A kernel framework for learning differential equations and their solution operators
Physica D: Nonlinear Phenomena(2024)
摘要
This article presents a three-step kernel framework for regression of the functional form of differential equations (DEs) and learning their solution operators. Given a training set consisting of pairs of noisy DE solutions and source/boundary terms on a mesh: (i) kernel smoothing is utilized to denoise the data and approximate derivatives of the solution; (ii) This information is then used in a kernel regression model to learn the functional form of the DE; (iii) The learned DE is then used within a numerical solver to approximate the solution of the DE with a new source term or initial data, thereby constituting an operator learning framework. Numerical experiments compare the method to state-of-the-art algorithms. In DE learning our framework matches the performance of Sparse Identification of nonlinear Dynamical Systems (SINDy) while in operator learning the method has superior performance compared to well-established neural network methods in low training data regimes.
更多查看译文
关键词
Equation discovery,Operator learning,Reproducing kernel Hilbert spaces,Physics informed machine learning
AI 理解论文
溯源树
样例
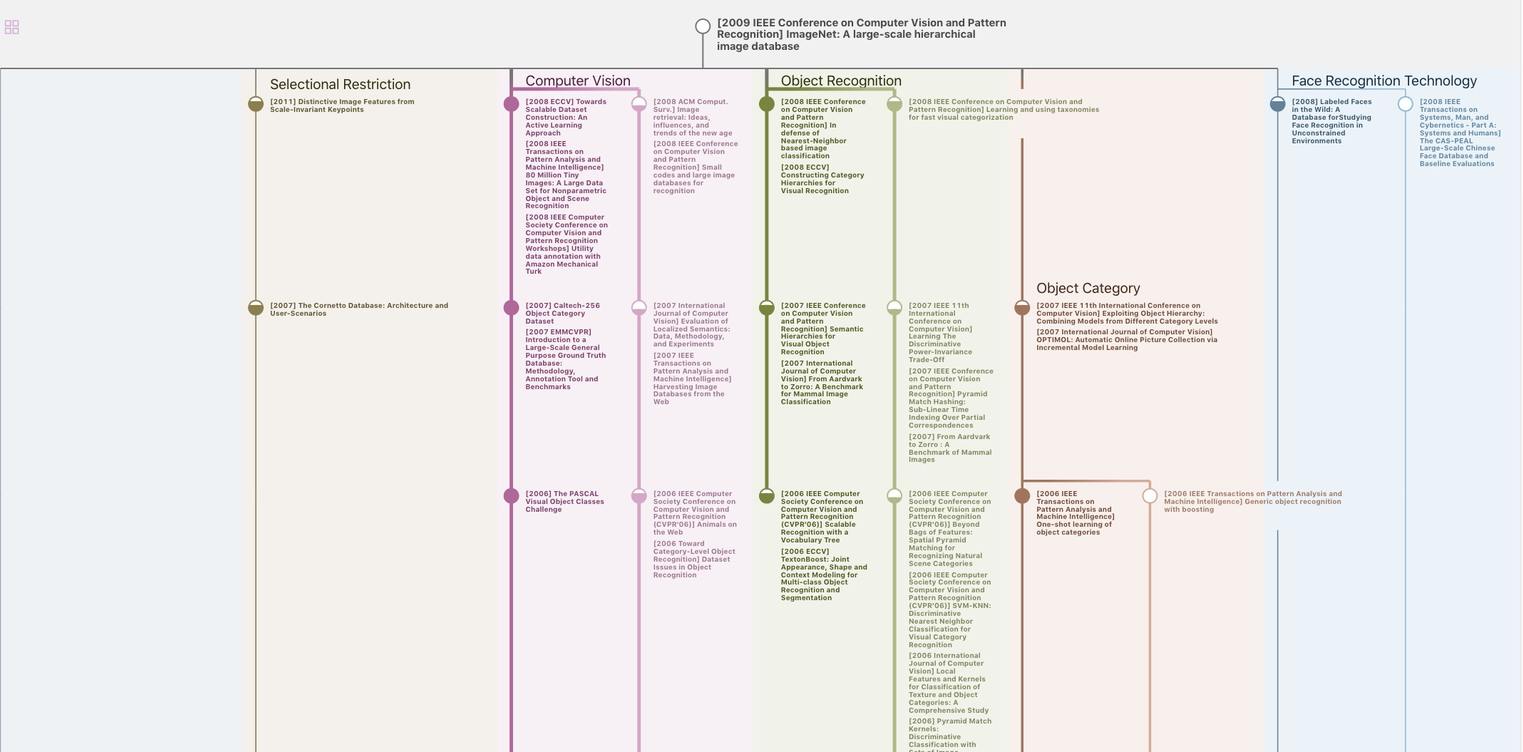
生成溯源树,研究论文发展脉络
Chat Paper
正在生成论文摘要