Comparing Binary Classification and Autoencoders for Vision-Based Anomaly Detection in Material Flow
Procedia CIRP(2024)
摘要
Increasing digitalization has revolutionized the manufacturing industry. Advances in sensor systems and artificial intelligence enable more efficient data collection and analysis. In this context, vision-based quality inspection for product quality assurance and cost reduction has become a critical part of manufacturing quality control processes. A relatively unexplored area in this context are Aanomalies in the material flow, which appear in various forms, from misplaced objects to positioning and rotation issues in all three dimensions. The complex nature of these features elaborates the use of advanced machine learning techniques. Conventional methods use binary classification to separate data into normal and anomalous classes. However, acquiring a sufficient amount of labeled anomaly data is still challenging. This work aims to investigate the effectiveness of binary classification and autoencoders for image-based anomaly detection in a frame-assembling material handling application. The two methods are evaluated in terms of their performance (ROC-AUC) in an anomaly detection task. Therefore, we designed a virtual test bench to create sufficient image data in a controlled setting. Here the rotation and position of the hook of the mounting device is varied to simulate normal and anomalous positionings. The study concludes that binary classification achieves higher accuracy than autoencoders. However, autoencoders require fewer data acquisition efforts and can detect subtle anomalies that binary classification may miss. In addition, autoencoders can improve over time with more data input but require more model engineering than image classification models. While the transferability of deep image classification models is shown in multiple applications, it remains an open question for autoencoders. The results suggest that the choice of which method to use depends on the specific requirements of the manufacturing process.
更多查看译文
关键词
Vision-based quality inspection,Anomaly detection,Binary classification,autoencoders,Material flow,Deep image classification models,Transfer Learning
AI 理解论文
溯源树
样例
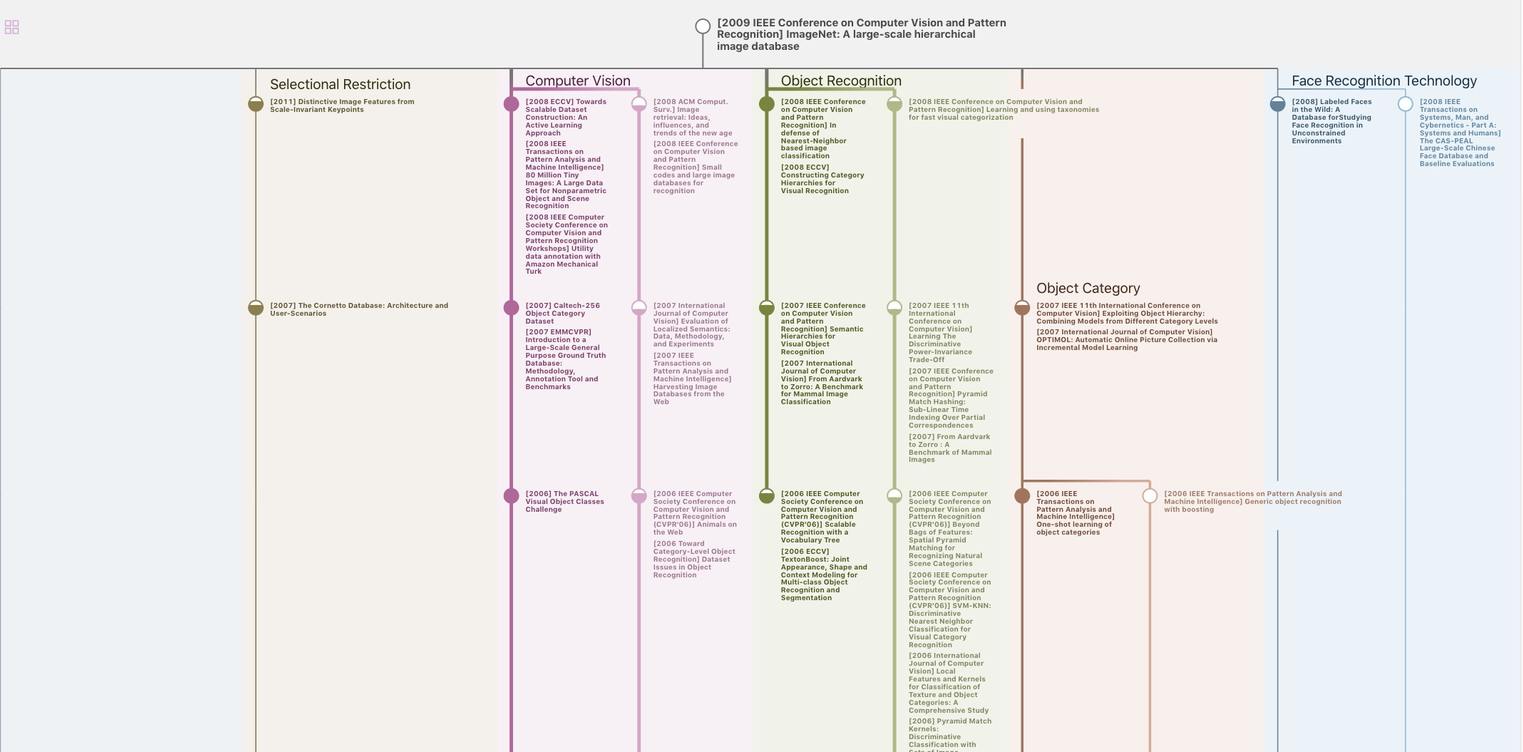
生成溯源树,研究论文发展脉络
Chat Paper
正在生成论文摘要