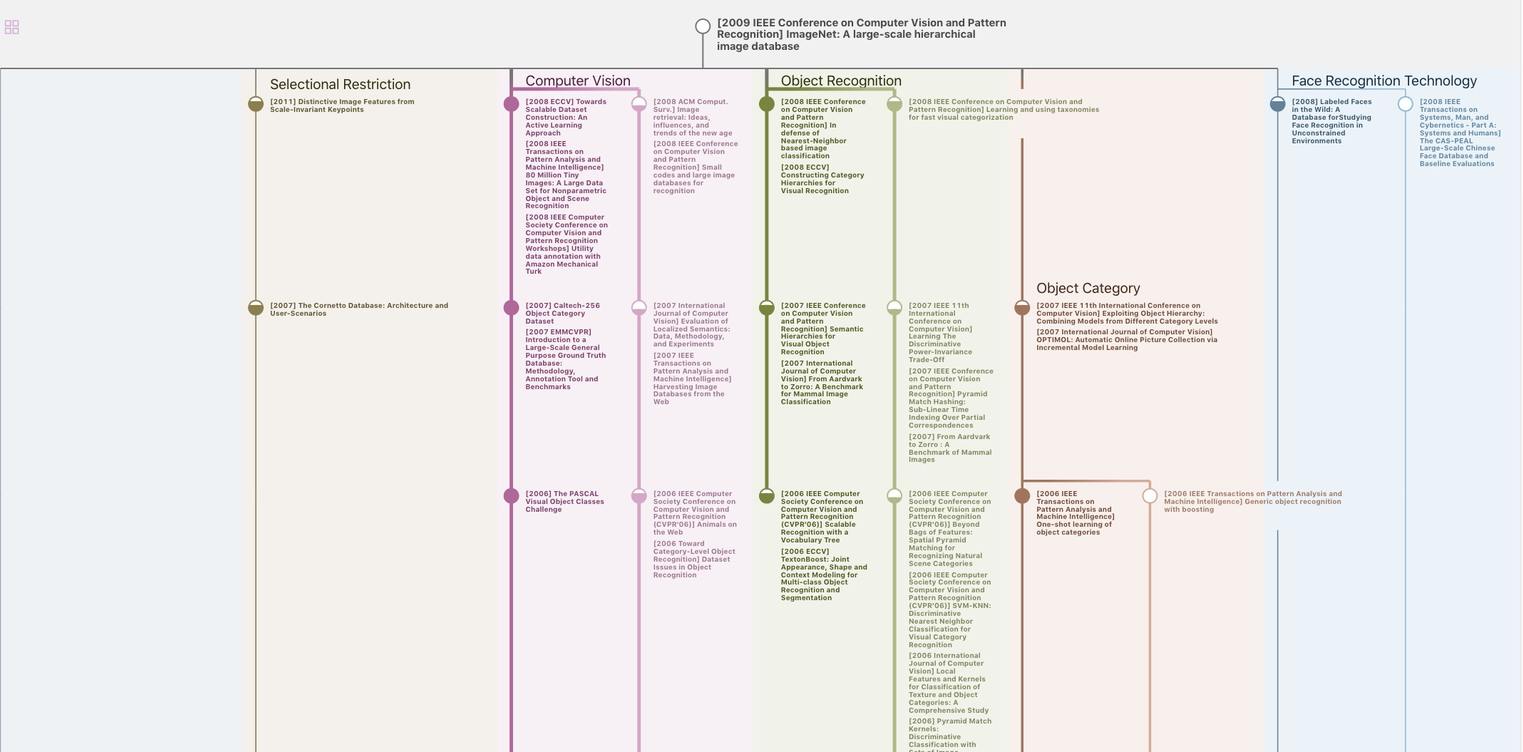
生成溯源树,研究论文发展脉络
Chat Paper
正在生成论文摘要