Multi-distribution mixture generative adversarial networks for fitting diverse data sets
EXPERT SYSTEMS WITH APPLICATIONS(2024)
摘要
There has been remarkable success in many areas with generative adversarial networks (GAN). However, their performance is usually limited when they are trained on data sets with different distributions. To address this challenge, we propose a novel model, termed the multi-distribution mixture generative adversarial networks (MDM-GAN), which takes the mixed distribution concatenated through latent dimension as the probability distribution of the latent adversarial space. When dealing with data sets from unknown distribution, we put mixed noise vectors sampled from the latent space of the mixture multi-distribution into model. To enhance the stability of the generation process, we utilize segmented generative networks to divide the generation process into two parts. Specifically, the first part generates fake dependent data in a uniform space, while the second part generates fake samples in the sample domain. Furthermore, we introduce a statistical constraint term into the generator and discriminator losses of the first network in order to improve its performance. We conducted extensive experiments, based on important quantitative metrics to evaluate the generated samples in terms of quality and diversity. The analysis on the results show that our proposed model excels in generating high-quality and diverse samples across different tasks, demonstrating strong adaptability and superior performance.
更多查看译文
关键词
Generative adversarial networks (GAN),Copulas,Multi-distribution,Dependence,Generality,Segmentation
AI 理解论文
溯源树
样例
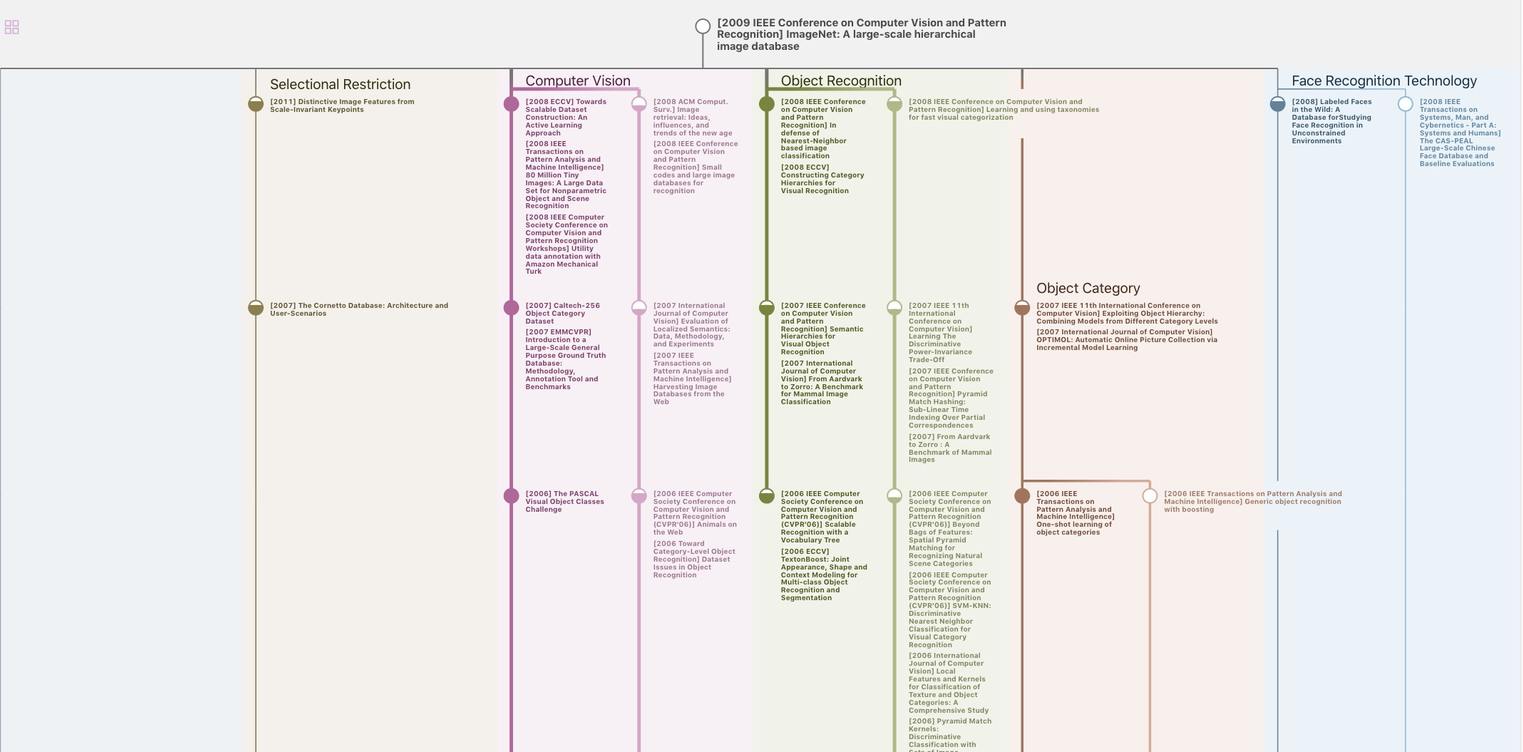
生成溯源树,研究论文发展脉络
Chat Paper
正在生成论文摘要