Dual-stream deep learning integrated multimodal sensors for complex stimulus detection in intelligent sensory systems
NANO ENERGY(2024)
摘要
Multimodal tactile sensors that can detect multiple external stimuli in a single device hold great promise within the domains of wearable technology and robotics. However, accurate decoupling of complex intermixed stimuli remains a significant challenge for real-time detective sensory system, hampering their versatile utilization. Here, we present a multimodal sensor platform that integrates a dual-stream deep learning process and microporous ionotronic multimodal tactile sensors. Importantly, the synergetic combination of carbon black and poly (vinylidene fluoride-co-hexafluoropropylene)/ion-gel (CBIG) facilitated a dual-mode sensing capability for both pressure and temperature (in capacitive and resistive modes), with high sensitivity of 0.350 kPa- 1 and - 0.745% celcius- 1, respectively. Micro-computed tomography revealed that the large capacitive change with pressure is attributed to the decrease of micro-pore volume and enlarged contact area between the electrode and the CBIG foam where an electric-double-layer is formed. By adopting a deep learning process based on a regression model, highly accurate identification of arbitrary intermixed pressure and temperature stimuli was possible, showing mean-absolute-percentage-error values of 1.58% and 2.37%, respectively. By utilizing the CBIG sensor integrated with the deep learning framework, simultaneous detection of surface temperature and pressure is demonstrated using a robotic arm, showcasing the versatile utilization of CBIG sensors in energy-efficient intelligent sensory systems.
更多查看译文
关键词
Multimodal sensors,Carbon black,Ion -gel,Dual -stream deep learning,Regression model
AI 理解论文
溯源树
样例
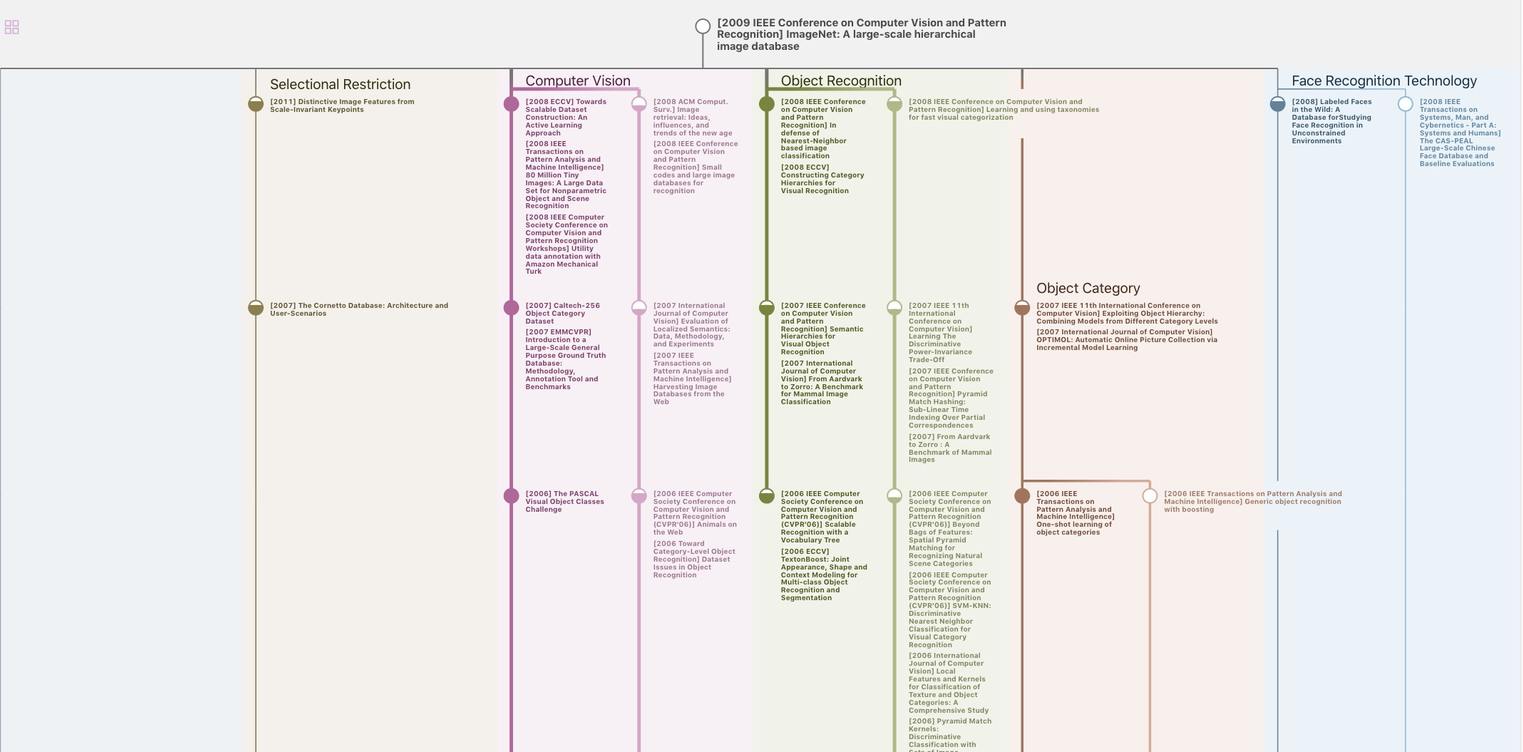
生成溯源树,研究论文发展脉络
Chat Paper
正在生成论文摘要