A ground-independent method for obtaining complete time series of in situ evapotranspiration observations
JOURNAL OF HYDROLOGY(2024)
摘要
In situ evapotranspiration (ET) observations play an important role in validating remotely-sensed ET models and products and analyzing eco-hydrological processes. The eddy covariance technique has been widely utilized to obtain ground truths. However, due to power failures, sensor malfunction, and unreasonable observation, ET measured by the eddy covariance is temporally discontinuous at the hourly and daily scales, which limits the application of the observed ET data. We cannot fill the gaps using ground-based auxiliary measurements because of their discontinuity due to similar reason. To solve this "double-discontinuous trouble" issue and obtain a complete time series of in situ ET, we proposed a ground-independent (GI) method based on multi-source data and machine learning (ML) algorithm. First, reference variables were selected from reanalysis and remote sensing data according to the Penman-Monteith (PM) equation and processed to form the temporally continuous data. Then, the ML algorithm was trained to establish the relationship between ET and reference variables. Finally, the gaps were filled by the well-trained ML model to obtain temporally continuous ET. This study took the random forest (RF) algorithm as an example, and the method was tested at 6 sites. Results show that the proposed GI method has excellent overall performance (averaged R2 = 0.85, RMSE = 39.88 W/m2) and showing better performance than previous studies at cropland and forested sites, with the averaged R2 of 0.85 and 0.82, respectively. Using reanalysis data as reference data causes 3.5 % decrease for R2 compared with the grounddependent (GD) method, but the GI method is more widely applicable. Furthermore, the GI method can effectively fill gaps under different gap length scenarios, even for very long gaps scenario (R2 = 0.75). We also found that the RF outperforms the other three ML algorithms, and it is essential to include at least radiation, normalized difference vegetation index, and air temperature in the reference data. In conclusion, we provided an accurate and efficient gap-filling method independent of ground-based auxiliary observations. This proposed GI method could be valuable in supporting hydro-ecological research and the validation of ET models and products.
更多查看译文
关键词
Evapotranspiration,Gap -filling,Machine learning,In situ observations,Eddy covariance,Multi -source data
AI 理解论文
溯源树
样例
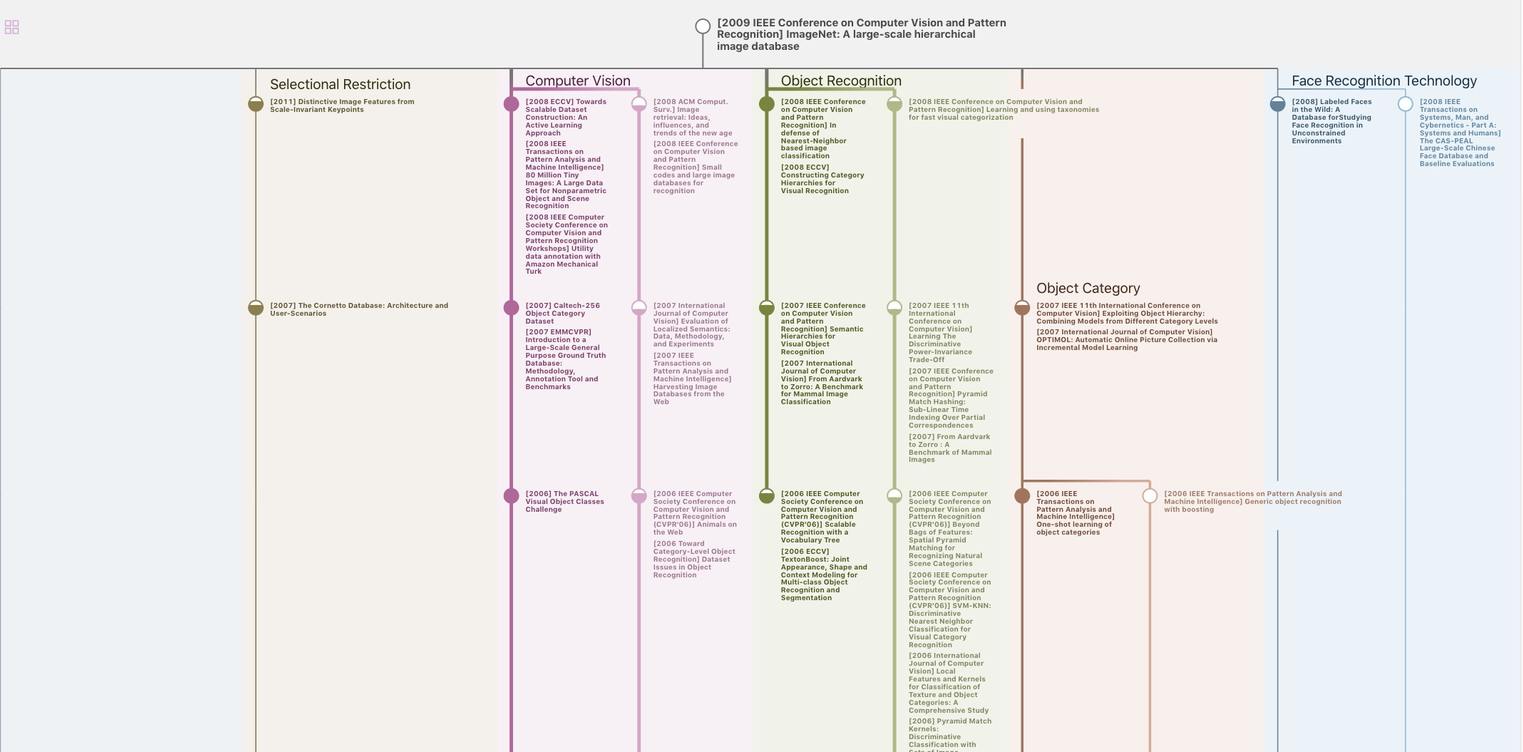
生成溯源树,研究论文发展脉络
Chat Paper
正在生成论文摘要