Machine learning-based discovery of molecular descriptors that control polymer gas permeation
JOURNAL OF MEMBRANE SCIENCE(2024)
摘要
While machine learning has found increasing use in predicting the properties of polymeric materials with only a knowledge of chain architecture, determining the molecular factors underpinning properties ("interpretable AI") has remained less well explored. We show that encoding chain chemistry in commonly employed formats, e.g., binary -valued fingerprints, leads to uniqueness issues during the hashing process to save storage space. This is because the hashing algorithm can map several chemical moieties into the same bit. These issues carry over into the ML algorithms, especially for "inverse"design and interpretable AI, and cannot be avoided by changing the length of the fingerprint. Using MACCS key featurizations of monomer repeats resolves some of these issues, and we show that a few substructures consistently appear in top features for maximizing permeability across several gases and ML models. These are carbon-carbon double bonds (as in polyacetylenes) especially when they are associated with methyl groups (found in branching architectures). These results, derived from the limited data set of - 500 polymers with experimental gas permeation data, are in agreement with physical insight and thus provide a robust foundation which could further enable study of these material classes through detailed experiments and simulations.
更多查看译文
关键词
Machine learning,Membranes,Polymers,Gas transport,Explainable AI
AI 理解论文
溯源树
样例
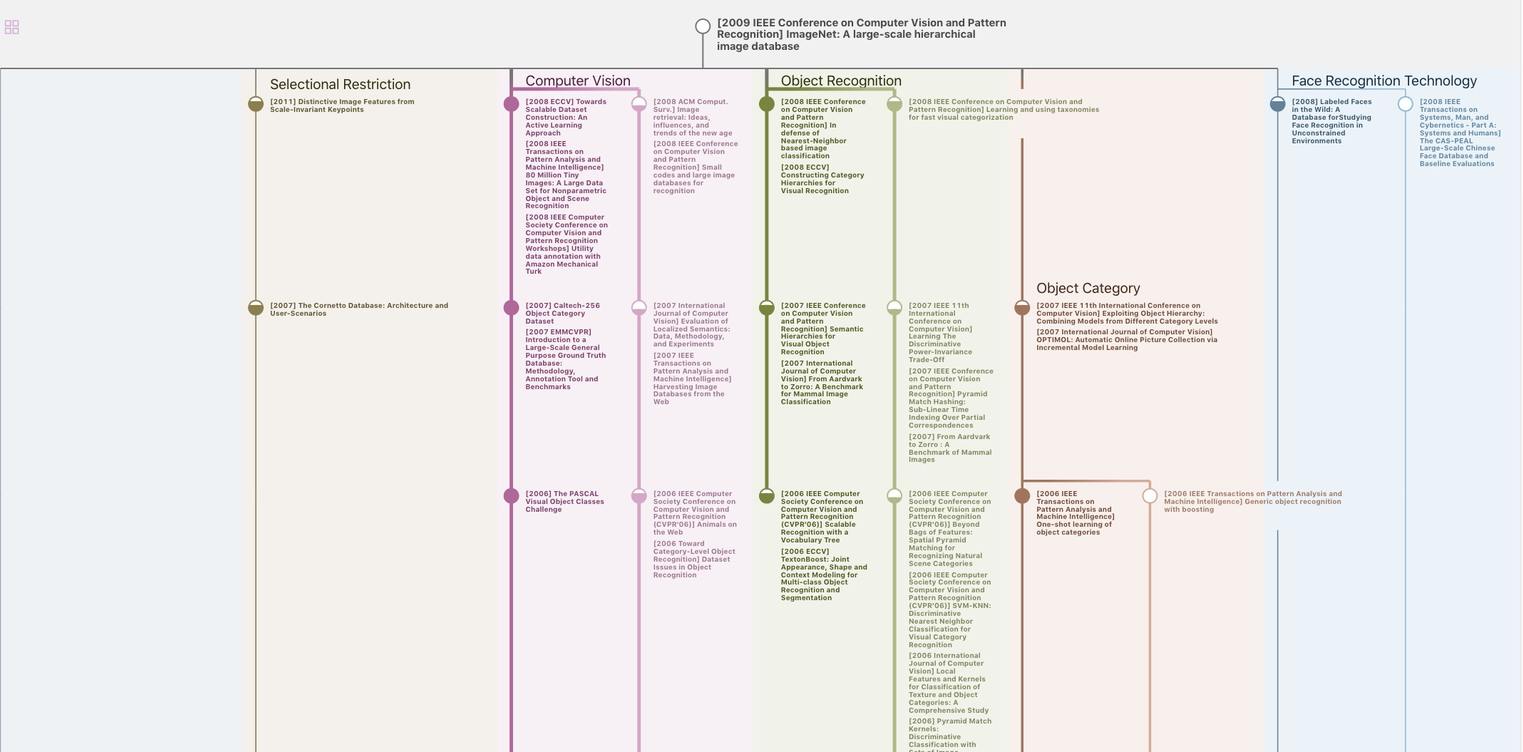
生成溯源树,研究论文发展脉络
Chat Paper
正在生成论文摘要