Contrastive learning for hierarchical topic modeling
Natural Language Processing Journal(2024)
摘要
Topic models have been widely used in automatic topic discovery from text corpora, for which, the external linguistic knowledge contained in Pre-trained Word Embeddings (PWEs) is valuable. However, the existing Neural Topic Models (NTMs), particularly Variational Auto-Encoder (VAE)-based NTMs, suffer from incorporating such external linguistic knowledge, and lacking of both accurate and efficient inference methods for approximating the intractable posterior. Furthermore, most existing topic models learn topics with a flat structure or organize them into a tree with only one root node. To tackle these limitations, we propose a new framework called as Contrastive Learning for Hierarchical Topic Modeling (CLHTM), which can efficiently mine hierarchical topics based on inputs of PWEs and Bag-of-Words (BoW). Experiments show that our model can automatically mine hierarchical topic structures, and have a better performance than the baseline models in terms of topic hierarchical rationality and flexibility.
更多查看译文
关键词
Hierarchical topic modeling,Contrastive learning,Neural variational inference
AI 理解论文
溯源树
样例
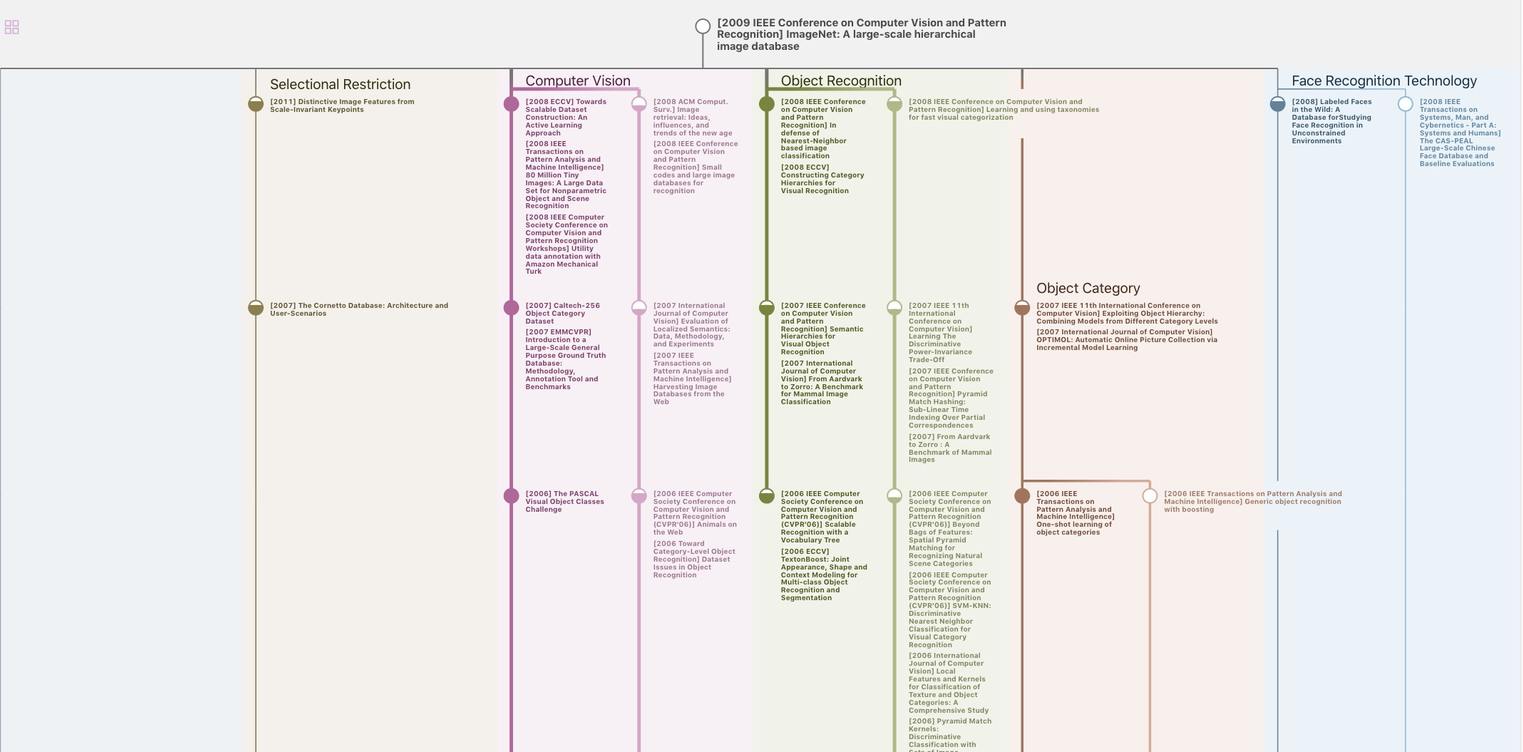
生成溯源树,研究论文发展脉络
Chat Paper
正在生成论文摘要