Lightweight image super-resolution network based on extended convolution mixer
Engineering Applications of Artificial Intelligence(2024)
摘要
The single image super-resolution (SISR) is a computer vision task needed in many real-world applications. There are many methods developed to solve ill-posed SISR problem; however, these methods are based on attention mechanisms that need a large computing processing cost. So, these attention-based models cannot be used in real-world applications that need fast models. Thus, we propose an enhanced convolution mixer (EConvMixer) module to solve this SISR problem by using lower computing convolution layers. The EConvMixer is designed based on utilizing three convolution types, namely the dilated depthwise convolution for increasing the receptive field, the depthwise convolution for mixing spatial locations, and the pointwise convolution for mixing channel locations. Based on using this EConvMixer layer, we build a lightweight extended convolution mixer network (EConvMixN) for SR images. The EConvMixN has the spirit of the transformer model but with a low computational complexity using only convolution layers. It is clear that our model achieves appealing visual quality and reconstruction accuracy. Also, the EConvMixN model is faster than the state-of-the-art results at different SR scales. Moreover, the EConvMixN achieves state-of-the-art runtime in multiple SR scales. Finally, our model improves PSNR compared to CoMoNet-S by 0.12 dB and 0.08 dB for datasets of Set5 and Set14 at the scale of × 3.
更多查看译文
关键词
Image super-resolution,Extended convolution mixer layer,Lightweight super-resolution model,Depthwise convolution,Pointwise convolution
AI 理解论文
溯源树
样例
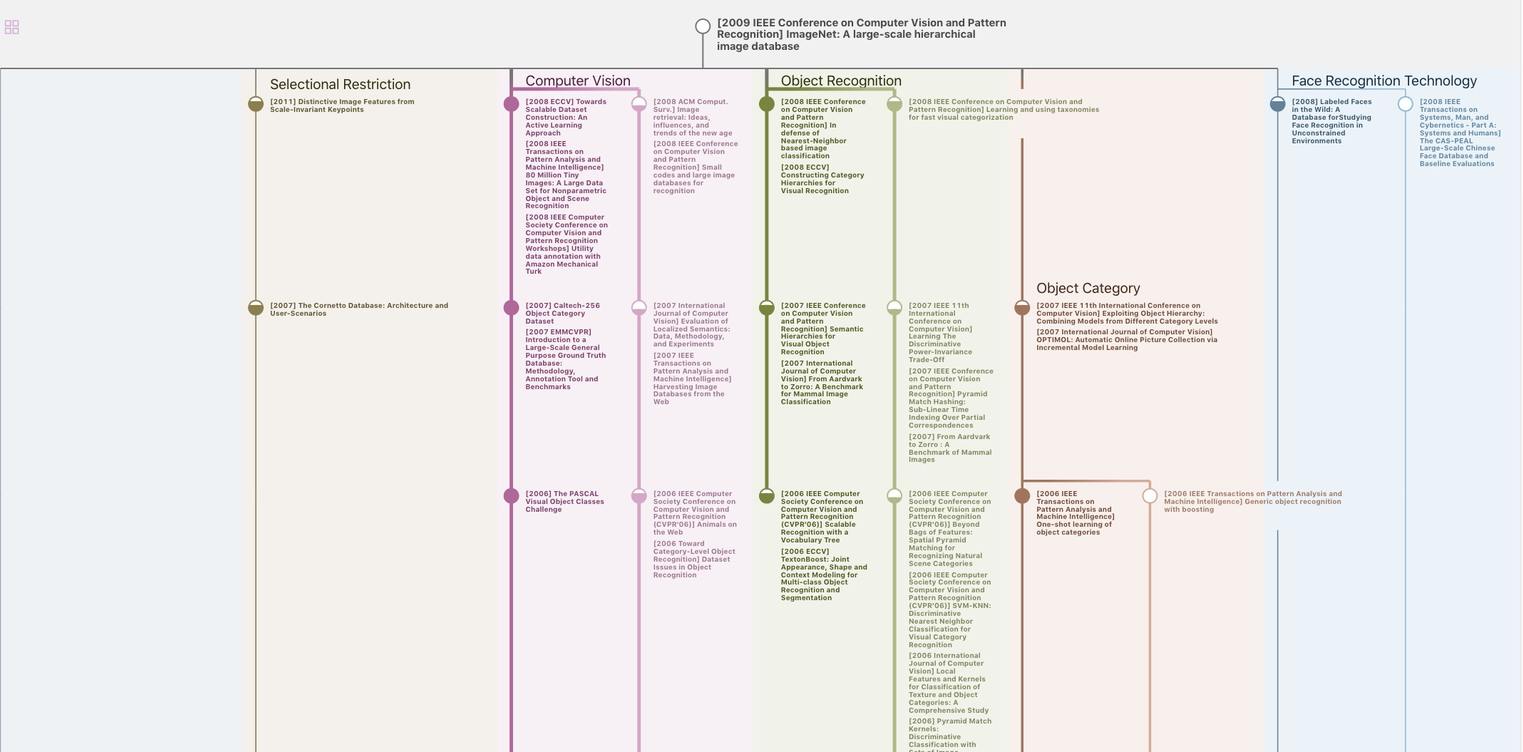
生成溯源树,研究论文发展脉络
Chat Paper
正在生成论文摘要