Green energy forecasting using multiheaded convolutional LSTM model for sustainable life
SUSTAINABLE ENERGY TECHNOLOGIES AND ASSESSMENTS(2024)
摘要
Using distributed energy resources can fulfil an individual's energy requirement, reducing electricity bills and creating sustainable energy solutions. Earlier, customers needed help utilising energy resources due to their limited knowledge. Technological advancement helps to utilise distributed energy sources using machine learning, deep learning, the Internet of Things, Wireless technologies, big data, etc. Although there are a lot of provisions for utilisation, the central issue is forecasting the generated renewable energy without wasting the generated power. Data is generated based on long periods of energy generated from wind and solar irradiance. Then, the generated data is trained using deep learning models. The trained models can predict the generated power through green energy resources by accurately forecasting the wind speed and solar irradiance. In this research, we propose an efficient approach for microgrid-level energy management in an intelligent community based on integrating energy resources and forecasting wind speed and solar irradiance using a deep learning model. An intellectual community with several smart homes and a microgrid is considered. This work proposes a multiheaded convolutional LSTM and particle swarm optimisation (PSO) technique (MHCLSTM-PSO). The results are obtained from data using wind speed and solar irradiance. The accuracy rate of CNN was 72.52%, LSTM was 78.16%, CLSTM was 85.56%, and our proposed work produced 93.54 %.
更多查看译文
关键词
Green energy forecasting,Sustainability,Multiheaded convolutional LSTM,Wind speed,Solar irradiance
AI 理解论文
溯源树
样例
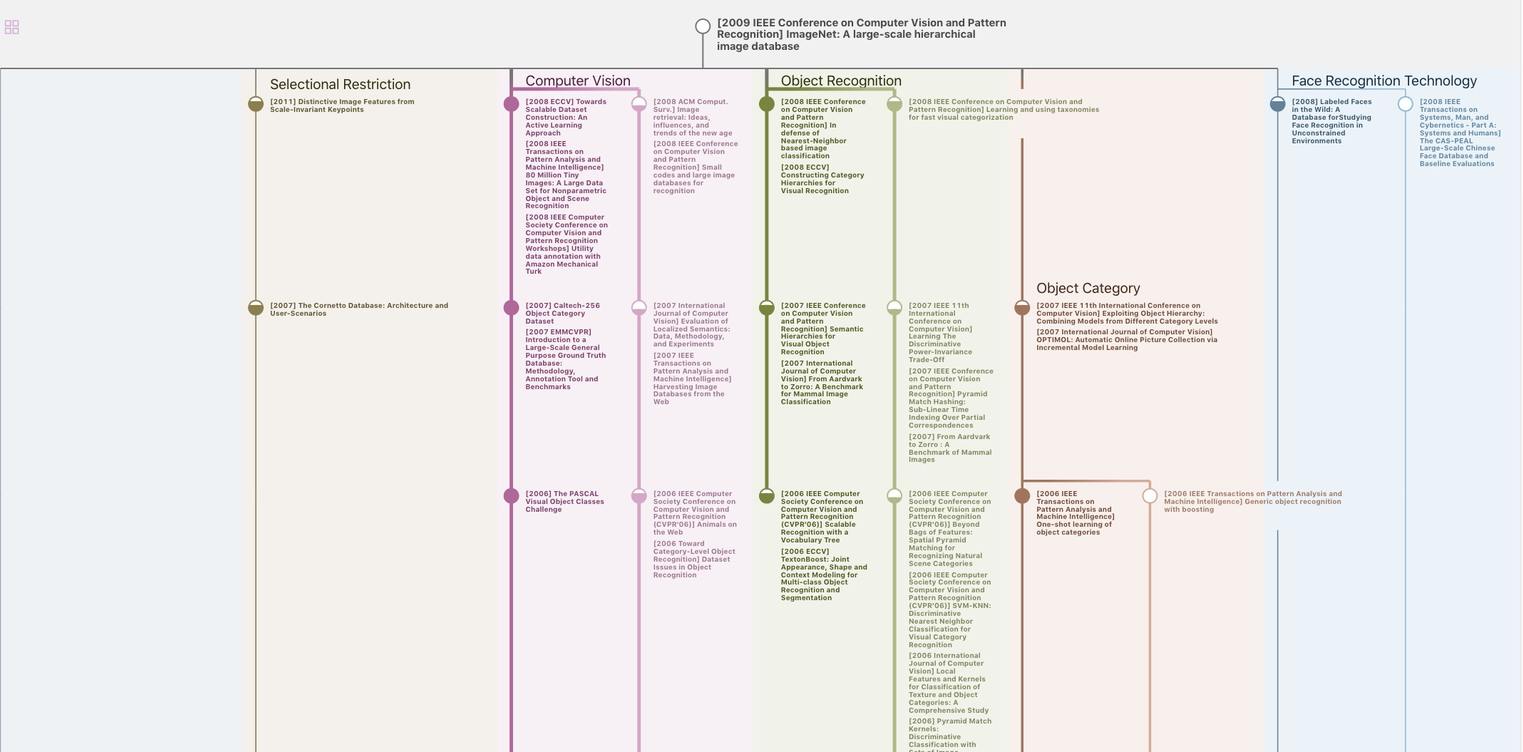
生成溯源树,研究论文发展脉络
Chat Paper
正在生成论文摘要